Experimental Research: Definition, Types, Design, Examples
Appinio Research · 14.05.2024 · 32min read
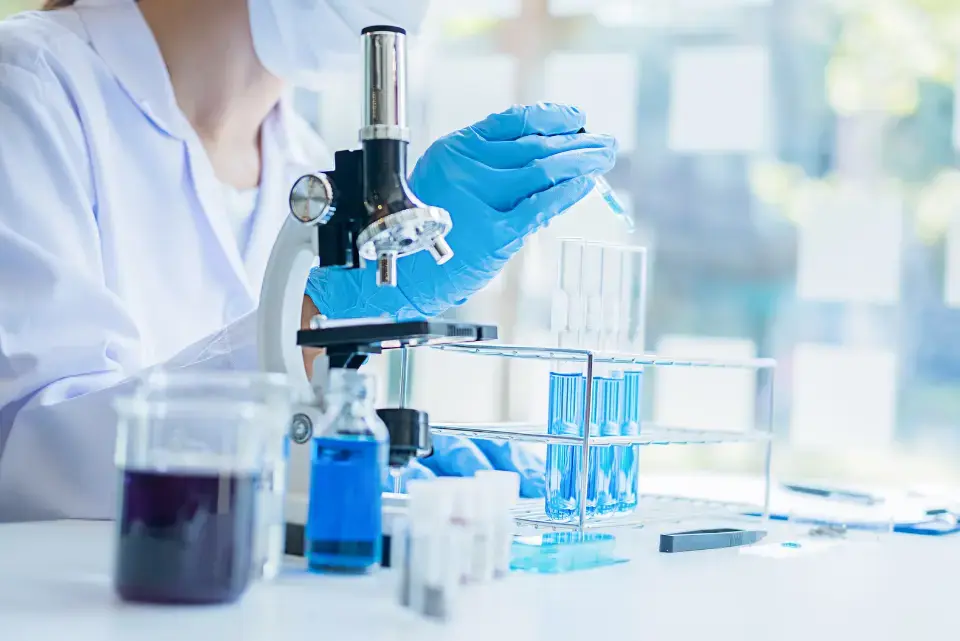
Content
Experimental research is a cornerstone of scientific inquiry, providing a systematic approach to understanding cause-and-effect relationships and advancing knowledge in various fields. At its core, experimental research involves manipulating variables, observing outcomes, and drawing conclusions based on empirical evidence. By controlling factors that could influence the outcome, researchers can isolate the effects of specific variables and make reliable inferences about their impact.
This guide offers a step-by-step exploration of experimental research, covering key elements such as research design, data collection, analysis, and ethical considerations. Whether you're a novice researcher seeking to understand the basics or an experienced scientist looking to refine your experimental techniques, this guide will equip you with the knowledge and tools needed to conduct rigorous and insightful research.
What is Experimental Research?
Experimental research is a systematic approach to scientific inquiry that aims to investigate cause-and-effect relationships by manipulating independent variables and observing their effects on dependent variables. Experimental research primarily aims to test hypotheses, make predictions, and draw conclusions based on empirical evidence.
By controlling extraneous variables and randomizing participant assignment, researchers can isolate the effects of specific variables and establish causal relationships. Experimental research is characterized by its rigorous methodology, emphasis on objectivity, and reliance on empirical data to support conclusions.
Importance of Experimental Research
- Establishing Cause-and-Effect Relationships: Experimental research allows researchers to establish causal relationships between variables by systematically manipulating independent variables and observing their effects on dependent variables. This provides valuable insights into the underlying mechanisms driving phenomena and informs theory development.
- Testing Hypotheses and Making Predictions: Experimental research provides a structured framework for testing hypotheses and predicting the relationship between variables. By systematically manipulating variables and controlling for confounding factors, researchers can empirically test the validity of their hypotheses and refine theoretical models.
- Informing Evidence-Based Practice: Experimental research generates empirical evidence that informs evidence-based practice in various fields, including healthcare, education, and business. Experimental research contributes to improving outcomes and informing decision-making in real-world settings by identifying effective interventions, treatments, and strategies.
