What is Sampling Bias? Definition, Types, Examples
Appinio Research · 10.10.2023 · 22min read
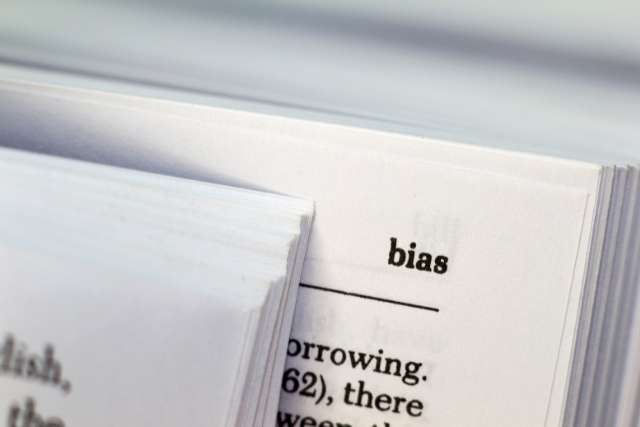
Content
Ever wondered why research findings sometimes seem to miss the mark or why data-driven decisions occasionally go awry? The answer often lies in an elusive yet powerful culprit: sampling bias. In this comprehensive guide, we delve deep into the world of sampling bias, uncovering its types, causes, and far-reaching impacts.
From detecting bias in your data to mastering techniques for minimizing it, we'll equip you with the tools and insights needed to ensure your research and analyses are as accurate and reliable as possible. So, let's jump right in and decode the intricacies of sampling bias, one layer at a time.
What is Sampling Bias?
Sampling bias occurs when the individuals or elements selected for a study are not truly random and do not accurately represent the entire population you are interested in studying. It introduces a systematic error into your research, as certain groups or characteristics are overrepresented or underrepresented in your sample. Simply put, sampling bias is like trying to understand a diverse group of people by only talking to a handful of them.
Why is Sampling Bias Important?
Sampling bias matters because it can lead to incorrect conclusions and misguided decisions. When your sample doesn't reflect the true characteristics of the population, you may end up with results that are misleading or even completely wrong. This can have severe consequences in fields such as healthcare, market research, and social sciences. For example, a biased sample in a medical study could lead to the development of ineffective treatments or misdiagnoses.
Types of Sampling Bias
Sampling bias can take various forms, each with its own unique characteristics and implications for research and data analysis. Let's explore these types in greater detail:
Non-Probability Sampling Bias
Non-probability sampling methods are susceptible to various types of bias because they do not involve random selection. Here are the key non-probability sampling biases:
Convenience Sampling Bias
Convenience sampling involves selecting individuals or elements that are easiest to access or reach. Researchers often choose participants based on their proximity, availability, or willingness to participate.
Impact: Convenience sampling can lead to a sample that is not representative of the larger population. Individuals who are more accessible may have different characteristics or experiences than those who are less accessible. This type of bias can result in skewed results and limited generalizability.
Judgmental Sampling Bias
Judgmental sampling, also known as purposive or selective sampling, occurs when researchers use their judgment to select participants or elements. They choose individuals based on specific criteria, often with the belief that these criteria are relevant to the research.
Impact: Judgmental sampling can introduce subjectivity into the sample selection process. Researchers may unintentionally favor individuals who align with their preconceived notions or hypotheses. This bias can lead to results that confirm researchers' expectations rather than providing an objective understanding of the population.
Quota Sampling Bias
Quota sampling involves selecting individuals to meet predefined quotas based on specific characteristics, such as age, gender, or income. Researchers aim to create a sample that mirrors the demographic composition of the population.
Impact: While quota sampling attempts to ensure diversity in the sample, it can still introduce bias if the quotas are not based on random criteria. If the criteria for quota assignment are not well-defined or are influenced by researchers' judgments, the resulting sample may not accurately represent the population.
Purposive Sampling Bias
Purposive sampling is similar to judgmental sampling but involves selecting participants based on specific, predetermined criteria that align with the research objectives. Researchers intentionally choose individuals who possess certain characteristics or experiences.
