Factor analysis: decipher complex data and make better decisions
Appinio Research · 05.12.2023 · 16min read
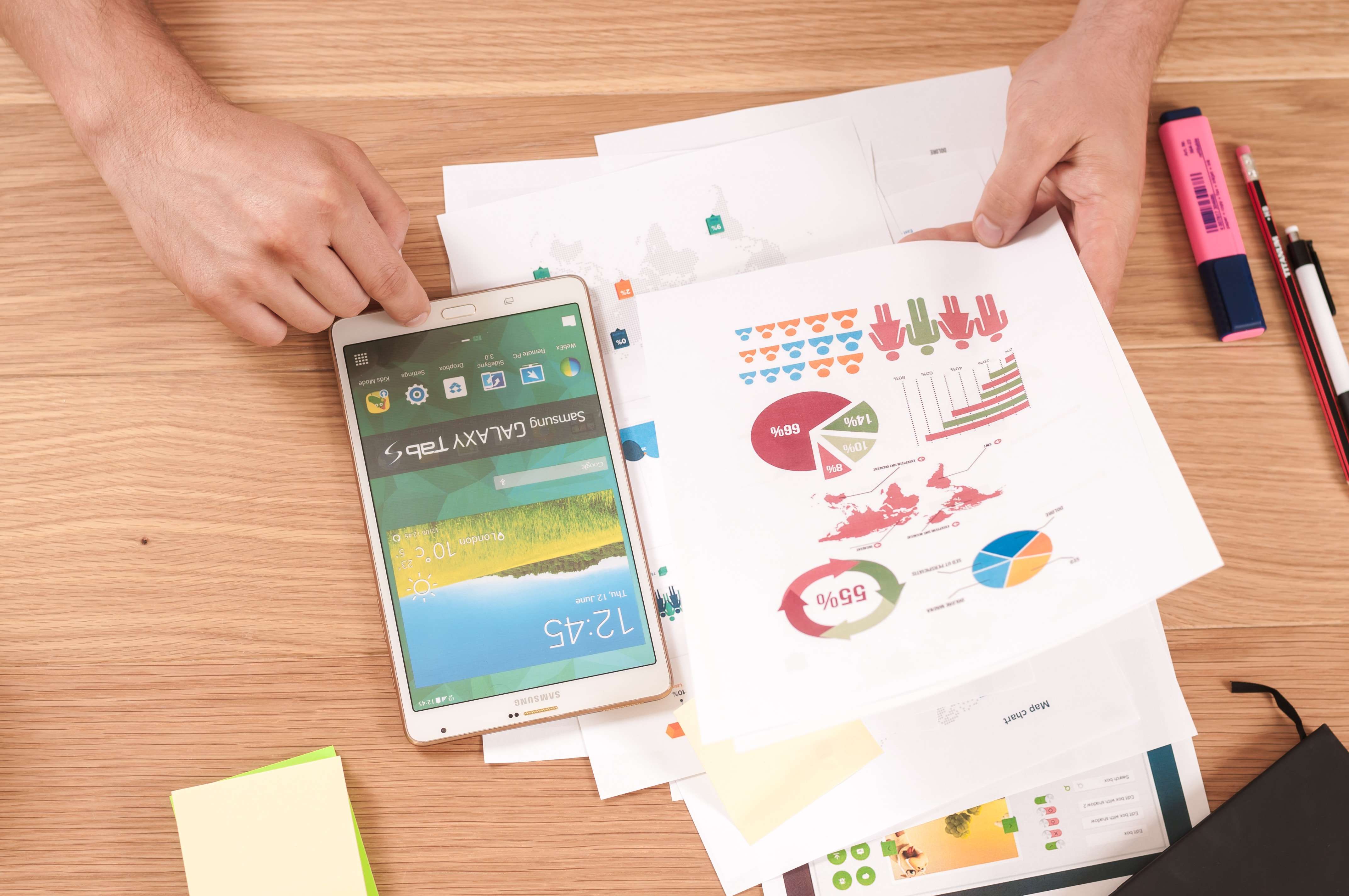
Content
In today's fast-paced marketing world, making decisions based on data gives companies a real edge. But let's face it, collecting data is one thing, figuring out what it all means is another ball game. That's where factor analysis swoops in like a superhero for your complex data sets.
Imagine it like this: it's like a magic trick to make big, messy data sets simpler and spot the cool patterns. In this guide, we're going to chat about where factor analysis comes from, how it does its thing, the good stuff it brings to the table, and even its not-so-great sides. Plus, we'll spill the beans on the difference between exploratory and confirmatory factor analysis and a bunch more exciting tips.