- Driving Innovation and Advancement: Experimental research drives innovation and advancement by uncovering new insights, challenging existing assumptions, and pushing the boundaries of knowledge. Through rigorous experimentation and empirical validation, researchers can develop novel solutions to complex problems and contribute to the advancement of science and technology.
- Enhancing Research Rigor and Validity: Experimental research upholds high research rigor and validity standards by employing systematic methods, controlling for confounding variables, and ensuring replicability of findings. By adhering to rigorous methodology and ethical principles, experimental research produces reliable and credible evidence that withstands scrutiny and contributes to the cumulative body of knowledge.
Experimental research plays a pivotal role in advancing scientific understanding, informing evidence-based practice, and driving innovation across various disciplines. By systematically testing hypotheses, establishing causal relationships, and generating empirical evidence, experimental research contributes to the collective pursuit of knowledge and the improvement of society.
Understanding Experimental Design
Experimental design serves as the blueprint for your study, outlining how you'll manipulate variables and control factors to draw valid conclusions.
Experimental Design Components
Experimental design comprises several essential elements:
- Independent Variable (IV): This is the variable manipulated by the researcher. It's what you change to observe its effect on the dependent variable. For example, in a study testing the impact of different study techniques on exam scores, the independent variable might be the study method (e.g., flashcards, reading, or practice quizzes).
- Dependent Variable (DV): The dependent variable is what you measure to assess the effect of the independent variable. It's the outcome variable affected by the manipulation of the independent variable. In our study example, the dependent variable would be the exam scores.
- Control Variables: These factors could influence the outcome but are kept constant or controlled to isolate the effect of the independent variable. Controlling variables helps ensure that any observed changes in the dependent variable can be attributed to manipulating the independent variable rather than other factors.
- Experimental Group: This group receives the treatment or intervention being tested. It's exposed to the manipulated independent variable. In contrast, the control group does not receive the treatment and serves as a baseline for comparison.
Types of Experimental Designs
Experimental designs can vary based on the research question, the nature of the variables, and the desired level of control. Here are some common types:
- Between-Subjects Design: In this design, different groups of participants are exposed to varying levels of the independent variable. Each group represents a different experimental condition, and participants are only exposed to one condition. For instance, in a study comparing the effectiveness of two teaching methods, one group of students would use Method A, while another would use Method B.
- Within-Subjects Design: Also known as repeated measures design, this approach involves exposing the same group of participants to all levels of the independent variable. Participants serve as their own controls, and the order of conditions is typically counterbalanced to control for order effects. For example, participants might be tested on their reaction times under different lighting conditions, with the order of conditions randomized to eliminate any research bias.
- Mixed Designs: Mixed designs combine elements of both between-subjects and within-subjects designs. This allows researchers to examine both between-group differences and within-group changes over time. Mixed designs help study complex phenomena that involve multiple variables and temporal dynamics.
Factors Influencing Experimental Design Choices
Several factors influence the selection of an appropriate experimental design:
- Research Question: The nature of your research question will guide your choice of experimental design. Some questions may be better suited to between-subjects designs, while others may require a within-subjects approach.
- Variables: Consider the number and type of variables involved in your study. A factorial design might be appropriate if you're interested in exploring multiple factors simultaneously. Conversely, if you're focused on investigating the effects of a single variable, a simpler design may suffice.
- Practical Considerations: Practical constraints such as time, resources, and access to participants can impact your choice of experimental design. Depending on your study's specific requirements, some designs may be more feasible or cost-effective than others.
- Ethical Considerations: Ethical concerns, such as the potential risks to participants or the need to minimize harm, should also inform your experimental design choices. Ensure that your design adheres to ethical guidelines and safeguards the rights and well-being of participants.
By carefully considering these factors and selecting an appropriate experimental design, you can ensure that your study is well-designed and capable of yielding meaningful insights.
Experimental Research Elements
When conducting experimental research, understanding the key elements is crucial for designing and executing a robust study. Let's explore each of these elements in detail to ensure your experiment is well-planned and executed effectively.