Impact: Purposive sampling can lead to bias because it focuses on specific attributes or characteristics of interest. If the chosen criteria do not represent the entire population, the sample may not accurately reflect the broader population, leading to skewed results.
Probability Sampling Bias
Probability sampling methods aim for random selection but can still be prone to bias. Here are the key types of probability sampling bias:
Simple Random Sampling Bias
Simple random sampling involves selecting individuals or elements from the population using a random process, such as drawing lots or using random number generators.
Impact: Even with random selection, bias can occur if the randomization process is flawed or not properly implemented. For example, if the randomization process is biased due to a technical error, certain groups may be overrepresented or underrepresented in the sample.
Systematic Sampling Bias
Systematic sampling selects individuals or elements at regular intervals from a population list. For example, every 10th person on a list might be chosen for the sample.
Impact: Systematic sampling can introduce bias if there is an underlying pattern in the population list. If the list is ordered in a way that correlates with the variable of interest, selecting at regular intervals may not provide a representative sample.
Stratified Sampling Bias
Stratified sampling divides the population into subgroups or strata based on specific characteristics, such as age, gender, or location. Researchers then sample proportionately from each stratum.
Impact: Stratified sampling is designed to ensure the representation of different subgroups, but bias can still occur if the criteria for stratification are chosen poorly. If the strata do not accurately reflect the population's diversity, the sample may not be truly representative.
Cluster Sampling Bias
Cluster sampling divides the population into clusters or groups, randomly selects a few clusters, and then samples all individuals within the selected clusters.
Impact: Cluster sampling can introduce bias if the clusters are not truly representative of the population. If the chosen clusters are not a random or accurate reflection of the population's characteristics, the sample may not be representative, leading to biased results.
Understanding these various types of sampling bias is essential for researchers and analysts to recognize potential sources of bias in their work and take steps to mitigate them effectively.
What Causes Sampling Bias?
Now that we've covered the types of sampling bias, let's explore what causes these biases to occur. Recognizing the underlying factors can help you mitigate bias effectively.
Selection Bias
Selection bias occurs when certain groups within the population have a higher probability of being included in the sample due to factors beyond randomness. This can happen for various reasons:
- Volunteer Bias: When participants volunteer to be part of a study, they may differ systematically from non-volunteers.
- Survivorship Bias: In cases where only survivors or successes are studied, this can lead to a skewed understanding of the population.
- Sampling Frame Issues: Problems with the list or database used for sampling can introduce bias.
Measurement Bias
Measurement bias arises from errors or inaccuracies in data collection or measurement techniques. Common sources include:
- Instrument Bias: The tools or instruments used to collect data may be flawed or incorrectly calibrated.
- Observer Bias: When researchers' personal beliefs or expectations influence their observations, this can lead to bias.
- Response Bias: Participants may provide inaccurate information due to social desirability or other factors.
Non-Response Bias
Non-response bias occurs when some individuals in the sample do not participate or provide data, and their absence introduces bias. This can happen for various reasons:
- Non-Contact: Certain groups may be underrepresented in the sample if they are more challenging to reach.
- Refusal to Participate: When individuals refuse to participate, their characteristics may differ from those who agree.
Time Interval Bias
Time interval bias is related to the timing of data collection. It can lead to inaccuracies when:
- Seasonal Variations: Data collected at a specific time of year may not accurately reflect the population's characteristics.
- Historical Trends: Trends and conditions can change over time, affecting the representativeness of data collected at different points.
Publication Bias
Publication bias occurs when studies with significant or positive results are more likely to be published than those with negative or null findings. This can create a distorted view of the available research.
Understanding these causes can help you identify potential sources of bias in your study and take steps to mitigate them effectively.
What is the Impact of Sampling Bias?
Sampling bias can have far-reaching consequences, affecting not only the reliability of your research but also its practical implications. Let's delve deeper into the specific impacts of sampling bias:
Distorted Results
When sampling bias creeps into your study, it can distort the results in several ways:
- Overestimation or Underestimation: Bias can cause you to overstate or understate the prevalence or effect of a phenomenon. For example, if you're studying public opinion and your sample predominantly consists of one demographic group, you may overestimate the support for a particular policy.
