What is A/B Testing? Guide, Tools, Examples
Appinio Research · 01.12.2023 · 42min read
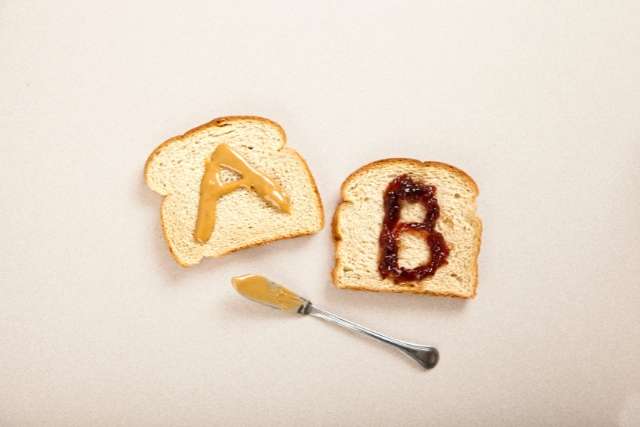
Content
Are you looking to transform your digital strategies into data-driven successes? In today's dynamic online landscape, achieving optimal user engagement, conversions, and performance is a constant challenge.
This guide will be your compass, guiding you through the intricate realm of data-driven decision-making. Dive into the world of A/B testing, where you'll discover the power to validate hypotheses, enhance user experiences, and ultimately drive success in the digital domain.
What is A/B Testing?
A/B testing, also known as split testing or bucket testing, is a method used to evaluate and compare two versions of a webpage, email campaign, app interface, or any digital asset. It involves presenting two variations, Version A (the control group) and Version B (the experimental group), to different groups of users simultaneously. By measuring user interactions and behavior, A/B testing helps businesses and organizations make data-driven decisions to optimize their digital assets for improved performance, user engagement, and conversions.
Key Aspects of A/B Testing
- Randomization: Users are randomly assigned to either Version A or Version B to eliminate bias and ensure a fair comparison.
- Statistical Significance: A/B tests use statistical analysis to determine whether differences between the variations are statistically significant or simply due to chance.
- Key Metrics: Tests are based on predefined key metrics or objectives, such as click-through rates, conversion rates, open rates, or revenue per visitor.
- Iterative Process: A/B testing is an iterative process, allowing businesses to continuously refine and enhance their digital assets over time.
Importance of A/B Testing
A/B testing plays a vital role in data-driven decision-making and optimization strategies for businesses and organizations. Here's why A/B testing is important:
- Evidence-Based Decision-Making: A/B testing provides concrete data and insights, allowing businesses to make decisions based on actual user behavior rather than assumptions or opinions.
- Optimizing User Experience: It helps in improving the user experience by identifying changes that resonate better with the audience, leading to increased engagement and satisfaction.
- Maximizing Conversions: A/B testing helps identify which elements or strategies lead to higher conversion rates, such as more purchases, sign-ups, or clicks, ultimately boosting revenue and ROI.
- Reducing Risk: By testing changes on a subset of users, organizations can assess potential risks and ensure that alterations do not negatively impact the overall user base.
- Continuous Improvement: A/B testing promotes a culture of continuous improvement, allowing businesses to refine their digital assets iteratively and stay ahead of competitors.
- Cost-Efficiency: It enables organizations to allocate resources effectively by investing in changes that are proven to yield positive results, avoiding costly missteps.
- Personalization: A/B testing can be used to personalize content and experiences for different user segments, enhancing relevance and engagement.
- Enhancing Marketing Campaigns: In marketing, A/B testing helps fine-tune email campaigns, ad copy, landing pages, and other assets to maximize effectiveness and ROI.
- Adapting to User Trends: Businesses can adapt to changing user preferences and trends by continuously testing and optimizing their digital presence.
- Validating Hypotheses: A/B testing allows organizations to validate hypotheses and ideas quickly, ensuring that changes are backed by empirical evidence.