Independent and Dependent Variables
In experimental research, the independent variable (IV) is the factor that the researcher manipulates or controls, while the dependent variable (DV) is the measured outcome or response. The independent variable is what you change in the experiment to observe its effect on the dependent variable.
For example, in a study investigating the effect of different fertilizers on plant growth, the type of fertilizer used would be the independent variable, while the plant growth (height, number of leaves, etc.) would be the dependent variable.
Control Groups and Experimental Groups
Control groups and experimental groups are essential components of experimental design. The control group serves as a baseline for comparison and does not receive the treatment or intervention being studied. Its purpose is to provide a reference point to assess the effects of the independent variable.
In contrast, the experimental group receives the treatment or intervention and is used to measure the impact of the independent variable. For example, in a drug trial, the control group would receive a placebo, while the experimental group would receive the actual medication.
Randomization and Random Sampling
Randomization is the process of randomly assigning participants to different experimental conditions to minimize biases and ensure that each participant has an equal chance of being assigned to any condition. Randomization helps control for extraneous variables and increases the study's internal validity.
Random sampling, on the other hand, involves selecting a representative sample from the population of interest to generalize the findings to the broader population. Random sampling ensures that each member of the population has an equal chance of being included in the sample, reducing the risk of sampling bias.
Replication and Reliability
Replication involves repeating the experiment to confirm the results and assess the reliability of the findings. It is essential for ensuring the validity of scientific findings and building confidence in the robustness of the results. A study that can be replicated consistently across different settings and by various researchers is considered more reliable. Researchers should strive to design experiments that are easily replicable and transparently report their methods to facilitate replication by others.
Validity: Internal, External, Construct, and Statistical Conclusion Validity
Validity refers to the degree to which an experiment measures what it intends to measure and the extent to which the results can be generalized to other populations or contexts. There are several types of validity that researchers should consider:
- Internal Validity: Internal validity refers to the extent to which the study accurately assesses the causal relationship between variables. Internal validity is threatened by factors such as confounding variables, selection bias, and experimenter effects. Researchers can enhance internal validity through careful experimental design and control procedures.
- External Validity: External validity refers to the extent to which the study's findings can be generalized to other populations or settings. External validity is influenced by factors such as the representativeness of the sample and the ecological validity of the experimental conditions. Researchers should consider the relevance and applicability of their findings to real-world situations.
- Construct Validity: Construct validity refers to the degree to which the study accurately measures the theoretical constructs of interest. Construct validity is concerned with whether the operational definitions of the variables align with the underlying theoretical concepts. Researchers can establish construct validity through careful measurement selection and validation procedures.
- Statistical Conclusion Validity: Statistical conclusion validity refers to the accuracy of the statistical analyses and conclusions drawn from the data. It ensures that the statistical tests used are appropriate for the data and that the conclusions drawn are warranted. Researchers should use robust statistical methods and report effect sizes and confidence intervals to enhance statistical conclusion validity.
By addressing these elements of experimental research and ensuring the validity and reliability of your study, you can conduct research that contributes meaningfully to the advancement of knowledge in your field.
How to Conduct Experimental Research?
Embarking on an experimental research journey involves a series of well-defined phases, each crucial for the success of your study. Let's explore the pre-experimental, experimental, and post-experimental phases to ensure you're equipped to conduct rigorous and insightful research.
Pre-Experimental Phase
The pre-experimental phase lays the foundation for your study, setting the stage for what's to come. Here's what you need to do:
- Formulating Research Questions and Hypotheses: Start by clearly defining your research questions and formulating testable hypotheses. Your research questions should be specific, relevant, and aligned with your research objectives. Hypotheses provide a framework for testing the relationships between variables and making predictions about the outcomes of your study.
- Reviewing Literature and Establishing Theoretical Framework: Dive into existing literature relevant to your research topic and establish a solid theoretical framework. Literature review helps you understand the current state of knowledge, identify research gaps, and build upon existing theories. A well-defined theoretical framework provides a conceptual basis for your study and guides your research design and analysis.
