What is Thematic Analysis and How to Do It Step-By-Step?
Appinio Research · 03.11.2023 · 33min read
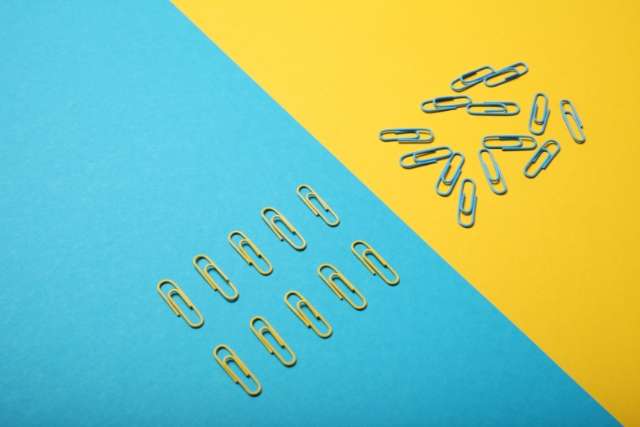
Content
Have you ever wondered how researchers make sense of the rich tapestry of qualitative data they gather from interviews, surveys, or textual sources? Thematic analysis serves as their guiding compass in unraveling the intricate stories within the data.
In this guide, we dive deep into thematic analysis, exploring its definition, purpose, applications, and step-by-step methodologies. Whether you're a seasoned researcher seeking to refine your qualitative analysis skills or a novice embarking on your research journey, this guide will equip you with the knowledge and tools needed to unlock the hidden meanings and patterns within your data.
What is Thematic Analysis?
Thematic analysis is a qualitative research method that involves systematically identifying, analyzing, and reporting patterns or themes within qualitative data. Its primary purpose is to uncover the underlying meanings and concepts embedded in textual, visual, or audio data.
Thematic analysis aims to provide a structured and comprehensive understanding of the content, enabling researchers to explore complex phenomena and answer research questions effectively.
Purpose of Thematic Analysis
- Data Exploration: Thematic analysis allows researchers to explore rich and unstructured qualitative data, such as interviews, focus group discussions, surveys, or written narratives. It helps reveal hidden insights that may not be apparent at first glance.
- Pattern Identification: The method is designed to identify patterns, recurring ideas, and common threads within the data. By categorizing data into themes, researchers can make sense of complex information.
- Contextual Understanding: Thematic analysis places a strong emphasis on understanding the context surrounding the data. It seeks to uncover the contextual factors that influence the emergence of specific themes.
- Interpretation and Explanation: It enables researchers to interpret and explain the meaning of the identified themes. Thematic analysis provides a deeper understanding of the phenomena under investigation.
- Theory Development: Thematic analysis can contribute to theory development by generating new concepts or refining existing theories. It helps researchers make theoretical connections based on empirical evidence.
- Practical Applications: Thematic analysis findings can have practical applications in various fields, such as healthcare, social sciences, business, and education. It informs decision-making, policy development, product improvement, and more.
In summary, the purpose of thematic analysis is to distill qualitative data into meaningful themes, providing researchers with a structured, interpretable, and contextually grounded understanding of the subject of study.
Importance of Thematic Analysis in Research
Thematic analysis holds significant importance in the field of research for several key reasons:
- Data Reduction and Organization: Qualitative data can be voluminous and unstructured. Thematic analysis acts as a powerful tool to reduce this complexity by organizing data into manageable themes and patterns. This reduction in data size makes it easier to extract meaningful insights.
- In-Depth Exploration: Thematic analysis enables researchers to conduct in-depth exploration of qualitative data. By identifying and examining themes, researchers can uncover nuances, contradictions, and intricacies within the data that may go unnoticed through other methods.
- Flexibility and Adaptability: Thematic analysis is highly flexible and adaptable to various research contexts and data types. It can be applied to textual data, visual data, audio data, and combinations thereof. Researchers can tailor the analysis to suit their specific research questions and objectives.
- Contextual Understanding: Thematic analysis places a strong emphasis on understanding the context in which data is generated. This contextual understanding is essential for accurate interpretation and meaningful insights.