What is factor analysis?
Factor analysis, a statistical method tailored for unraveling complex data sets, serves as a crucial analytical tool in modern decision-making processes.
At its core, factor analysis strives to streamline extensive datasets by distilling them into a few essential factors. The ultimate goal is to enhance clarity and manageability in handling substantial amounts of data.
Additionally, factor analysis plays a pivotal role in unearthing concealed patterns and structures within datasets, shedding light on intricate phenomena and relationships.
To appreciate the significance of this methodology, let's delve into a concise historical overview
A Historical Perspective
Originating in the late 19th century within the realm of psychology, factor analysis found its early roots during the endeavors of Francis Galton and Charles Spearman. Their pursuit revolved around developing tests to gauge intelligence.
Spearman, in 1904, introduced the first mathematical approach to factor analysis, laying the groundwork for its subsequent applications across diverse disciplines such as statistics, social sciences, economics, and market research.
Advancements in technology, coupled with the advent of sophisticated data analysis software, have propelled factor analysis into a more accessible and precise realm.
Far from a relic of the past, this methodology stands as a contemporary powerhouse for pattern recognition and information extraction from intricate data sets.
What is factor analysis used for?
Whether deployed in a confirmatory or exploratory manner, factor analysis proves instrumental in simplifying market segmentation, devising targeted marketing strategies, and optimizing products and services.
Its ability to facilitate data-driven decision-making processes positions factor analysis as a catalyst for organizations seeking a competitive advantage.
By distilling complex data sets, factor analysis provides valuable insights into consumer behavior and preferences.
Market researchers leverage this methodology to identify patterns and structures in surveys and customer evaluations, offering a deeper understanding of purchasing behavior.
Ultimately, the reduction of factors and variables to their core elements enhances comprehension of the needs and preferences of target groups.
When is a factor analysis useful?
Factor analysis proves its worth in various scenarios, offering a multifaceted approach to data exploration. Let's break down its utility:
- Streamlining variables
In instances where datasets boast an extensive array of variables, factor analysis steps in to categorize and simplify the data. By doing so, it transforms a potentially overwhelming set into a clearer and more interpretable format. - Unveiling patterns
Factor analysis, particularly in its explorative form, plays the role of a detective, unveiling concealed patterns and structures within the data. This process brings hidden connections to light, providing a richer understanding of the underlying dynamics. -
Variable classification
Ever wondered which factors carry the most weight in a dataset? Factor analysis shines a light on the variance within datasets, elucidating the influence each factor wields. This classification proves invaluable in discerning the pivotal elements within complex data structures. - Testing theories and hypotheses
For situations where concrete assumptions or hypotheses already exist regarding data content and structure, confirmatory factor analysis steps in. It systematically checks these assumptions, serving as a validation tool that either affirms or challenges existing theories. - Segmentation mastery
Factor analysis serves as a keen observer in the realm of customer profiles. By scrutinizing similarities and differences among these profiles, it becomes a linchpin in the definition of distinct customer segments. This segmentation approach provides nuanced insights into diverse consumer behaviors.
In essence, factor analysis transcends the mere simplification of data; it acts as a versatile analytical ally, unveiling the intricacies of datasets and offering a structured lens through which to understand, validate, and strategically utilize information.
Where does factor analysis reach its limits?
While factor analysis stands as a formidable tool, it's imperative to acknowledge its limits and exercise discretion based on specific objectives and data quality. This method, powerful as it may be, isn't a one-size-fits-all solution. Let's explore the situations where factor analysis might take a backseat:
- Not a panacea
Factor analysis excels at reducing variables and exploring relationships within a dataset. However, when the goal is to pinpoint and form groups of similar data for a more specific segmentation, cluster analysis takes the lead. It's tailored for the task of identifying distinct groups and segments within a dataset, surpassing factor analysis in this particular arena.
- The power of regression
When the emphasis shifts to understanding the relationship between variables, regression analysis often emerges as the more fitting choice. Unlike factor analysis, which hones in on complex patterns and hidden structures, regression analysis specifically delves into the influence of one or more independent variables on a dependent variable.
- Navigating customer preferences
Factor analysis encounters its limitations when the focus zeroes in on discerning customer preferences or specific values tied to various product characteristics and features. In such cases, where a detailed understanding of customer preferences is key, conjoint analysis is a more tailored alternative.