Experimental Phase
The experimental phase is where the magic happens – it's time to put your hypotheses to the test and gather data. Here's what you need to consider:
- Participant Recruitment and Sampling Techniques: Carefully recruit participants for your study using appropriate sampling techniques. The sample should be representative of the population you're studying to ensure the generalizability of your findings. Consider factors such as sample size, demographics, and inclusion criteria when recruiting participants.
- Implementing Experimental Procedures: Once you've recruited participants, it's time to implement your experimental procedures. Clearly outline the experimental protocol, including instructions for participants, procedures for administering treatments or interventions, and measures for controlling extraneous variables. Standardize your procedures to ensure consistency across participants and minimize sources of bias.
- Data Collection and Measurement: Collect data using reliable and valid measurement instruments. Depending on your research questions and variables of interest, data collection methods may include surveys, observations, physiological measurements, or experimental tasks. Ensure that your data collection procedures are ethical, respectful of participants' rights, and designed to minimize errors and biases.
Post-Experimental Phase
In the post-experimental phase, you make sense of your data, draw conclusions, and communicate your findings to the world. Here's what you need to do:
- Data Analysis Techniques: Analyze your data using appropriate statistical techniques. Choose methods that are aligned with your research design and hypotheses. Standard statistical analyses include descriptive statistics, inferential statistics (e.g., t-tests, ANOVA), regression analysis, and correlation analysis. Interpret your findings in the context of your research questions and theoretical framework.
- Interpreting Results and Drawing Conclusions: Once you've analyzed your data, interpret the results and draw conclusions. Discuss the implications of your findings, including any theoretical, practical, or real-world implications. Consider alternative explanations and limitations of your study and propose avenues for future research. Be transparent about the strengths and weaknesses of your study to enhance the credibility of your conclusions.
- Reporting Findings: Finally, communicate your findings through research reports, academic papers, or presentations. Follow standard formatting guidelines and adhere to ethical standards for research reporting. Clearly articulate your research objectives, methods, results, and conclusions. Consider your target audience and choose appropriate channels for disseminating your findings to maximize impact and reach.
By meticulously planning and executing each experimental research phase, you can generate valuable insights, advance knowledge in your field, and contribute to scientific progress.
As you navigate the intricate phases of experimental research, leveraging Appinio can streamline your journey toward actionable insights. With our intuitive platform, you can swiftly gather real-time consumer data, empowering you to make informed decisions with confidence. Say goodbye to the complexities of traditional market research and hello to a seamless, efficient process that puts you in the driver's seat of your research endeavors.
Ready to revolutionize your approach to data-driven decision-making? Book a demo today and discover the power of Appinio in transforming your research experience!
Experimental Research Examples
Understanding how experimental research is applied in various contexts can provide valuable insights into its practical significance and effectiveness. Here are some examples illustrating the application of experimental research in different domains:
Market Research
Experimental studies are crucial in market research in testing hypotheses, evaluating marketing strategies, and understanding consumer behavior. For example, a company may conduct an experiment to determine the most effective advertising message for a new product. Participants could be exposed to different versions of an advertisement, each emphasizing different product features or appeals.
By measuring variables such as brand recall, purchase intent, and brand perception, researchers can assess the impact of each advertising message and identify the most persuasive approach.
Software as a Service (SaaS)
In the SaaS industry, experimental research is often used to optimize user interfaces, features, and pricing models to enhance user experience and drive engagement. For instance, a SaaS company may conduct A/B tests to compare two versions of its software interface, each with a different layout or navigation structure.
Researchers can identify design elements that lead to higher user satisfaction and retention by tracking user interactions, conversion rates, and customer feedback. Experimental research also enables SaaS companies to test new product features or pricing strategies before full-scale implementation, minimizing risks and maximizing return on investment.
Business Management
Experimental research is increasingly utilized in business management to inform decision-making, improve organizational processes, and drive innovation. For example, a business may conduct an experiment to evaluate the effectiveness of a new training program on employee productivity. Participants could be randomly assigned to either receive the training or serve as a control group.