- False Associations: Bias can create inaccurate relationships between variables that don't actually exist. This can lead to incorrect assumptions about causality. For instance, if you're researching the effects of a specific dietary habit on health, but your sample is primarily composed of health-conscious individuals, you might falsely conclude that the dietary pattern has a more significant impact than it does.
- Misleading Trends: Biased results can lead to identifying trends or patterns that do not reflect the broader population. Making decisions or predictions based on these trends can lead to ineffective strategies and wasted resources.
Inaccurate Conclusions
The impact of sampling bias extends beyond distorted results and can directly affect the conclusions drawn from your research:
- Policy and Decision-Making Errors: Decision-makers often rely on research findings to formulate policies or make critical decisions. When these findings are based on biased data, it can lead to poor choices and ineffective policies.
- Wasted Resources: Resources invested in addressing a problem based on biased findings may be misallocated. This not only leads to inefficient resource utilization but can also exacerbate the issue you intended to solve.
- Misguided Interventions: In fields like healthcare or social services, biased conclusions can lead to interventions that don't achieve their intended outcomes. For example, if a medical study on a specific drug primarily includes participants who tolerate the drug well, it may lead to ineffective treatment plans.
Reduced External Validity
External validity, the ability to generalize research findings to a broader population, is often compromised by sampling bias:
- Limited Applicability: Research findings based on biased samples may not apply to the larger population you intend to study. This can hinder the generalizability of your results and limit their real-world relevance.
- Ineffective Policies: Policies or interventions developed based on research with limited external validity may not work as expected when applied to a broader context. This can lead to wasted efforts and unintended consequences.
Ethical Considerations
Ethical concerns come into play when certain groups are systematically excluded or misrepresented in research:
- Fairness and Equity: Sampling bias can perpetuate inequalities by neglecting underrepresented groups. This raises ethical questions about fairness and equity in research.
- Informed Consent: Ethical research requires informed consent from participants. If participants are not selected fairly or if they are unaware of potential biases, the ethical foundation of the study can be compromised.
- Social Impact: Biased research can have a social impact by reinforcing stereotypes or discriminatory practices. It's essential to consider the ethical implications of research on vulnerable or marginalized groups.
Understanding the potential consequences of sampling bias is critical for researchers, policymakers, and anyone who relies on research findings to make informed decisions. By recognizing these impacts, you can appreciate the importance of mitigating bias in your research.
How to Detect Sampling Bias?
Detecting sampling bias is a crucial step in ensuring the credibility of your research or analysis. Let's explore various methods and techniques to identify bias in your data:
Data Analysis Techniques
Effective data analysis can reveal patterns or inconsistencies that suggest the presence of bias:
- Compare to Known Demographics: Compare the demographic characteristics of your sample to the known demographics of the population you're studying. Significant discrepancies may indicate bias.
- Statistical Tests: Utilize statistical tests to assess the likelihood of bias in your sample. For example, you can perform chi-squared tests or t-tests to identify differences between your sample and the population.
- Data Triangulation: Cross-reference data from multiple sources or methods to identify inconsistencies. If data from different sources produce conflicting results, it may signal bias.
- Hypothesis Testing: Evaluate whether your findings are consistent with existing theories or prior research. If your results significantly deviate without a valid explanation, bias could be the cause.
Visualization Methods
Data visualization can be a powerful tool for detecting bias, as it can make patterns and irregularities more apparent:
- Histograms and Distributions: Create histograms to visualize the distribution of key variables in your sample. Anomalies or uneven distributions may indicate bias.
- Heatmaps: Use heatmaps to identify correlations or patterns among variables. Unexpected or skewed relationships may signal bias.
- Geospatial Analysis: If your data includes geographic information, conduct geospatial analysis to explore regional patterns. Disproportionate concentrations in specific areas may suggest bias.
- Comparative Visualization: Compare your sample's characteristics visually to those of the population. Side-by-side visualizations can highlight discrepancies.
Peer Review and Validation
Engaging peers or experts in your field for a review of your methodology and findings can provide valuable insights into potential bias:
- External Review: Seek input from independent experts who can scrutinize your research methods and data analysis for potential sources of bias.