A/B testing is a powerful tool that empowers businesses and organizations to make informed decisions, enhance user experiences, and achieve better outcomes in a data-driven and cost-effective manner. It is a fundamental practice in the world of digital optimization and marketing.
How to Plan an A/B Test?
Before you jump into A/B testing, it's essential to have a well-thought-out plan in place. Proper planning sets the foundation for a successful experiment and ensures you gain meaningful insights. Let's delve deeper into each aspect of planning your A/B test.
1. Define Clear Objectives
When defining clear objectives for your A/B test, think about what specific goals you want to achieve. Your objectives should be:
- Specific: Clearly state what you intend to accomplish. For example, instead of a vague objective like "improve website performance," you might specify, "increase the click-through rate on the product page by 15%."
- Measurable: Establish metrics that will allow you to measure success. In the example above, the measurable metric is the click-through rate.
- Aligned with Goals: Ensure that your objectives align with your overall business or project goals. If your primary goal is to boost revenue, focus on objectives that contribute to that goal.
- Time-Bound: Set a timeframe for achieving your objectives. This adds urgency and helps you determine when the test is considered complete.
- Realistic: Make sure your objectives are attainable within the scope of your test. Setting overly ambitious goals may lead to unrealistic expectations.
For instance, if you're running an e-commerce site, your A/B test objectives might include increasing the conversion rate, reducing cart abandonment, or improving product page engagement. Having clear objectives guides the entire testing process and ensures you have a specific target to aim for.
2. Identify Key Metrics
Identifying the right key metrics is crucial for measuring the success of your A/B test. The choice of metrics should align with your objectives and provide valuable insights into user behavior. Here are some common key metrics to consider:
- Conversion Rate: This is one of the most fundamental metrics. It measures the percentage of visitors who take the desired action, such as making a purchase or signing up.
- Click-Through Rate (CTR): CTR measures the effectiveness of links or calls-to-action. It's especially relevant for email campaigns and landing pages.
- Revenue per Visitor (RPV): If your goal is revenue-driven, RPV helps determine how much each visitor contributes to your income.
- Bounce Rate: Bounce rate indicates the percentage of visitors who leave your site after viewing only one page. Reducing the bounce rate often leads to improved user engagement.
- Session Duration: This metric tells you how long visitors spend on your site. Longer sessions often indicate more engaged users.
- Cart Abandonment Rate: For e-commerce sites, this metric measures the percentage of users who add products to their cart but don't complete the purchase.
The choice of key metrics will vary depending on your specific objectives. For instance, if your aim is to increase engagement, you might focus on metrics like session duration and page views. If you're aiming to boost revenue, then conversion rate and RPV become crucial.
3. Select the Test Variable
The test variable, also known as the "treatment" or "variant," is the element or elements you want to test. This can include any aspect of your digital content, such as:
- Headlines: Testing different headline variations to see which one grabs more attention.
- Images: Comparing the impact of various photos or graphics on user engagement.
- Call-to-Action (CTA) Buttons: Experimenting with different CTA button texts, colors, or placements.
- Page Layout: Testing different page layouts, navigation menus, or content organization.
- Product Descriptions: Evaluating the effectiveness of different product descriptions in driving conversions.
When selecting the test variable, it's essential to focus on one element at a time to isolate the impact of the change. Testing multiple variables simultaneously can lead to ambiguous results. Make sure the variable you choose directly relates to your objectives and metrics.
4. Determine Sample Size
Determining the right sample size is critical to the statistical validity of your A/B test. A sample size that's too small may produce unreliable results, while a sample size that's too large can be resource-intensive and time-consuming. Here's how to calculate an appropriate sample size:
- Statistical Significance Level: Decide on the level of statistical significance you want to achieve. Common levels are 95% and 99%, indicating the level of confidence you have in the results.
- Minimum Detectable Effect (MDE): Determine the smallest meaningful difference you want to detect. This is often based on your objectives. For instance, if you want to detect a 10% increase in conversion rate, the MDE is 10%.