By measuring performance metrics such as sales revenue, customer satisfaction, and employee turnover, researchers can assess the training program's impact and determine its return on investment. Experimental research in business management provides empirical evidence to support strategic initiatives and optimize resource allocation.
Healthcare
In healthcare, experimental research is instrumental in testing new treatments, interventions, and healthcare delivery models to improve patient outcomes and quality of care. For instance, a clinical trial may be conducted to evaluate the efficacy of a new drug in treating a specific medical condition. Participants are randomly assigned to either receive the experimental drug or a placebo, and their health outcomes are monitored over time.
By comparing the effectiveness of the treatment and placebo groups, researchers can determine the drug's efficacy, safety profile, and potential side effects. Experimental research in healthcare informs evidence-based practice and drives advancements in medical science and patient care.
These examples illustrate the versatility and applicability of experimental research across diverse domains, demonstrating its value in generating actionable insights, informing decision-making, and driving innovation. Whether in market research or healthcare, experimental research provides a rigorous and systematic approach to testing hypotheses, evaluating interventions, and advancing knowledge.
Experimental Research Challenges
Even with careful planning and execution, experimental research can present various challenges. Understanding these challenges and implementing effective solutions is crucial for ensuring the validity and reliability of your study. Here are some common challenges and strategies for addressing them.
Sample Size and Statistical Power
Challenge: Inadequate sample size can limit your study's generalizability and statistical power, making it difficult to detect meaningful effects. Small sample sizes increase the risk of Type II errors (false negatives) and reduce the reliability of your findings.
Solution: Increase your sample size to improve statistical power and enhance the robustness of your results. Conduct a power analysis before starting your study to determine the minimum sample size required to detect the effects of interest with sufficient power. Consider factors such as effect size, alpha level, and desired power when calculating sample size requirements. Additionally, consider using techniques such as bootstrapping or resampling to augment small sample sizes and improve the stability of your estimates.
To enhance the reliability of your experimental research findings, you can leverage our Sample Size Calculator. By determining the optimal sample size based on your desired margin of error, confidence level, and standard deviation, you can ensure the representativeness of your survey results. Don't let inadequate sample sizes hinder the validity of your study and unlock the power of precise research planning!
Confounding Variables and Bias
Challenge: Confounding variables are extraneous factors that co-vary with the independent variable and can distort the relationship between the independent and dependent variables. Confounding variables threaten the internal validity of your study and can lead to erroneous conclusions.
Solution: Implement control measures to minimize the influence of confounding variables on your results. Random assignment of participants to experimental conditions helps distribute confounding variables evenly across groups, reducing their impact on the dependent variable. Additionally, consider using matching or blocking techniques to ensure that groups are comparable on relevant variables. Conduct sensitivity analyses to assess the robustness of your findings to potential confounders and explore alternative explanations for your results.
Researcher Effects and Experimenter Bias
Challenge: Researcher effects and experimenter bias occur when the experimenter's expectations or actions inadvertently influence the study's outcomes. This bias can manifest through subtle cues, unintentional behaviors, or unconscious biases, leading to invalid conclusions.
Solution: Implement double-blind procedures whenever possible to mitigate researcher effects and experimenter bias. Double-blind designs conceal information about the experimental conditions from both the participants and the experimenters, minimizing the potential for bias. Standardize experimental procedures and instructions to ensure consistency across conditions and minimize experimenter variability. Additionally, consider using objective outcome measures or automated data collection procedures to reduce the influence of experimenter bias on subjective assessments.
External Validity and Generalizability
Challenge: External validity refers to the extent to which your study's findings can be generalized to other populations, settings, or conditions. Limited external validity restricts the applicability of your results and may hinder their relevance to real-world contexts.
Solution: Enhance external validity by designing studies closely resembling real-world conditions and populations of interest. Consider using diverse samples that represent the target population's demographic, cultural, and ecological variability. Conduct replication studies in different contexts or with different populations to assess the robustness and generalizability of your findings. Additionally, consider conducting meta-analyses or systematic reviews to synthesize evidence from multiple studies and enhance the external validity of your conclusions.