- Theory Development and Testing: Thematic analysis can contribute to theory development by identifying patterns and concepts that inform or extend existing theories. It also allows researchers to test the applicability of theoretical frameworks in real-world settings.
- Practical Applications: The findings of thematic analysis have practical applications in diverse fields. They inform decision-making, guide policy development, drive product improvements, and provide valuable insights for addressing real-world challenges.
- Interdisciplinary Relevance: Thematic analysis transcends disciplinary boundaries, making it applicable in fields such as psychology, sociology, anthropology, education, healthcare, marketing, and more. Its interdisciplinary relevance enhances its utility in research.
In summary, thematic analysis plays a pivotal role in research by facilitating the systematic exploration and interpretation of qualitative data, leading to a deeper understanding of complex phenomena and informing decision-making and theory development across various domains.
How to Prepare for Thematic Analysis?
Before you embark on your thematic analysis journey, thorough preparation is vital. We'll delve into the main steps involved in getting your qualitative data ready for analysis.
1. Data Collection and Selection
Data collection is the foundation of any qualitative research project. You need to carefully plan, gather, and select your data to ensure it aligns with your research objectives.
- Research Goals: Clearly define your research questions or objectives. Your data should directly relate to what you want to explore or understand.
- Data Sources: Identify the sources of your qualitative data. Common sources include interviews, focus groups, surveys, field notes, or even existing documents and texts.
- Sampling: Decide on your sampling strategy. Will you use purposive sampling to select specific participants or content, or will you opt for more random sampling methods?
- Data Richness: Ensure your data is rich and comprehensive enough to answer your research questions. Collect enough data to reach data saturation, where new information or themes stop emerging.
2. Data Cleaning and Organization
Once you have your qualitative data in hand, the next step is data cleaning and organization. This process ensures that your data is in a usable format and is structured for efficient analysis.
- Transcription: If your data is in the form of interviews or recorded conversations, you may need to transcribe them. Accurate transcription is crucial for maintaining the integrity of the data.
- Data Format: Standardize the format of your data. This includes ensuring consistent date and time formats, naming conventions, and file organization.
- Data Validation: Check for data accuracy and consistency. Address any discrepancies or errors that may have arisen during data collection.
- Data Management: Organize your data systematically. Create a clear file structure, labeling, and version control to prevent data mix-ups or loss.
3. Choose the Right Software Tools
The choice of software tools for your thematic analysis can significantly impact the efficiency and effectiveness of your analysis process. Here's what you need to consider:
- Analysis Goals: Determine your specific analysis goals. Different software options may be better suited for certain types of projects or research questions.
- Ease of Use: Evaluate the user-friendliness of the software. Consider your team's familiarity with the tool and the learning curve involved.
- Collaboration Features: If you're working with a team, look for software that supports collaboration, allowing multiple researchers to work on the same project simultaneously.
- Data Import and Export: Ensure that the software can handle the data formats you are working with and provides robust import and export capabilities.
- Support and Training: Consider the availability of support resources, such as tutorials, user forums, and customer support, to assist you in case you encounter issues during analysis.
Some popular software options for thematic analysis include NVivo, ATLAS.ti, MAXQDA, and Dedoose. Each has its own strengths and features, so it's essential to choose the one that best fits your project's needs.
By carefully preparing your data, cleaning and organizing it effectively, and selecting the right software tools, you'll set a solid foundation for a successful thematic analysis. These steps ensure that you have high-quality data that can be analyzed efficiently and accurately, leading to meaningful insights for your research.
How to Do Thematic Analysis?
Thematic analysis involves a systematic process of identifying, analyzing, and reporting patterns or themes within qualitative data. In this section, we'll explore each step in detail, guiding you through the process of conducting thematic analysis effectively.
1. Familiarize Yourself with the Data
The initial step in thematic analysis is to become intimately acquainted with your qualitative data. This process, known as familiarization with data, allows you to gain a deep understanding of the content and context.
- Multiple Readings: Begin by reading through your data numerous times. This repeated exposure helps you become familiar with the nuances and intricacies of the material.
- Note-Taking: Take notes as you read. Document your initial thoughts, observations, and any patterns or ideas that emerge during this phase.