- Baseline Conversion Rate: Know the current conversion rate of the element you're testing. This serves as your baseline.
- Variability: Understand the variation or uncertainty in your data.
Once you have these parameters, you can use online sample size calculators or statistical software to determine the sample size needed for your test. A larger sample size increases the likelihood of detecting small but meaningful changes, while a smaller sample size is more efficient but may miss subtle improvements.
Properly planning your A/B test sets the stage for a successful experiment. Clear objectives, well-chosen metrics, a thoughtfully selected test variable, and an appropriate sample size will ensure that your test is both informative and actionable.
How to Set Up an A/B Test?
Once you have a clear plan in place, it's time to set up your A/B test effectively. This phase involves creating the test variations, ensuring unbiased testing through randomization, and implementing robust tracking and analytics to collect valuable data.
1. Create Variations (A and B)
Creating variations A and B is the heart of A/B testing. Here's how to do it effectively:
- Identify the Element to Test: Refer back to the test variable you selected during the planning phase. This could be a headline, image, CTA button, page layout, or any other element.
- Design the Variations: Create two distinct versions of the element. Version A (the control group) should remain unchanged and serve as the baseline. Version B (the experimental group) should include the specific changes you want to test.
Example: If you're testing a CTA button's color, Version A might have a green button, while Version B has a red button. - Maintain Consistency: Ensure that any other elements on the page remain consistent between the two variations. This means keeping the same text, images, and layout, except for the element you're testing.
- Test One Variable at a Time: It's essential to isolate the impact of the change you're testing. If you make multiple changes in Version B, you won't know which one influenced the results.
- Create Multiple Versions: If your test involves multiple elements (e.g., both headline and CTA button), create variations for each element combination. This allows you to test their individual and combined effects.
2. Randomization and Control Groups
Randomization and the use of control groups are critical to ensuring the validity of your A/B test:
- Random Assignment: Visitors should be randomly assigned to either Version A or Version B. This randomization helps eliminate bias and ensures that the groups are comparable.
Example: An e-commerce website randomly displays either Version A or Version B to incoming visitors, giving each version an equal chance to be seen. - Control Group: The control group (Version A) is essential because it provides a baseline for comparison. It represents how your webpage or content performs without any changes.
- Avoiding Selection Bias: Make sure your method of assigning visitors to groups doesn't introduce bias. For instance, don't assign all new visitors to one version and returning visitors to another.
- Balanced Traffic: Aim for a roughly equal distribution of traffic between the two versions to ensure that the results are representative of your overall audience.
3. Implement Tracking and Analytics
Accurate tracking and robust analytics are vital for gathering insights from your A/B test:
- Tracking Tools: Implement tracking tools like Google Analytics, Mixpanel, or dedicated A/B testing platforms to collect data on user behavior, conversions, and key metrics.
- Event Tracking: Set up event tracking to monitor specific user interactions related to your objectives. This might include tracking clicks on CTA buttons, form submissions, or product purchases.
- Goal Configuration: Define clear goals within your analytics platform to accurately measure conversions and other relevant actions.
- Data Consistency: Ensure that your tracking code is correctly implemented on both variations to avoid data discrepancies.
- Real-Time Monitoring: Monitor the test in real-time to detect any issues or anomalies that may arise during the test period.
- A/B Testing Software: Consider using dedicated A/B testing software that provides randomization, tracking, and reporting features. These tools can simplify the process and offer advanced analytics.
- Data Privacy and Compliance: Adhere to data privacy regulations, such as GDPR or CCPA, when collecting and handling user data.
By setting up your A/B test with well-designed variations, proper randomization, and robust tracking and analytics, you'll ensure that you can accurately measure the impact of your changes and draw meaningful conclusions from the results.
How to Run an A/B Test?
Now that you've set up your A/B test with clear objectives, variations, and tracking, it's time to run the test. We will guide you through the test period, monitoring and data collection, and how to handle external factors that may impact your results.