- Validation Studies: Conduct additional studies or surveys to validate your findings and assess their generalizability. Compare the results to ensure consistency.
- Blind Review: Consider blind reviews where reviewers cannot access your sample demographics. This can reduce the potential for reviewer bias.
Detecting bias through data analysis, visualization, and external review can help you identify and address potential sources of bias early in your research process. By taking these steps, you can enhance the credibility and reliability of your findings.
How to Reduce Sampling Bias?
Minimizing sampling bias is essential to ensure the integrity of your research or data analysis. Let's explore strategies and practices that can help reduce the risk of bias in your work:
Randomization Techniques
Randomization is a powerful tool to ensure that your sample accurately represents the larger population:
- Simple Random Sampling: Implement simple random sampling to ensure that every individual or element in your population has an equal chance of being selected. This approach minimizes the risk of cherry-picking or favoring specific groups unintentionally.
- Random Assignment: In experimental research, use random assignment to allocate participants to different treatment groups. This method helps ensure that each group is comparable, reducing the risk of systematic bias.
- Random Start Points: When selecting data points or participants, use random start points to minimize the potential for bias. For example, if you're conducting telephone surveys, start calling at an arbitrary point in the phonebook.
Proper Sampling Methods
Selecting the suitable sampling method is crucial to minimize bias in your research:
- Stratified Sampling: Employ stratified sampling when you want to ensure the representation of specific subgroups within your population. This method divides the population into strata based on relevant characteristics, such as age or income, and then samples proportionately from each stratum.
- Cluster Sampling: In cases where accessing every element in your population is challenging, cluster sampling can be adequate. You select clusters (groups of elements) at random and then sample all elements within the selected clusters.
- Probability Sampling: Whenever possible, opt for probability sampling methods that involve random selection. These methods are inherently less prone to bias than non-probability sampling techniques.
Careful Study Design
A well-designed study can mitigate bias from the outset:
- Clear Inclusion Criteria: Define clear and objective inclusion criteria for selecting participants or data points. These criteria should be based on the research objectives and relevant population characteristics.
- Avoiding Leading Questions: In surveys and interviews, be cautious not to ask leading questions that could influence respondents' answers. Neutral and unbiased wording is essential to prevent response bias.
- Randomization Protocols: Implement rigorous randomization protocols in your study design. Ensure that the randomization process is well-documented and consistently applied.
- Blinding: In experimental research, consider using blinding techniques to prevent both participants and researchers from knowing which group they belong to. This can minimize experimenter bias and participant bias.
Pre-testing and Pilot Studies
Before launching your main study, consider conducting pre-testing and pilot studies:
- Identify Issues Early: Pre-testing allows you to identify potential sources of bias or data collection problems early in the research process. This is particularly important for survey instruments or measurement tools.
- Adjust as Needed: Based on the findings from pre-testing or pilot studies, be willing to modify your research design, questionnaire, or data collection procedures to address identified issues. This iterative approach can help fine-tune your research and reduce bias.
- Sample Size Determination: Pilot studies can also assist in estimating the required sample size for your main study accurately. This ensures that your sample size is sufficient for meaningful analysis without introducing unnecessary bias.
To ensure the success of your research endeavors, use the Appinio sample size calculator. By accurately determining the minimum sample size needed based on your desired margin of error, confidence level, and standard deviation, you can ensure that your survey results are truly representative of the population.
In addition, you can enhance the efficiency of your pre-testing and pilot studies with Appinio's advanced research tools. By leveraging our platform, you can streamline the process of identifying potential biases and data collection issues early on. With Appinio, you have the flexibility to adjust your research design and questionnaire based on real-time insights, ensuring the success of your main study.
Ready to optimize your research process and achieve more accurate results? Schedule a demo today and experience the power of Appinio firsthand!
Sampling Bias Examples and Case Studies
To gain a deeper understanding of the impact of sampling bias, let's explore some real-world examples where bias played a significant role in research outcomes. These case studies will not only shed light on the consequences of bias but also provide valuable lessons for researchers and decision-makers.