- Maintain an Open Mind: Avoid preconceived notions or biases. Approach the data with an open mind to allow for unbiased exploration.
- Identify Interesting Features: Look for exciting features, such as recurrent phrases, significant events, or notable trends within the data.
Familiarization sets the stage for the subsequent steps, as it enables you to approach the data with a fresh perspective and a foundation of knowledge.
2. Generate Initial Codes
Once you're familiar with the data, the next step is generating initial codes. Codes are labels or tags assigned to specific portions of text that capture the essence of what's being expressed.
- Start Small: Begin by coding smaller sections of data, such as sentences or paragraphs. Focus on breaking down the data into manageable units.
- Use In-Vivo Codes: Whenever possible, use in-vivo codes, which are codes that use the participants' own words. This helps maintain the authenticity of the data.
- Stay Close to the Data: Keep your codes closely tied to the content of the data. Avoid overly abstract or generalized labels.
- Constant Comparison: Continuously compare new data segments with existing codes to ensure consistency and relevance.
- Document Your Codebook: Create a codebook or list that outlines the codes you've generated and their definitions. This document will serve as a reference throughout your analysis.
Generating initial codes is a fundamental step that involves systematically dissecting the data into meaningful elements, setting the stage for subsequent theme development.
3. Search for Themes
With a set of initial codes in hand, it's time to move on to searching for themes. Themes are overarching patterns or recurring ideas that emerge from the coded data.
- Pattern Recognition: Look for patterns in the codes. Identify codes that appear frequently or codes that seem conceptually related.
- Grouping Codes: Start grouping codes together based on their similarities or connections. This process forms the basis for theme development.
- Stay Open to New Themes: Be open to the possibility of new themes emerging as you continue your analysis. Themes may evolve or shift as you delve deeper into the data.
- Subthemes: Recognize that themes can have subthemes, providing a hierarchical structure to your analysis.
Searching for themes is a dynamic process that involves organizing and categorizing codes to uncover the underlying patterns and meanings within the data.
4. Review and Define Themes
Once you've identified potential themes, the next step is to review and define themes more rigorously. This phase ensures that your themes accurately represent the patterns in your data.
- Refinement: Refine and clarify your themes. Review them to ensure they align with the data and accurately capture the essence of the content.
- Definition: Provide clear definitions for each theme. What does each theme represent, and how does it relate to the data?
- Validation: Seek validation from colleagues or peers. Discuss your themes with others to ensure they are robust and well-defined.
- Naming Themes: Give each theme a concise and descriptive name that encapsulates its meaning.
Reviewing and defining themes is a crucial step in the thematic analysis process, as it ensures the accuracy and validity of your findings.
5. Write and Describe Themes
With well-defined themes in hand, it's time to write and describe themes in greater detail. This step involves fleshing out the themes with supporting evidence from your data.
- Quote Integration: Include quotes or excerpts from the data that exemplify each theme. These quotes serve as concrete examples of the theme in action.
- Narrative Development: Develop a narrative around each theme. Explain its significance and relevance within the context of your research.
- Contextual Understanding: Consider the broader context in which each theme exists. How do these themes contribute to the overall understanding of your research questions?
- Illustrative Examples: Provide multiple examples within each theme to demonstrate its consistency and depth.
Writing and describing themes is where the richness of your analysis comes to life, allowing readers to grasp the significance of the patterns you've uncovered.
6. Report Results
The final step in thematic analysis is reporting results. This involves presenting your findings in a clear and structured manner.
- Structure Your Report: Organize your report according to your research objectives, themes, and supporting evidence.
- Narrative Flow: Create a narrative flow that guides the reader through your analysis process, from data familiarization to theme development.
- Visual Aids: Consider using visual aids such as tables, charts, or graphs to enhance the presentation of your themes and findings.
- Discussion: Discuss the implications of your themes in the context of your research questions or objectives. What do these themes reveal, and how do they contribute to the broader understanding of your topic?
- Conclusion: Summarize your findings and their significance. Offer suggestions for future research or practical applications if applicable.
Reporting results effectively ensures that your thematic analysis is not only comprehensive but also accessible to your target audience, whether it's fellow researchers, stakeholders, or the broader community.
