What is Data Collection? Methods, Types, Tools, Examples
Appinio Research · 09.11.2023 · 33min read
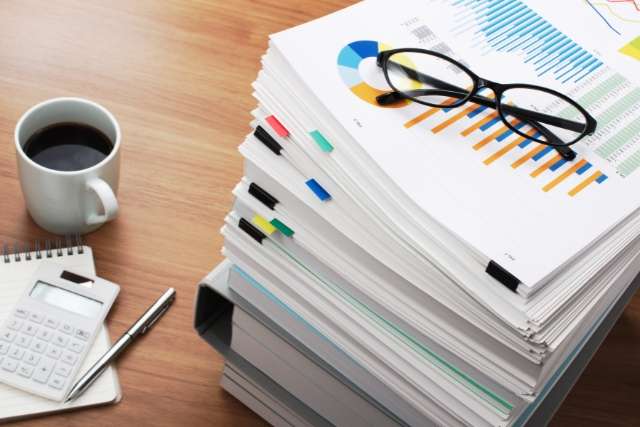
Content
Are you ready to unlock the power of data? In today's data-driven world, understanding the art and science of data collection is the key to informed decision-making and achieving your objectives.
This guide will walk you through the intricate data collection process, from its fundamental principles to advanced strategies and ethical considerations. Whether you're a business professional, researcher, or simply curious about the world of data, this guide will equip you with the knowledge and tools needed to harness the potential of data collection effectively.
What is Data Collection?
Data collection is the systematic process of gathering and recording information or data from various sources for analysis, interpretation, and decision-making. It is a fundamental step in research, business operations, and virtually every field where information is used to understand, improve, or make informed choices.
Key Elements of Data Collection
- Sources: Data can be collected from a wide range of sources, including surveys, interviews, observations, sensors, databases, social media, and more.
- Methods: Various methods are employed to collect data, such as questionnaires, data entry, web scraping, and sensor networks. The choice of method depends on the type of data, research objectives, and available resources.
- Data Types: Data can be qualitative (descriptive) or quantitative (numerical), structured (organized into a predefined format) or unstructured (free-form text or media), and primary (collected directly) or secondary (obtained from existing sources).
- Data Collection Tools: Technology plays a significant role in modern data collection, with software applications, mobile apps, sensors, and data collection platforms facilitating efficient and accurate data capture.
- Ethical Considerations: Ethical guidelines, including informed consent and privacy protection, must be followed to ensure that data collection respects the rights and well-being of individuals.
- Data Quality: The accuracy, completeness, and reliability of collected data are critical to its usefulness. Data quality assurance measures are implemented to minimize errors and biases.
- Data Storage: Collected data needs to be securely stored and managed to prevent loss, unauthorized access, and breaches. Data storage solutions range from on-premises servers to cloud-based platforms.
Importance of Data Collection in Modern Businesses
Data collection is of paramount importance in modern businesses for several compelling reasons:
- Informed Decision-Making: Collected data serves as the foundation for informed decision-making at all levels of an organization. It provides valuable insights into customer behavior, market trends, operational efficiency, and more.
- Competitive Advantage: Businesses that effectively collect and analyze data gain a competitive edge. Data-driven insights help identify opportunities, optimize processes, and stay ahead of competitors.
- Customer Understanding: Data collection allows businesses to better understand their customers, their preferences, and their pain points. This insight is invaluable for tailoring products, services, and marketing strategies.
- Performance Measurement: Data collection enables organizations to assess the performance of various aspects of their operations, from marketing campaigns to production processes. This helps identify areas for improvement.
- Risk Management: Businesses can use data to identify potential risks and develop strategies to mitigate them. This includes financial risks, supply chain disruptions, and cybersecurity threats.
- Innovation: Data collection supports innovation by providing insights into emerging trends and customer demands. Businesses can use this information to develop new products or services.
- Resource Allocation: Data-driven decision-making helps allocate resources efficiently. For example, marketing budgets can be optimized based on the performance of different channels.
Goals and Objectives of Data Collection
The goals and objectives of data collection depend on the specific context and the needs of the organization or research project. However, there are some common overarching objectives:
- Information Gathering: The primary goal is to gather accurate, relevant, and reliable information that addresses specific questions or objectives.
- Analysis and Insight: Collected data is meant to be analyzed to uncover patterns, trends, relationships, and insights that can inform decision-making and strategy development.