By proactively addressing these challenges and implementing effective solutions, you can strengthen the validity, reliability, and impact of your experimental research. Remember to remain vigilant for potential pitfalls throughout the research process and adapt your strategies as needed to ensure the integrity of your findings.
Advanced Topics in Experimental Research
As you delve deeper into experimental research, you'll encounter advanced topics and methodologies that offer greater complexity and nuance.
Quasi-Experimental Designs
Quasi-experimental designs resemble true experiments but lack random assignment to experimental conditions. They are often used when random assignment is impractical, unethical, or impossible. Quasi-experimental designs allow researchers to investigate cause-and-effect relationships in real-world settings where strict experimental control is challenging. Common examples include:
- Non-Equivalent Groups Design: This design compares two or more groups that were not created through random assignment. While similar to between-subjects designs, non-equivalent group designs lack the random assignment of participants, increasing the risk of confounding variables.
- Interrupted Time Series Design: In this design, multiple measurements are taken over time before and after an intervention is introduced. Changes in the dependent variable are assessed over time, allowing researchers to infer the impact of the intervention.
- Regression Discontinuity Design: This design involves assigning participants to different groups based on a cutoff score on a continuous variable. Participants just above and below the cutoff are treated as if they were randomly assigned to different conditions, allowing researchers to estimate causal effects.
Quasi-experimental designs offer valuable insights into real-world phenomena but require careful consideration of potential confounding variables and limitations inherent to non-random assignment.
Factorial Designs
Factorial designs involve manipulating two or more independent variables simultaneously to examine their main effects and interactions. By systematically varying multiple factors, factorial designs allow researchers to explore complex relationships between variables and identify how they interact to influence outcomes. Common types of factorial designs include:
- 2x2 Factorial Design: This design manipulates two independent variables, each with two levels. It allows researchers to examine the main effects of each variable as well as any interaction between them.
- Mixed Factorial Design: In this design, one independent variable is manipulated between subjects, while another is manipulated within subjects. Mixed factorial designs enable researchers to investigate both between-subjects and within-subjects effects simultaneously.
Factorial designs provide a comprehensive understanding of how multiple factors contribute to outcomes and offer greater statistical efficiency compared to studying variables in isolation.
Longitudinal and Cross-Sectional Studies
Longitudinal studies involve collecting data from the same participants over an extended period, allowing researchers to observe changes and trajectories over time. Cross-sectional studies, on the other hand, involve collecting data from different participants at a single point in time, providing a snapshot of the population at that moment. Both longitudinal and cross-sectional studies offer unique advantages and challenges:
- Longitudinal Studies: Longitudinal designs allow researchers to examine developmental processes, track changes over time, and identify causal relationships. However, longitudinal studies require long-term commitment, are susceptible to attrition and dropout, and may be subject to practice effects and cohort effects.
- Cross-Sectional Studies: Cross-sectional designs are relatively quick and cost-effective, provide a snapshot of population characteristics, and allow for comparisons across different groups. However, cross-sectional studies cannot assess changes over time or establish causal relationships between variables.
Researchers should carefully consider the research question, objectives, and constraints when choosing between longitudinal and cross-sectional designs.
Meta-Analysis and Systematic Reviews
Meta-analysis and systematic reviews are quantitative methods used to synthesize findings from multiple studies and draw robust conclusions. These methods offer several advantages:
- Meta-Analysis: Meta-analysis combines the results of multiple studies using statistical techniques to estimate overall effect sizes and assess the consistency of findings across studies. Meta-analysis increases statistical power, enhances generalizability, and provides more precise estimates of effect sizes.
- Systematic Reviews: Systematic reviews involve systematically searching, appraising, and synthesizing existing literature on a specific topic. Systematic reviews provide a comprehensive summary of the evidence, identify gaps and inconsistencies in the literature, and inform future research directions.
Meta-analysis and systematic reviews are valuable tools for evidence-based practice, guiding policy decisions, and advancing scientific knowledge by aggregating and synthesizing empirical evidence from diverse sources.
By exploring these advanced topics in experimental research, you can expand your methodological toolkit, tackle more complex research questions, and contribute to deeper insights and understanding in your field.