Thematic Analysis Approaches
Thematic analysis is a flexible method that can be approached in different ways based on your research goals and the nature of your data. In this section, we'll explore three primary approaches to thematic analysis: inductive thematic analysis, deductive thematic analysis, and reflexive thematic analysis. Each approach has its own unique characteristics and applications.
Inductive Thematic Analysis
Inductive thematic analysis is characterized by its bottom-up, data-driven approach. In this approach, you start without predefined themes or theories. Instead, you allow themes to emerge organically from your data.
Process:
- Data Familiarization: Begin by immersing yourself in the data, reading and re-reading it multiple times to develop a deep understanding.
- Open Coding: Start coding the data without any preconceived ideas. Codes emerge directly from the data, capturing concepts and patterns as they appear.
- Code Grouping: Group similar codes together, gradually forming initial themes. These themes are derived solely from the data and may evolve as you progress.
- Theme Definition: Define and refine the emerging themes. Ensure they accurately represent the patterns and concepts within your data.
- Review and Validation: Continuously review and validate the themes with colleagues or peer researchers. This iterative process enhances the trustworthiness of the analysis.
Example: Imagine conducting interviews with employees about their experiences in the workplace. Through inductive thematic analysis, you may find that themes like "Work-Life Balance Challenges" and "Employee Empowerment" emerge from the interviews, even though you had no preconceived notions about these topics.
Deductive Thematic Analysis
Deductive thematic analysis, in contrast, begins with predefined themes or theories based on existing research or theoretical frameworks. This approach is particularly useful when you want to test specific hypotheses or apply existing concepts to your data.
Process:
- Theory or Framework Selection: Start by selecting a theoretical framework or pre-existing themes that align with your research objectives.
- Data Collection: Gather data with these predefined themes or theories in mind. Your data collection process is guided by the established concepts.
- Initial Coding: Code your data according to the predefined themes. This involves assigning data segments to specific categories based on the chosen framework.
- Theme Refinement: Refine and adapt the predefined themes as you analyze the data. You may discover nuances or subthemes that were not initially accounted for.
- Validation: Seek validation from peers or experts to ensure the adapted themes accurately represent the data.
Example: Suppose you're studying customer feedback on a new product launch. You begin with predefined themes like "Product Usability" and "Customer Satisfaction" based on established criteria for evaluating products. As you analyze the data, you refine these themes and add subthemes like "User Interface Design" and "Product Performance."
Reflexive Thematic Analysis
Reflexive thematic analysis emphasizes the researcher's active role in shaping the analysis. It is often used in interpretive and intuitive research paradigms, acknowledging that the researcher's subjectivity plays a significant role in the analysis process.
Process:
- Engage Reflexively: Acknowledge your own perspectives, biases, and preconceptions. Be aware of how your background and experiences influence the analysis.
- Data Immersion: Immerse yourself in the data while considering your own positionality. How do your personal experiences and beliefs intersect with the data?
- Coding with Reflexivity: Code the data while reflecting on your own interpretive lens. How does your perspective shape the codes and themes you identify?
- Constant Reflexivity: Continuously engage in reflexivity throughout the analysis process. Be open to adjusting your interpretations based on ongoing self-awareness.
- Interpretation: Interpret the themes within the context of both the data and your reflexive insights. Recognize the co-construction of meaning between you as the researcher and the data.
Example: In a study on cultural perceptions of healthcare, you, as the researcher, openly acknowledge your cultural background and experiences. This reflexivity prompts you to recognize nuances in the data related to cultural sensitivities that might have been overlooked otherwise. Themes related to "Cultural Health Practices" and "Healthcare Access Barriers" are informed by both the data and your reflexive insights.
These three approaches to thematic analysis offer flexibility in how you approach your data. Your choice of approach should align with your research objectives, the nature of your data, and your epistemological stance as a researcher. Whether you start with a blank slate (inductive), apply existing theories (deductive), or embrace reflexivity, thematic analysis can be tailored to suit your research needs.
Data Analysis Techniques
Thematic analysis can be conducted using various data analysis techniques, each with its advantages and considerations. In this section, we'll delve into the three primary data analysis techniques for thematic analysis: manual coding, using qualitative data analysis software, and comparison with quantitative analysis.