- Measurement and Evaluation: Data collection allows for the measurement and evaluation of various factors, such as performance, customer satisfaction, or market potential.
- Problem Solving: Data collection can be directed toward solving specific problems or challenges faced by an organization, such as identifying the root causes of quality issues.
- Monitoring and Surveillance: In some cases, data collection serves as a continuous monitoring or surveillance function, allowing organizations to track ongoing processes or conditions.
- Benchmarking: Data collection can be used for benchmarking against industry standards or competitors, helping organizations assess their performance relative to others.
- Planning and Strategy: Data collected over time can support long-term planning and strategy development, ensuring that organizations adapt to changing circumstances.
In summary, data collection is a foundational activity with diverse applications across industries and sectors. Its objectives range from understanding customers and making informed decisions to improving processes, managing risks, and driving innovation. The quality and relevance of collected data are pivotal in achieving these goals.
How to Plan Your Data Collection Strategy?
Before kicking things off, we'll review the crucial steps of planning your data collection strategy. Your success in data collection largely depends on how well you define your objectives, select suitable sources, set clear goals, and choose appropriate collection methods.
Defining Your Research Questions
Defining your research questions is the foundation of any effective data collection effort. The more precise and relevant your questions, the more valuable the data you collect.
- Specificity is Key: Make sure your research questions are specific and focused. Instead of asking, "How can we improve customer satisfaction?" ask, "What specific aspects of our service do customers find most satisfying or dissatisfying?"
- Prioritize Questions: Determine the most critical questions that will have the most significant impact on your goals. Not all questions are equally important, so allocate your resources accordingly.
- Alignment with Objectives: Ensure that your research questions directly align with your overall objectives. If your goal is to increase sales, your research questions should be geared toward understanding customer buying behaviors and preferences.
Identifying Key Data Sources
Identifying the proper data sources is essential for gathering accurate and relevant information. Here are some examples of key data sources for different industries and purposes.
- Customer Data: This can include customer demographics, purchase history, website behavior, and feedback from customer service interactions.
- Market Research Reports: Utilize industry reports, competitor analyses, and market trend studies to gather external data and insights.
- Internal Records: Your organization's databases, financial records, and operational data can provide valuable insights into your business's performance.
- Social Media Platforms: Monitor social media channels to gather customer feedback, track brand mentions, and identify emerging trends in your industry.
- Web Analytics: Collect data on website traffic, user behavior, and conversion rates to optimize your online presence.
Setting Clear Data Collection Goals
Setting clear and measurable goals is essential to ensure your data collection efforts remain on track and deliver valuable results. Goals should be:
- Specific: Clearly define what you aim to achieve with your data collection. For instance, increasing website traffic by 20% in six months is a specific goal.
- Measurable: Establish criteria to measure your progress and success. Use metrics such as revenue growth, customer satisfaction scores, or conversion rates.
- Achievable: Set realistic goals that your team can realistically work towards. Overly ambitious goals can lead to frustration and burnout.
- Relevant: Ensure your goals align with your organization's broader objectives and strategic initiatives.
- Time-Bound: Set a timeframe within which you plan to achieve your goals. This adds a sense of urgency and helps you track progress effectively.
Choosing Data Collection Methods
Selecting the correct data collection methods is crucial for obtaining accurate and reliable data. Your choice should align with your research questions and goals. Here's a closer look at various data collection methods and their practical applications.
Types of Data Collection Methods
Now, let's explore different data collection methods in greater detail, including examples of when and how to use them effectively:
Surveys and Questionnaires
Surveys and questionnaires are versatile tools for gathering data from a large number of respondents. They are commonly used for:
- Customer Feedback: Collecting opinions and feedback on products, services, and overall satisfaction.
- Market Research: Assessing market preferences, identifying trends, and evaluating consumer behavior.
- Employee Surveys: Measuring employee engagement, job satisfaction, and feedback on workplace conditions.
Example: If you're running an e-commerce business and want to understand customer preferences, you can create an online survey asking customers about their favorite product categories, preferred payment methods, and shopping frequency.
To enhance your data collection endeavors, check out Appinio, a modern research platform that simplifies the process and maximizes the quality of insights. Appinio offers user-friendly survey and questionnaire tools that enable you to effortlessly design surveys tailored to your needs. It also provides seamless integration with interview and observation data, allowing you to consolidate your findings in one place.
Discover how Appinio can elevate your data collection efforts. Book a demo today to unlock a world of possibilities in gathering valuable insights!