In essence, while factor analysis stands as a robust methodology, its effectiveness is context-dependent.
Recognizing the unique strengths of other analytical tools, such as cluster analysis and regression analysis, ensures a comprehensive approach to data exploration and interpretation. The selection of the most suitable method hinges on a nuanced understanding of the specific objectives and the intricacies of the dataset at hand.
Where is factor analysis used?
Despite its roots in psychology, factor analysis has evolved into a versatile tool, finding applications across a spectrum of disciplines.
- Market research insights
In the realm of market research, factor analysis takes center stage. Its primary role involves segmenting target and buyer groups, offering a nuanced understanding of product preferences. By deciphering these preferences, businesses can craft targeted marketing strategies that resonate with specific consumer segments. - Economic and financial analysis
Factor analysis extends its reach into the economic and financial sectors. Here, it delves into variables such as interest rates, inflation, and share prices. The objective is clear: to analyze and determine the risk-return profiles of investment portfolios. By identifying and understanding these key factors, financial analysts can make informed decisions, optimizing investment strategies. - Healthcare insights
The medical and healthcare domains leverage factor analysis for a different purpose. Here, it plays a crucial role in identifying associated symptoms or risk factors for diseases, particularly in fields like cardiovascular health. By discerning these factors, medical professionals can enhance diagnostic accuracy and refine treatment approaches. - Tech-driven advancements
Beyond traditional sectors, factor analysis steps into the realms of image and speech processing. In image processing, it excels at extracting features from large datasets, contributing to advancements in facial recognition technology. Similarly, in speech processing, factor analysis aids in enhancing the extraction of key features, refining the capabilities of speech recognition systems.
Exploratory vs. confirmatory factor analysis
Factor analysis adapts to the specific needs of research objectives and data scenarios, presenting two distinct methodologies: exploratory and confirmatory factor analysis.
- Exploratory Factor Analysis (EFA)
Positioned at the inception of research endeavors, exploratory factor analysis shines when the terrain is uncharted or information is incomplete. It becomes the investigator's tool, delving into large datasets to unearth latent patterns that were previously unknown. In this phase, exploratory factor analysis not only simplifies data but also acts as a catalyst for generating new hypotheses, providing a solid foundation for subsequent stages of research. - Confirmatory Factor Analysis (CFA)
As research progresses into more advanced stages, confirmatory factor analysis takes the spotlight. This method is tailor-made for situations where existing hypotheses need validation. To employ confirmatory factor analysis effectively, a well-defined model is crucial, built upon established theoretical frameworks or previous studies. It operates as a precision tool, rigorously testing specific relationships between variables, aligning research outcomes with pre-existing theories.
In essence, the choice between exploratory and confirmatory factor analysis hinges on the stage of research and the clarity of existing hypotheses.
Exploratory factor analysis pioneers the exploration of unknown territories, unraveling patterns and generating new insights.
On the flip side, confirmatory factor analysis stands as the sentinel of validation, ensuring that research findings align with established theoretical constructs.
Together, these dual approaches empower researchers to navigate the intricate landscape of data analysis with precision and purpose.
What are the advantages and disadvantages of factor analysis?
Factor analysis, like any analytical tool, comes with a set of advantages and drawbacks, shaping its utility in various contexts.
Advantages
- Data simplification and focus
Factor analysis excels in simplifying intricate datasets, distilling them to their essential components. By highlighting the most crucial points, it transforms data complexity into a more manageable and insightful form.
- Unearthing hidden patterns
The method serves as a detective, uncovering latent patterns that might otherwise slip through the cracks. This capability brings valuable insights, contributing to a more comprehensive understanding of the data.
- Clarity amidst complexity
In the midst of the data jungle, factor analysis brings clarity. It acts as a guide, simplifying interpretation and making the data more accessible, aiding in effective decision-making.
- Testing ideas and theories
Factor analysis provides a robust platform for hypothesis testing. By comparing data and checking for plausibility, it becomes a reliable tool for scrutinizing ideas and theories against empirical evidence.
Disadvantages
- Dependency on data quality
The reliability of factor analysis is contingent upon the quality and comprehensiveness of the input data. In cases where data is lacking in these aspects, the results may be compromised. - Assumption sensitivity
The outcomes of factor analysis are sensitive to the clarity of data summaries and the assumptions underlying them. Ambiguities in these aspects can introduce uncertainties into the results. - Incompatibility with poor data
Factor analysis is not the go-to approach when dealing with incomplete or poor-quality data. Its effectiveness is contingent upon a robust dataset, and deviations from this standard may undermine its validity. - Human judgment in factor selection
The method relies on human judgment for factor selection, introducing an element of subjectivity. This reliance on interpretation can lead to errors, impacting the accuracy of the analysis. - Risk of over-extraction
Factor analysis, in its quest for patterns, runs the risk of extracting excessive detail from the data. This unintended consequence may increase complexity, potentially hindering rather than aiding understanding.