Manual Coding
Manual coding involves the process of reviewing your qualitative data and assigning codes to segments of text that represent specific concepts or themes. While it may be more time-consuming than using software tools, manual coding offers a deep and intimate understanding of your data.
Process:
- Data Familiarization: Begin by thoroughly immersing yourself in the data. Read through it multiple times to gain a comprehensive understanding of the content.
- Code Generation: Start identifying meaningful segments in the data and assign relevant codes to them. Codes should capture the essence of what is being expressed.
- Codebook Development: Create a codebook that documents all the codes you've generated along with their definitions. This serves as a reference throughout the analysis.
- Code Sorting and Grouping: Organize and group codes into potential themes based on similarities or connections between codes.
- Theme Development: Review and refine the themes that emerge from the grouped codes. Ensure they accurately represent the patterns in your data.
- Validation: Seek validation from colleagues or peer researchers to enhance the trustworthiness of the analysis.
Manual coding allows for a meticulous examination of the data, ensuring a deep and nuanced understanding of the content. It's especially valuable when you have a smaller dataset or want to maintain a high level of researcher involvement in the analysis.
Using Qualitative Data Analysis Software
Qualitative data analysis software provides tools and features to streamline the coding and analysis process, making it more efficient and collaborative.
Some of the top tools used for thematic analysis include:
- Appinio: A real-time market research platform that excels in providing fast access to consumer insights. With a focus on user experience and the ability to define precise target groups, Appinio helps you make data-driven decisions seamlessly and quickly, making it an exciting and intuitive choice for thematic analysis.
- NVivo: is a widely used software tool that offers a range of features for qualitative analysis, including coding, data visualization, and collaboration.
- ATLAS.ti: is known for its user-friendly interface and robust coding and analysis capabilities. It allows for the systematic organization of codes and themes.
- MAXQDA: provides a comprehensive suite of tools for qualitative analysis, including advanced text coding, multimedia analysis, and robust reporting options.
- Dedoose: is a web-based application designed for qualitative and mixed-methods research. It offers real-time collaboration features and intuitive coding.
To get started with these tools, all you have to do is:
- Data Import: Import your qualitative data into the software. This can include text, audio, video, or other forms of qualitative data.
- Coding: Use the software's coding features to assign codes to segments of your data. You can create a coding structure, code hierarchy, and attach memos.
- Theme Development: Organize and analyze your codes to identify themes. Many software tools offer tools for visualizing themes and subthemes.
- Data Querying: Use the software to search for specific codes or themes within your data. This can help you identify patterns and relationships.
- Collaboration: If working with a team, collaborate in real-time within the software, making it easier to manage and validate codes and themes.
Using qualitative data analysis software can significantly speed up the coding and analysis process, especially with larger datasets. It also enhances the organization and management of your data, making it easier to revisit and revise your analysis.
Thematic Analysis vs Quantitative Analysis
Thematic analysis is a qualitative research method, but it can be valuable when used in conjunction with quantitative analysis. Here's how thematic analysis compares to quantitative analysis.
Thematic Analysis
- Qualitative method
- Focuses on exploring meanings, patterns, and themes in qualitative data.
- Involves coding, categorizing, and interpreting textual or visual data.
- Emphasizes rich, context-specific insights.
- Typically involves smaller sample sizes.
- Subjective and context-dependent.
Quantitative Analysis
- Quantitative method
- Focuses on numerical data, statistics, and generalizability.
- Involves structured surveys, experiments, or data collection instruments.
- Emphasizes statistical relationships and patterns.
- Typically involves larger sample sizes.
- Objective and aims for generalizability.
Thematic vs Quantitative Analysis Comparison
- Complementarity: Thematic analysis and quantitative analysis can complement each other. Qualitative analysis provides depth and context, while quantitative analysis offers breadth and statistical significance.
- Mixed-Methods Research: Researchers often employ mixed-methods research, combining both qualitative and quantitative approaches to gain a comprehensive understanding of a research question.
- Sequential or Concurrent: Researchers may choose to conduct thematic analysis before or after quantitative analysis, depending on the research design and objectives.