Interviews
Interviews involve one-on-one or group conversations with participants to gather detailed insights. They are particularly useful for:
- Qualitative Research: Exploring complex topics, motivations, and personal experiences.
- In-Depth Analysis: Gaining a deep understanding of specific issues or situations.
- Expert Opinions: Interviewing industry experts or thought leaders to gather valuable insights.
Example: If you're a healthcare provider aiming to improve patient experiences, conducting interviews with patients can help you uncover specific pain points and suggestions for improvement.
Observations
Observations entail watching and recording behaviors or events in their natural context. This method is ideal for:
- Behavioral Studies: Analyzing how people interact with products or environments.
- Field Research: Collecting data in real-world settings, such as retail stores, public spaces, or classrooms.
- Ethnographic Research: Immersing yourself in a specific culture or community to understand their practices and customs.
Example: If you manage a retail store, observing customer traffic flow and purchasing behaviors can help optimize store layout and product placement.
Document Analysis
Document analysis involves reviewing and extracting information from written or digital documents. It is valuable for:
- Historical Research: Studying historical records, manuscripts, and archives.
- Content Analysis: Analyzing textual or visual content from websites, reports, or publications.
- Legal and Compliance: Reviewing contracts, policies, and legal documents for compliance purposes.
Example: If you're a content marketer, you can analyze competitor blog posts to identify common topics and keywords used in your industry.
Web Scraping
Web scraping is the automated process of extracting data from websites. It's suitable for:
- Competitor Analysis: Gathering data on competitor product prices, descriptions, and customer reviews.
- Market Research: Collecting data on product listings, reviews, and trends from e-commerce websites.
- News and Social Media Monitoring: Tracking news articles, social media posts, and comments related to your brand or industry.
Example: If you're in the travel industry, web scraping can help you collect pricing data for flights and accommodations from various travel booking websites to stay competitive.
Social Media Monitoring
Social media monitoring involves tracking and analyzing conversations and activities on social media platforms. It's valuable for:
- Brand Reputation Management: Monitoring brand mentions and sentiment to address customer concerns or capitalize on positive feedback.
- Competitor Analysis: Keeping tabs on competitors' social media strategies and customer engagement.
- Trend Identification: Identifying emerging trends and viral content within your industry.
Example: If you run a restaurant, social media monitoring can help you track customer reviews, comments, and hashtags related to your establishment, allowing you to respond promptly to customer feedback and trends.
By understanding the nuances and applications of these data collection methods, you can choose the most appropriate approach to gather valuable insights for your specific objectives. Remember that a well-thought-out data collection strategy is the cornerstone of informed decision-making and business success.
How to Design Your Data Collection Instruments?
Now that you've defined your research questions, identified data sources, set clear goals, and chosen appropriate data collection methods, it's time to design the instruments you'll use to collect data effectively.
Design Effective Survey Questions
Designing survey questions is a crucial step in gathering accurate and meaningful data. Here are some key considerations:
- Clarity: Ensure that your questions are clear and concise. Avoid jargon or ambiguous language that may confuse respondents.
- Relevance: Ask questions that directly relate to your research objectives. Avoid unnecessary or irrelevant questions that can lead to survey fatigue.
- Avoid Leading Questions: Formulate questions that do not guide respondents toward a particular answer. Maintain neutrality to get unbiased responses.
- Response Options: Provide appropriate response options, including multiple-choice, Likert scales, or open-ended formats, depending on the type of data you need.
- Pilot Testing: Before deploying your survey, conduct pilot tests with a small group to identify any issues with question wording or response options.
Craft Interview Questions for Insightful Conversations
Developing interview questions requires thoughtful consideration to elicit valuable insights from participants:
- Open-Ended Questions: Use open-ended questions to encourage participants to share their thoughts, experiences, and perspectives without being constrained by predefined answers.
- Probing Questions: Prepare follow-up questions to delve deeper into specific topics or clarify responses.
- Structured vs. Semi-Structured Interviews: Decide whether your interviews will follow a structured format with predefined questions or a semi-structured approach that allows flexibility.
- Avoid Biased Questions: Ensure your questions do not steer participants toward desired responses. Maintain objectivity throughout the interview.
Build an Observation Checklist for Data Collection
When conducting observations, having a well-structured checklist is essential:
- Clearly Defined Variables: Identify the specific variables or behaviors you are observing and ensure they are well-defined.