7 steps to optimal factor analysis
Wie funktioniert eine Faktorenanalyse in der Marktforschung? Dazu braucht es lediglich sieben Schritte – ohne dabei zu sehr ins Detail zu gehen:
The integration of factor analysis into market research involves a systematic process, encompassing seven key steps that pave the way for valuable insights:
-
Data preparation
Begin by collecting comprehensive datasets, ensuring that the data is normalized for effective comparison. The choice between exploratory and confirmatory factor analysis is a critical decision at this stage and sets the tone for the subsequent analytical journey. -
Determining factors
The next step involves determining the number of factors to be extracted. This decision is driven by research objectives or can be guided by statistical methods, aligning the analysis with the specific goals of the study. -
Implementation
Employing statistical software, such as SPSS, becomes essential in this phase. These tools perform intricate mathematical calculations to extract factors, unveiling the underlying patterns within the datasets. -
Factor rotation
Factor rotation, while not altering the data's content, enhances interpretability. This step simplifies the factors' interpretation, making the results more accessible without modifying the intrinsic meaning of the data. -
Interpretation of factors and factor loadings
With factors in hand, attention turns to interpreting factor loadings. This analysis unveils the relationships between factors and original variables, paving the way to name and interpret these factors. It's the bridge to understanding the intricate interplay within the data. -
Interpretation of results
Delving into the interpreted factors provides insights into the underlying structures and correlations of data and variables. This critical phase aids in forming a cohesive narrative around the relationships discovered during factor analysis. -
Use of results
The derived factors, beyond merely simplifying datasets, become powerful tools. They serve as a foundation for developing hypotheses or, in the context of market research, segmenting target groups. The insights gained from factor analysis become integral to shaping strategic decisions and refining marketing approaches.
Aggregation of several observed statistical variables into a few latent variables (factors)
Example of a factor analysis: Reasons for buying a car
Let's shift from theory to the real-world application of factor analysis, exemplified in the automotive industry where understanding the factors influencing car buyers becomes paramount.
In this scenario, a company embarks on a data collection journey, gathering insights from hundreds of customers regarding various vehicle features. These features encompass a spectrum, ranging from engine performance and fuel efficiency to price, design, and safety features.
- Data collection
The first step involves collecting a diverse dataset that encapsulates the multifaceted aspects of a car. Engine performance, fuel efficiency, price, design, and safety features are meticulously cataloged, forming the foundation for factor analysis. - Factor identification
Employing factor analysis, the company identifies the underlying factors exerting the most significant influence on the purchase decision. Through this analytical lens, factors like "environment" and "safety" emerge as the driving forces shaping customers' choices. - Strategic decision-making
Armed with these insights, the company gains a nuanced understanding of customer preferences. This clarity enables the development of targeted marketing strategies, tailoring messages to highlight the environmentally conscious and safety-oriented aspects of their vehicles. - Product development
Beyond marketing, the findings guide decisions in product development. Understanding that environmental concerns and safety features top the priority list, the company can focus on integrating and enhancing these elements in their vehicles, aligning their offerings with the genuine needs and desires of their customer base.
Conclusion: Understanding target groups better with factor analysis
In the contemporary landscape of data-driven decision-making, factor analysis stands out as an invaluable asset for companies and market researchers alike.
Its ability in distilling clarity from complex data sets, unearthing concealed patterns, and facilitating informed decision-making positions it as an indispensable tool. By simplifying data and enhancing its structural organization, factor analysis empowers companies to decipher customer preferences, fine-tune marketing strategies, and align products with market needs.
The insights garnered extend beyond mere statistical analysis, delving into the intricate realm of consumer behaviors and preferences.
Factor analysis emerges not just as a methodology but as a powerful ally in navigating the competitive terrain, enabling businesses to not only survive but thrive by better serving the evolving needs of their customers.
Interested in running your own study?
In our dashboard, you will find questionnaire templates that you can customize and get the insights you need to bring your brand to the next level.
Get facts and figures 🧠
Want to see more data insights? Our free reports are just the right thing for you!