For example, in a healthcare study, qualitative thematic analysis may be used to understand patients' experiences and preferences (qualitative), while quantitative analysis can assess the effectiveness of a new treatment based on numerical outcomes (quantitative). These approaches together provide a holistic view of the research question.
How to Ensure Thematic Analysis Quality?
Ensuring the quality and rigor of your thematic analysis is essential to maintain the validity and trustworthiness of your findings. In this section, we'll explore three key aspects of quality assurance in thematic analysis: trustworthiness and credibility, inter-coder reliability, and addressing bias and reflexivity.
Trustworthiness and Credibility
Trustworthiness and credibility refer to the extent to which your thematic analysis can be considered reliable and valid. Establishing trustworthiness and credibility is crucial to ensure that your findings accurately represent the data and can withstand scrutiny.
To ensure trustworthiness and credibility:
- Member Checking: Seek feedback from participants to ensure that your analysis aligns with their perspectives and experiences.
- Peer Debriefing: Engage with colleagues or experts in the field to discuss your analysis process and findings. Their insights can help identify any potential biases or oversights.
- Audit Trail: Maintain a detailed record of your analysis process, including coding decisions, codebook development, and theme generation. This audit trail serves as a transparent documentation of your work.
- Triangulation: Use multiple sources of data or methods to validate your findings. Triangulation can involve comparing data from interviews, observations, and documents to identify converging themes.
- Peer Review: Submit your analysis and findings for peer review in academic or professional settings. Peer reviewers can provide valuable feedback and validation.
- Clear Reporting: Ensure that your research report or article clearly and transparently documents your analysis process, including the steps taken to establish trustworthiness.
By implementing these methods, you enhance the trustworthiness and credibility of your thematic analysis, increasing its validity and reliability.
Inter-Coder Reliability
Inter-coder reliability is the degree of agreement between different coders or researchers when coding the same data. It is a measure of consistency and ensures that your analysis is not overly influenced by individual subjectivity.
To establish inter-coder reliability:
- Coding Training: Train coders or researchers in the coding process, ensuring they understand the codebook and coding guidelines.
- Coding Samples: Have multiple coders independently code a sample of your data. This sample should represent the diversity of your dataset.
- Calculate Agreement: Calculate inter-coder agreement using a statistical measure such as Cohen's Kappa or percentage agreement. This measures the level of agreement between coders.
- Discuss Discrepancies: When discrepancies arise, convene coder meetings to discuss and resolve differences. This may involve refining code definitions or guidelines.
- Repeat Coding: After resolving discrepancies, have coders recode the data to assess improved inter-coder reliability.
- Ongoing Monitoring: Maintain constant communication and monitoring among coders to ensure consistency throughout the analysis process.
Establishing inter-coder reliability is crucial when working with a team of coders or researchers. It minimizes the risk of individual biases and subjectivity affecting the analysis.
Addressing Bias and Reflexivity
Bias and reflexivity acknowledgment and management are integral parts of maintaining the quality and rigor of thematic analysis.
Bias
Researchers bring their own perspectives, beliefs, and experiences to the analysis process, which can introduce bias into the interpretation of data. To address bias:
- Engage in reflexivity by regularly reflecting on your own positionality and potential biases.
- Maintain transparency by documenting your reflexive insights and how they may influence your analysis.
- Seek feedback from peers or colleagues to identify and mitigate bias in your analysis.
Reflexivity
Reflexivity involves recognizing and acknowledging the role of the researcher in shaping the analysis process and findings. Researchers should:
- Be aware of their assumptions and preconceptions and how these may impact their interpretation.
- Consider how their background, experiences, and cultural context influence their understanding of the data.
- Use reflexivity to enhance the depth and validity of their analysis by recognizing and addressing their subjectivity.
By addressing bias and embracing reflexivity, researchers can conduct a more transparent and rigorous thematic analysis, leading to more credible and valid findings.
Thematic Analysis Challenges
Thematic analysis, like any research method, comes with its own set of challenges. We'll explore three common challenges researchers may encounter during thematic analysis: data overload, maintaining consistency, and subjectivity and interpretation.