- Checklist Format: Create a checklist format that is easy to use and follow during observations. This may include checkboxes, scales, or space for notes.
- Training Observers: If you have a team of observers, provide thorough training to ensure consistency and accuracy in data collection.
- Pilot Observations: Before starting formal data collection, conduct pilot observations to refine your checklist and ensure it captures the necessary information.
Streamline Data Collection with Forms and Templates
Creating user-friendly data collection forms and templates helps streamline the process:
- Consistency: Ensure that all data collection forms follow a consistent format and structure, making it easier to compare and analyze data.
- Data Validation: Incorporate data validation checks to reduce errors during data entry. This can include dropdown menus, date pickers, or required fields.
- Digital vs. Paper Forms: Decide whether digital forms or traditional paper forms are more suitable for your data collection needs. Digital forms often offer real-time data validation and remote access.
- Accessibility: Make sure your forms and templates are accessible to all team members involved in data collection. Provide training if necessary.
The Data Collection Process
Now that your data collection instruments are ready, it's time to embark on the data collection process itself. This section covers the practical steps involved in collecting high-quality data.
1. Preparing for Data Collection
Adequate preparation is essential to ensure a smooth data collection process:
- Resource Allocation: Allocate the necessary resources, including personnel, technology, and materials, to support data collection activities.
- Training: Train data collection teams or individuals on the use of data collection instruments and adherence to protocols.
- Pilot Testing: Conduct pilot data collection runs to identify and resolve any issues or challenges that may arise.
- Ethical Considerations: Ensure that data collection adheres to ethical standards and legal requirements. Obtain necessary permissions or consent as applicable.
2. Conducting Data Collection
During data collection, it's crucial to maintain consistency and accuracy:
- Follow Protocols: Ensure that data collection teams adhere to established protocols and procedures to maintain data integrity.
- Supervision: Supervise data collection teams to address questions, provide guidance, and resolve any issues that may arise.
- Documentation: Maintain detailed records of the data collection process, including dates, locations, and any deviations from the plan.
- Data Security: Implement data security measures to protect collected information from unauthorized access or breaches.
3. Ensuring Data Quality and Reliability
After collecting data, it's essential to validate and ensure its quality:
- Data Cleaning: Review collected data for errors, inconsistencies, and missing values. Clean and preprocess the data to ensure accuracy.
- Quality Checks: Perform quality checks to identify outliers or anomalies that may require further investigation or correction.
- Data Validation: Cross-check data with source documents or original records to verify its accuracy and reliability.
- Data Auditing: Conduct periodic audits to assess the overall quality of the collected data and make necessary adjustments.
4. Managing Data Collection Teams
If you have multiple team members involved in data collection, effective management is crucial:
- Communication: Maintain open and transparent communication channels with team members to address questions, provide guidance, and ensure consistency.
- Performance Monitoring: Regularly monitor the performance of data collection teams, identifying areas for improvement or additional training.
- Problem Resolution: Be prepared to promptly address any challenges or issues that arise during data collection.
- Feedback Loop: Establish a feedback loop for data collection teams to share insights and best practices, promoting continuous improvement.
By following these steps and best practices in the data collection process, you can ensure that the data you collect is reliable, accurate, and aligned with your research objectives. This lays the foundation for meaningful analysis and informed decision-making.
How to Store and Manage Data?
It's time to explore the critical aspects of data storage and management, which are pivotal in ensuring the security, accessibility, and usability of your collected data.
Choosing Data Storage Solutions
Selecting the proper data storage solutions is a strategic decision that impacts data accessibility, scalability, and security. Consider the following factors:
- Cloud vs. On-Premises: Decide whether to store your data in the cloud or on-premises. Cloud solutions offer scalability, accessibility, and automatic backups, while on-premises solutions provide more control but require significant infrastructure investments.
- Data Types: Assess the types of data you're collecting, such as structured, semi-structured, or unstructured data. Choose storage solutions that accommodate your data formats efficiently.
- Scalability: Ensure that your chosen solution can scale as your data volume grows. This is crucial for preventing storage bottlenecks.
- Data Accessibility: Opt for storage solutions that provide easy and secure access to authorized users, whether they are on-site or remote.
- Data Recovery and Backup: Implement robust data backup and recovery mechanisms to safeguard against data loss due to hardware failures or disasters.
Data Security and Privacy
Data security and privacy are paramount, especially when handling sensitive or personal information.