Experimental Research Ethical Considerations
When conducting experimental research, it's imperative to uphold ethical standards and prioritize the well-being and rights of participants. Here are some key ethical considerations to keep in mind throughout the research process:
- Informed Consent: Obtain informed consent from participants before they participate in your study. Ensure that participants understand the purpose of the study, the procedures involved, any potential risks or benefits, and their right to withdraw from the study at any time without penalty.
- Protection of Participants' Rights: Respect participants' autonomy, privacy, and confidentiality throughout the research process. Safeguard sensitive information and ensure that participants' identities are protected. Be transparent about how their data will be used and stored.
- Minimizing Harm and Risks: Take steps to mitigate any potential physical or psychological harm to participants. Conduct a risk assessment before starting your study and implement appropriate measures to reduce risks. Provide support services and resources for participants who may experience distress or adverse effects as a result of their participation.
- Confidentiality and Data Security: Protect participants' privacy and ensure the security of their data. Use encryption and secure storage methods to prevent unauthorized access to sensitive information. Anonymize data whenever possible to minimize the risk of data breaches or privacy violations.
- Avoiding Deception: Minimize the use of deception in your research and ensure that any deception is justified by the scientific objectives of the study. If deception is necessary, debrief participants fully at the end of the study and provide them with an opportunity to withdraw their data if they wish.
- Respecting Diversity and Cultural Sensitivity: Be mindful of participants' diverse backgrounds, cultural norms, and values. Avoid imposing your own cultural biases on participants and ensure that your research is conducted in a culturally sensitive manner. Seek input from diverse stakeholders to ensure your research is inclusive and respectful.
- Compliance with Ethical Guidelines: Familiarize yourself with relevant ethical guidelines and regulations governing research with human participants, such as those outlined by institutional review boards (IRBs) or ethics committees. Ensure that your research adheres to these guidelines and that any potential ethical concerns are addressed appropriately.
- Transparency and Openness: Be transparent about your research methods, procedures, and findings. Clearly communicate the purpose of your study, any potential risks or limitations, and how participants' data will be used. Share your research findings openly and responsibly, contributing to the collective body of knowledge in your field.
By prioritizing ethical considerations in your experimental research, you demonstrate integrity, respect, and responsibility as a researcher, fostering trust and credibility in the scientific community.
Conclusion for Experimental Research
Experimental research is a powerful tool for uncovering causal relationships and expanding our understanding of the world around us. By carefully designing experiments, collecting data, and analyzing results, researchers can make meaningful contributions to their fields and address pressing questions. However, conducting experimental research comes with responsibilities. Ethical considerations are paramount to ensure the well-being and rights of participants, as well as the integrity of the research process. Researchers can build trust and credibility in their work by upholding ethical standards and prioritizing participant safety and autonomy.
Furthermore, as you continue to explore and innovate in experimental research, you must remain open to new ideas and methodologies. Embracing diversity in perspectives and approaches fosters creativity and innovation, leading to breakthrough discoveries and scientific advancements. By promoting collaboration and sharing findings openly, we can collectively push the boundaries of knowledge and tackle some of society's most pressing challenges.
How to Conduct Research in Minutes?
Discover the power of Appinio, the real-time market research platform revolutionizing experimental research. With Appinio, you can access real-time consumer insights to make better data-driven decisions in minutes. Join the thousands of companies worldwide who trust Appinio to deliver fast, reliable consumer insights.
Here's why you should consider using Appinio for your research needs:
- From questions to insights in minutes: With Appinio, you can conduct your own market research and get actionable insights in record time, allowing you to make fast, informed decisions for your business.
- Intuitive platform for anyone: You don't need a PhD in research to use Appinio. Our platform is designed to be user-friendly and intuitive so that anyone can easily create and launch surveys.
- Extensive reach and targeting options: Define your target audience from over 1200 characteristics and survey them in over 90 countries. Our platform ensures you reach the right people for your research needs, no matter where they are.
Join the loop 💌
Be the first to hear about new updates, product news, and data insights. We'll send it all straight to your inbox.