Data Overload
Data overload occurs when you have a large volume of qualitative data to analyze, making it challenging to manage and extract meaningful patterns. To address data overload:
- Chunking Data: Break the data into manageable chunks or segments for analysis. This helps prevent feeling overwhelmed.
- Prioritization: Focus on the most relevant or central data that directly relates to your research questions or objectives.
- Use of Software: Consider using qualitative data analysis software to assist with data organization and coding efficiency.
Maintaining Consistency
Maintaining consistency throughout the analysis process is crucial to ensure that codes and themes are applied consistently across the dataset. To maintain consistency:
- Develop a clear codebook with well-defined code definitions and examples.
- Regularly check in with coding team members to address any inconsistencies or questions.
- Use regular team meetings or discussions to clarify interpretations and ensure a shared understanding.
Subjectivity and Interpretation
Subjectivity and interpretation are inherent to thematic analysis, as researchers actively engage in interpreting data. To address subjectivity:
- Engage in reflexivity to acknowledge and manage your subjectivity and biases.
- Seek external validation or peer input to challenge or confirm your interpretations.
- Use transparency in reporting to clarify your interpretive stance and decision-making process.
By recognizing and addressing these common challenges, researchers can navigate the complexities of thematic analysis more effectively and produce robust, high-quality results.
Thematic Analysis Applications
Thematic analysis is a versatile qualitative research method widely applied in various fields and contexts. Its flexibility makes it suitable for exploring a wide range of research questions and topics.
Healthcare Research
Thematic analysis is frequently used in healthcare research to explore patients' experiences, healthcare provider perspectives, and healthcare policy analysis. Researchers in this field use thematic analysis to uncover themes related to patient satisfaction, healthcare disparities, the impact of treatments, and more. For example, a study might employ thematic analysis to understand the emotional challenges faced by cancer patients during their treatment journey, leading to the identification of themes like "Emotional Resilience" and "Support Systems."
Social Sciences
In the social sciences, thematic analysis helps researchers examine complex social phenomena and human behaviors. It is employed in studies related to sociology, psychology, anthropology, and education. Researchers use thematic analysis to explore themes in narratives, interviews, focus groups, and surveys. For instance, in educational research, thematic analysis can reveal themes in teacher-student interactions, leading to insights into classroom dynamics and pedagogical approaches.
Market Research
Thematic analysis is valuable in market research to extract insights from consumer feedback, product reviews, and focus group discussions. Researchers analyze themes in customer opinions to inform product development, marketing strategies, and customer experience improvements. For example, in analyzing online product reviews, thematic analysis can uncover themes like "Product Reliability" and "Customer Service Satisfaction," guiding companies in enhancing their offerings.
Psychology and Counseling
In psychology and counseling, thematic analysis is utilized to explore qualitative data from interviews, therapy sessions, or written narratives. It aids in understanding psychological processes, coping mechanisms, and therapeutic outcomes. Researchers might use thematic analysis to identify themes related to mental health stigma reduction or recovery narratives in individuals with mental health challenges.
Policy Analysis
Thematic analysis plays a critical role in policy analysis by extracting key themes from policy documents, legislative texts, or public opinion. Researchers can use thematic analysis to uncover themes related to policy effectiveness, public perception, and policy impact assessment. For instance, in analyzing environmental policies, themes like "Sustainability Goals" and "Community Engagement" may emerge, informing policymakers about areas of focus.
Examples of Thematic Analysis in Research
To gain a more comprehensive understanding of how thematic analysis is applied in research, let's explore several detailed examples across different fields and research contexts.
Example 1: Exploring Mental Health Stigma
Research Question: What are the key themes in narratives of individuals who have experienced mental health stigma?
Data: In-depth interviews with individuals who have faced mental health stigma.
Thematic Analysis Process:
- Data Familiarization: Researchers immerse themselves in interview transcripts, noting significant statements related to mental health stigma.
- Initial Coding: Initial codes are generated, including "Negative Stereotypes," "Experiences of Discrimination," and "Coping Strategies."
- Theme Development: Codes are grouped into broader themes, leading to the emergence of themes like "Internalization of Stigma" and "Empowerment through Advocacy."