- Encryption: Implement encryption for data at rest and in transit. Use encryption protocols like SSL/TLS for communication and robust encryption algorithms for storage.
- Access Control: Set up role-based access control (RBAC) to restrict access to data based on job roles and responsibilities. Limit access to only those who need it.
- Compliance: Ensure that your data storage and management practices comply with relevant data protection regulations, such as GDPR, HIPAA, or CCPA.
- Data Masking: Use data masking techniques to conceal sensitive information in non-production environments.
- Monitoring and Auditing: Continuously monitor access logs and perform regular audits to detect unauthorized activities and maintain compliance.
Data Organization and Cataloging
Organizing and cataloging your data is essential for efficient retrieval, analysis, and decision-making.
- Metadata Management: Maintain detailed metadata for each dataset, including data source, date of collection, data owner, and description. This makes it easier to locate and understand your data.
- Taxonomies and Categories: Develop taxonomies or data categorization schemes to classify data into logical groups, making it easier to find and manage.
- Data Versioning: Implement data versioning to track changes and updates over time. This ensures data lineage and transparency.
- Data Catalogs: Use data cataloging tools and platforms to create a searchable inventory of your data assets, facilitating discovery and reuse.
- Data Retention Policies: Establish clear data retention policies that specify how long data should be retained and when it should be securely deleted or archived.
How to Analyze and Interpret Data?
Once you've collected your data, let's take a look at the process of extracting valuable insights from your collected data through analysis and interpretation.
Data Cleaning and Preprocessing
Data cleaning and preprocessing are essential steps to ensure that your data is accurate and ready for analysis.
- Handling Missing Data: Develop strategies for dealing with missing data, such as imputation or removal, based on the nature of your data and research objectives.
- Outlier Detection: Identify and address outliers that can skew analysis results. Consider whether outliers should be corrected, removed, or retained based on their significance.
- Normalization and Scaling: Normalize or scale data to bring it within a common range, making it suitable for certain algorithms and models.
- Data Transformation: Apply data transformations, such as logarithmic scaling or categorical encoding, to prepare data for specific types of analysis.
- Data Imbalance: Address class imbalance issues in datasets, particularly machine learning applications, to avoid biased model training.
Exploratory Data Analysis (EDA)
EDA is the process of visually and statistically exploring your data to uncover patterns, trends, and potential insights.
- Descriptive Statistics: Calculate basic statistics like mean, median, and standard deviation to summarize data distributions.
- Data Visualization: Create visualizations such as histograms, scatter plots, and heatmaps to reveal relationships and patterns within the data.
- Correlation Analysis: Examine correlations between variables to understand how they influence each other.
- Hypothesis Testing: Conduct hypothesis tests to assess the significance of observed differences or relationships in your data.
Statistical Analysis Techniques
Choose appropriate statistical analysis techniques based on your research questions and data types.
- Descriptive Statistics: Use descriptive statistics to summarize and describe your data, providing an initial overview of key features.
- Inferential Statistics: Apply inferential statistics, including t-tests, ANOVA, or regression analysis, to test hypotheses and draw conclusions about population parameters.
- Non-parametric Tests: Employ non-parametric tests when assumptions of normality are not met or when dealing with ordinal or nominal data.
- Time Series Analysis: Analyze time-series data to uncover trends, seasonality, and temporal patterns.
Data Visualization
Data visualization is a powerful tool for conveying complex information in a digestible format.
- Charts and Graphs: Utilize various charts and graphs, such as bar charts, line charts, pie charts, and heatmaps, to represent data visually.
- Interactive Dashboards: Create interactive dashboards using tools like Tableau, Power BI, or custom web applications to allow stakeholders to explore data dynamically.
- Storytelling: Use data visualization to tell a compelling data-driven story, highlighting key findings and insights.
- Accessibility: Ensure that data visualizations are accessible to all audiences, including those with disabilities, by following accessibility guidelines.
Drawing Conclusions and Insights
Finally, drawing conclusions and insights from your data analysis is the ultimate goal.
- Contextual Interpretation: Interpret your findings in the context of your research objectives and the broader business or research landscape.
- Actionable Insights: Identify actionable insights that can inform decision-making, strategy development, or future research directions.
- Report Generation: Create comprehensive reports or presentations that communicate your findings clearly and concisely to stakeholders.
- Validation: Cross-check your conclusions with domain experts or subject matter specialists to ensure accuracy and relevance.