Real-World Sampling Bias Examples
1. Asbestos and Occupational Health
Background: In the mid-20th century, the health effects of asbestos exposure became a significant concern. Researchers conducted studies to assess the risk to workers, particularly those in asbestos mining and manufacturing industries.
Sampling Bias: Many early studies on asbestos exposure suffered from significant sampling bias. They often focused on healthy workers who were still employed, excluding individuals who had already become ill or died due to asbestos-related diseases. As a result, these studies underestimated the actual health risks associated with asbestos exposure.
Consequences: The underestimated risks delayed the recognition of asbestos-related diseases, such as mesothelioma and asbestosis, and hindered necessary regulatory actions to protect workers. It took decades to establish the true extent of the health hazards associated with asbestos.
2. Political Polling and Election Predictions
Background: Political polling and election predictions rely heavily on sampling techniques to gauge public opinion and forecast election outcomes.
Sampling Bias: In the 1936 U.S. presidential election, the Literary Digest conducted a poll using telephone directories and car registration lists. This method led to a significant sampling bias, as it primarily reached affluent individuals who could afford telephones and cars. The poll predicted a landslide victory for one candidate.
Consequences: The Literary Digest's prediction was wildly inaccurate, as it failed to capture the sentiments of a broad cross-section of the population. Franklin D. Roosevelt won by a wide margin, leading to a loss of credibility for the Literary Digest and a shift towards more representative sampling methods in political polling.
Lessons Learned
1. Representativeness is Key
These case studies underscore the critical importance of ensuring that your sample is representative of the larger population you intend to study. In the asbestos example, failing to include affected individuals led to a significant underestimation of health risks. Researchers and data analysts must make every effort to include diverse and relevant participants or data points.
2. Evolving Methods
The history of political polling demonstrates that methods can become outdated and biased over time. Researchers and pollsters must continually adapt their techniques to account for technological changes, communication, and demographics. Sticking to traditional methods without considering evolving factors can lead to substantial bias.
3. Ethical Considerations
The asbestos case reminds us of the ethical responsibility of researchers. In cases where people's health and well-being are at stake, it is crucial to prioritize ethical research practices. This includes transparency about potential biases, especially when the research has real-world consequences.
4. Public Trust
The inaccurate election prediction by the Literary Digest shows that public trust in research and polling can be fragile. Misleading results due to sampling bias can erode confidence in the credibility of research organizations. Maintaining trust requires rigorous adherence to unbiased sampling and reporting practices.
5. Validation and Peer Review
In both examples, the validation of results and peer review played a critical role in revealing bias and ensuring the accuracy of findings. Researchers should welcome critical review and verification of their work by peers to identify and address potential biases.
These case studies highlight the profound impact of sampling bias on research outcomes and the importance of continuous improvement in research methods. By learning from these examples and applying the lessons, researchers can enhance the quality and reliability of their work while minimizing the impact of bias on decision-making and policy development.
Conclusion for Sampling Bias
Understanding and addressing sampling bias is essential for anyone involved in research and data analysis. It's like having a compass in the vast sea of data, helping you navigate towards accurate results and informed decisions. By recognizing the types and causes of bias, being vigilant in detection, and implementing strategies to minimize it, you can ensure your findings are trustworthy, and your contributions to knowledge and decision-making are as robust as they can be.
Remember, the journey to conquering sampling bias may not always be straightforward, but the rewards in terms of reliable data and insights are well worth the effort. So, as you embark on your research endeavors, keep these lessons close, stay mindful of bias, and chart a course toward data-driven success.
How to Avoid Sampling Bias?
Discover the future of market research with Appinio, the real-time market research platform that puts the power of unbiased insights at your fingertips. Say goodbye to sampling bias and hello to quick, data-driven decisions.
Here's why you'll love using Appinio:
- Instant Insights: From your questions to valuable insights in minutes, no more waiting around for research results.
- User-Friendly: You don't need a PhD in research to use our intuitive platform; it's designed for anyone to navigate effortlessly.
- Global Reach: Define your target audience from over 1,200 characteristics and survey them in 90+ countries, ensuring your research reaches the right people, anywhere in the world.
Get facts and figures ðŸ§
Want to see more data insights? Our free reports are just the right thing for you!