- Refinement and Definition: Each theme is refined and defined with illustrative quotes to capture the nuances of participants' experiences.
- Interpretation: Researchers interpret the findings, highlighting the impact of stigma on mental health and the importance of support systems.
This thematic analysis sheds light on the multifaceted nature of mental health stigma and offers insights into the coping mechanisms individuals employ to navigate these challenges.
Example 2: Evaluating Customer Feedback for a Tech Product
Research Question: What themes emerge from an analysis of customer feedback for a new smartphone model?
Data: Analysis of online customer reviews and feedback for a recently launched smartphone.
Thematic Analysis Process:
- Data Collection: Collect customer reviews and comments from online platforms, aggregating a substantial dataset.
- Data Cleaning: Remove duplicates and irrelevant data to streamline the analysis process.
- Coding: Codes are generated for common sentiments and topics found in the reviews, such as "Camera Quality," "Battery Life," and "User-Friendly Interface."
- Theme Development: Codes are organized into overarching themes, revealing key themes like "Performance and Speed," "Durability Concerns," and "User Experience."
- Visualization: Visual aids such as word clouds and frequency distributions are used to present the prevalence of themes in customer feedback.
- Implications: The analysis highlights areas for product improvement and informs marketing strategies based on customer perceptions.
This thematic analysis of customer feedback provides valuable insights into the strengths and weaknesses of the smartphone model, guiding product development and marketing efforts.
Example 3: Analyzing Qualitative Data in Educational Research
Research Question: What themes emerge from open-ended survey responses regarding the challenges of remote learning during the COVID-19 pandemic?
Data: Qualitative data collected from open-ended survey responses of students and educators.Thematic Analysis Process:
- Data Organization: Survey responses are organized for systematic analysis.
- Initial Coding: Codes are generated for recurring issues, such as "Technology Challenges," "Lack of Social Interaction," and "Time Management."
- Theme Development: Codes are grouped into overarching themes, resulting in themes like "Digital Divide" and "Adaptive Teaching Strategies."
- Subtheme Identification: Subthemes may emerge within larger themes, providing a more detailed understanding of specific issues.
- Contextual Analysis: The analysis considers the broader context of the pandemic's impact on education, including policy implications and pedagogical adaptations.
This thematic analysis of survey responses offers insights into the unique challenges faced by students and educators during the pandemic, informing educational policies and strategies.
These examples showcase the adaptability and effectiveness of thematic analysis in uncovering meaningful patterns and themes across diverse research contexts. Whether exploring personal experiences, customer feedback, or educational challenges, thematic analysis serves as a versatile qualitative research method that provides valuable insights and informs decision-making.
Conclusion for Thematic Analysis
Thematic analysis is a versatile and powerful method that helps researchers uncover patterns and themes within qualitative data. By following the steps outlined in this guide, you can embark on your journey of discovery and gain deeper insights into the world of qualitative research.
Remember, whether you're studying people's experiences, analyzing customer feedback, or exploring social phenomena, thematic analysis offers a structured approach to make sense of complex data. It's a valuable tool for researchers across diverse fields, providing a clear path to understanding, interpretation, and meaningful insights. So, as you venture into the realm of thematic analysis, embrace the richness of your data and let it tell its story. Your research journey has just begun, and the possibilities are boundless.
How to Conduct Thematic Analysis in Minutes?
In the world of thematic analysis, Appinio shines as a real-time market research platform, revolutionizing the way you gain consumer insights. Say goodbye to long, tedious research processes and embrace the exciting and intuitive world of real-time data-driven decisions.
Here's why Appinio is your go-to partner for thematic analysis:
- Lightning-Fast Insights: From formulating questions to obtaining actionable insights, Appinio delivers results in minutes. When you need answers pronto, we've got you covered.
- User-Friendly Excellence: No need for a Ph.D. in research. Our platform is designed to be so intuitive that anyone can navigate it with ease, empowering you to take control of your market research.
- Global Reach, Local Precision: With access to over 90 countries and the ability to define your target group from 1200+ characteristics, Appinio ensures that your thematic analysis is not only insightful but also globally relevant.
Join the loop 💌
Be the first to hear about new updates, product news, and data insights. We'll send it all straight to your inbox.