By following these steps in data analysis and interpretation, you can transform raw data into valuable insights that drive informed decisions, optimize processes, and create new opportunities for your organization.
How to Report and Present Data?
Now, let's explore the crucial steps of reporting and presenting data effectively, ensuring that your findings are communicated clearly and meaningfully to stakeholders.
1. Create Data Reports
Data reports are the culmination of your data analysis efforts, presenting your findings in a structured and comprehensible manner.
- Report Structure: Organize your report with a clear structure, including an introduction, methodology, results, discussion, and conclusions.
- Visualization Integration: Incorporate data visualizations, charts, and graphs to illustrate key points and trends.
- Clarity and Conciseness: Use clear and concise language, avoiding technical jargon, to make your report accessible to a diverse audience.
- Actionable Insights: Highlight actionable insights and recommendations that stakeholders can use to make informed decisions.
- Appendices: Include appendices with detailed methodology, data sources, and any additional information that supports your findings.
2. Leverage Data Visualization Tools
Data visualization tools can significantly enhance your ability to convey complex information effectively. Top data visualization tools include:
- Tableau: Tableau offers a wide range of visualization options and interactive dashboards, making it a popular choice for data professionals.
- Power BI: Microsoft's Power BI provides powerful data visualization and business intelligence capabilities, suitable for creating dynamic reports and dashboards.
- Python Libraries: Utilize Python libraries such as Matplotlib, Seaborn, and Plotly for custom data visualizations and analysis.
- Excel: Microsoft Excel remains a versatile tool for creating basic charts and graphs, particularly for smaller datasets.
- Custom Development: Consider custom development for specialized visualization needs or when existing tools don't meet your requirements.
3. Communicate Findings to Stakeholders
Effectively communicating your findings to stakeholders is essential for driving action and decision-making.
- Audience Understanding: Tailor your communication to the specific needs and background knowledge of your audience. Avoid technical jargon when speaking to non-technical stakeholders.
- Visual Storytelling: Craft a narrative that guides stakeholders through the data, highlighting key insights and their implications.
- Engagement: Use engaging and interactive presentations or reports to maintain the audience's interest and encourage participation.
- Question Handling: Be prepared to answer questions and provide clarifications during presentations or discussions. Anticipate potential concerns or objections.
- Feedback Loop: Encourage feedback and open dialogue with stakeholders to ensure your findings align with their objectives and expectations.
Data Collection Examples
To better understand the practical application of data collection in various domains, let's explore some real-world examples, including those in the business context. These examples illustrate how data collection can drive informed decision-making and lead to meaningful insights.
Business Customer Feedback Surveys
Scenario: A retail company wants to enhance its customer experience and improve product offerings. To achieve this, they initiate customer feedback surveys.
Data Collection Approach:
- Survey Creation: The company designs a survey with specific questions about customer preferences, shopping experiences, and product satisfaction.
- Distribution: Surveys are distributed through various channels, including email, in-store kiosks, and the company's website.
- Data Gathering: Responses from thousands of customers are collected and stored in a centralized database.
Data Analysis and Insights:
- Customer Sentiment Analysis: Using natural language processing (NLP) techniques, the company analyzes open-ended responses to gauge customer sentiment.
- Product Performance: Analyzing survey data, the company identifies which products receive the highest and lowest ratings, leading to decisions on which products to improve or discontinue.
- Store Layout Optimization: By examining feedback related to in-store experiences, the company can adjust store layouts and signage to enhance customer flow and convenience.
Healthcare Patient Record Digitization
Scenario: A healthcare facility aims to transition from paper-based patient records to digital records for improved efficiency and patient care.
Data Collection Approach:
- Scanning and Data Entry: Existing paper records are scanned, and data entry personnel convert them into digital format.
- Electronic Health Record (EHR) Implementation: The facility adopts an EHR system to store and manage patient data securely.
- Continuous Data Entry: As new patient information is collected, it is directly entered into the EHR system.
Data Analysis and Insights:
- Patient History Access: Physicians and nurses gain instant access to patient records, improving diagnostic accuracy and treatment.
- Data Analytics: Aggregated patient data can be analyzed to identify trends in diseases, treatment outcomes, and healthcare resource utilization.
- Resource Optimization: Analysis of patient data allows the facility to allocate resources more efficiently, such as staff scheduling based on patient admission patterns.
Social Media Engagement Monitoring
Scenario: A digital marketing agency manages social media campaigns for various clients and wants to track campaign performance and audience engagement.
Data Collection Approach:
- Social Media Monitoring Tools: The agency employs social media monitoring tools to collect data on post engagement, reach, likes, shares, and comments.
- Custom Tracking Links: Unique tracking links are created for each campaign to monitor traffic and conversions.
- Audience Demographics: Data on the demographics of engaged users is gathered from platform analytics.
Data Analysis and Insights:
- Campaign Effectiveness: The agency assesses which campaigns are most effective in terms of engagement and conversion rates.
- Audience Segmentation: Insights into audience demographics help tailor future campaigns to specific target demographics.
- Content Strategy: Analyzing which types of content (e.g., videos, infographics) generate the most engagement informs content strategy decisions.
These examples showcase how data collection serves as the foundation for informed decision-making and strategy development across diverse sectors. Whether improving customer experiences, enhancing healthcare services, or optimizing marketing efforts, data collection empowers organizations to harness valuable insights for growth and improvement.
Ethical Considerations in Data Collection
Ethical considerations are paramount in data collection to ensure privacy, fairness, and transparency. Addressing these issues is not only responsible but also crucial for building trust with stakeholders.
Informed Consent
Obtaining informed consent from participants is an ethical imperative. Transparency is critical, and participants should fully understand the purpose of data collection, how their data will be used, and any potential risks or benefits involved. Consent should be voluntary, and participants should have the option to withdraw their consent at any time without consequences.
Consent forms should be clear and comprehensible, avoiding overly complex language or legal jargon. Special care should be taken when collecting sensitive or personal data to ensure privacy rights are respected.
Privacy Protection
Protecting individuals' privacy is essential to maintain trust and comply with data protection regulations. Data anonymization or pseudonymization should be used to prevent the identification of individuals, especially when sharing or publishing data. Data encryption methods should be implemented to protect data both in transit and at rest, safeguarding it from unauthorized access.
Strict access controls should be in place to restrict data access to authorized personnel only, and clear data retention policies should be established and adhered to, preventing unnecessary data storage. Regular privacy audits should be conducted to identify and address potential vulnerabilities or compliance issues.
Bias and Fairness in Data Collection
Addressing bias and ensuring fairness in data collection is critical to avoid perpetuating inequalities. Data collection methods should be designed to minimize potential biases, such as selection bias or response bias. Efforts should be made to achieve diverse and representative samples, ensuring that data accurately reflects the population of interest. Fair treatment of all participants and data sources is essential, with discrimination based on characteristics such as race, gender, or socioeconomic status strictly avoided.
If algorithms are used in data collection or analysis, biases that may arise from automated processes should be assessed and mitigated. Ethical reviews or expert consultations may be considered when dealing with sensitive or potentially biased data. By adhering to ethical principles throughout the data collection process, individuals' rights are protected, and a foundation for responsible and trustworthy data-driven decision-making is established.
Conclusion
Data collection is the cornerstone of informed decision-making and insight generation in today's data-driven world. Whether you're a business seeking to understand your customers better, a researcher uncovering valuable trends, or anyone eager to harness the power of data, this guide has equipped you with the essential knowledge and tools. Remember, ethical considerations are paramount, and the quality of data matters.
Furthermore, as you embark on your data collection journey, always keep in mind the impact and potential of the information you gather. Each data point is a piece of the puzzle that can help you shape strategies, optimize operations, and make a positive difference. Data collection is not just a task; it's a powerful tool that empowers you to unlock opportunities, solve challenges, and stay ahead in a dynamic and ever-changing landscape. So, continue to explore, analyze, and draw valuable insights from your data, and let it be your compass on the path to success.
How to Collect Data in Minutes?
Imagine having the power to conduct your own market research in minutes, without the need for a PhD in research. Appinio is the real-time market research platform that empowers you to get instant consumer insights, fueling your data-driven decisions. We've transformed market research from boring and intimidating to exciting and intuitive.
Here's why Appinio is your go-to platform:
- Lightning-Fast Insights: From questions to insights in minutes. When you need answers, Appinio delivers swiftly.
- User-Friendly: Our platform is so intuitive that anyone can use it; no research degree required.
- Global Reach: Define your target group from over 1200 characteristics and survey them in 90+ countries.
- Guided Expertise: Our dedicated research consultants will support you every step of the way, ensuring your research journey is seamless and effective.
Join the loop 💌
Be the first to hear about new updates, product news, and data insights. We'll send it all straight to your inbox.