1. Running the Test Period
Running the test period is a crucial step in A/B testing, as it determines the duration for which you'll collect data. Here's how to manage this phase effectively:
- Define a Test Duration: Decide how long you will run the test. It's essential to balance between collecting enough data for statistical significance and minimizing the time it takes to make informed decisions.
- Statistical Significance: Monitor the progress of your test and keep an eye on the statistical significance of your results. You may need to extend the test duration if significance hasn't been reached.
- Consider Visitor Behavior: Take into account visitor behavior patterns, such as daily or weekly cycles. Ensure your test duration covers a representative sample of your audience.
- Avoid Seasonal Biases: If your website or app experiences seasonal variations in traffic, consider running the test for a full cycle to account for these fluctuations.
- Split Traffic Evenly: Ensure that traffic is split evenly between the two variations throughout the test period. Any imbalance can skew your results.
- Limit Changes: Resist making changes to the test variations during the test period, as this can introduce confounding factors.
2. Monitoring and Data Collection
Effective monitoring and data collection are essential to ensure the integrity of your A/B test:
- Regularly Check Data: Monitor your test daily or at regular intervals to ensure that data collection is functioning correctly. Look for any anomalies or technical issues.
- Event Tracking: Pay special attention to the events you're tracking, such as clicks or conversions. Verify that these events are recorded accurately.
- Traffic Volume: Keep an eye on the volume of traffic to each variation. Significant deviations can impact the reliability of your results.
- Engagement Metrics: Monitor engagement metrics like bounce rate, session duration, and page views to understand how users are interacting with your variations.
- Goal Conversions: Track the progress of your goals and conversions throughout the test period to assess whether your objectives are being met.
- Segmentation: Consider segmenting your data by user demographics or behavior to gain deeper insights into how different groups are responding to the variations.
- Data Backup: Ensure you have backups of your data in case of technical issues or data loss during the test.
3. Dealing with External Factors
External factors can influence your A/B test results, and it's essential to account for them:
- Seasonality: If your business experiences seasonal fluctuations, acknowledge that these can impact your results. Make seasonal adjustments if necessary, or consider running the test over multiple seasons.
- Marketing Campaigns: Be aware of any ongoing marketing campaigns or promotions that may affect user behavior. Ideally, run tests during periods of relative stability in your marketing efforts.
- Technical Glitches: Address any technical issues promptly to ensure they don't skew your results. For example, if your website experiences downtime, it can affect your data collection.
- External Events: Consider external events that might influence user behavior, such as industry news, holidays, or global events. While you can't control these factors, awareness is critical to interpreting your results.
- A/B Test Duration: Ensure that the duration of your A/B test is long enough to account for external factors. Longer tests can help smooth out the impact of short-term disruptions.
- Documentation: Document any significant external events or anomalies that occurred during the test period. This information can be valuable when analyzing your results.
Effectively managing the test period, monitoring data collection, and addressing external factors are critical for obtaining reliable results from your A/B test.
How to Analyze A/B Testing Results?
Once you've completed the test phase of your A/B test, it's time to analyze the results. This critical step involves assessing the statistical significance of your findings, interpreting the data, and drawing meaningful conclusions.
Statistical Significance
Statistical significance is a fundamental concept in A/B testing. It helps you determine whether the differences observed between variations A and B are meaningful or simply due to chance. Here's how to assess statistical significance:
- Choose a Significance Level: Decide on a significance level, often denoted as alpha (α), which represents the probability of making a Type I error (incorrectly concluding a significant difference when none exists). Common significance levels are 0.05 (5%) and 0.01 (1%).
- Calculate P-Value: Conduct a statistical test, such as a t-test or chi-squared test, to calculate the p-value. The p-value represents the probability of observing the results you obtained if there were no real differences between the variations.
Example: If your p-value is 0.03 (3%), it means there is a 3% chance that the observed differences occurred by random chance. - Compare P-Value to Significance Level: If the p-value is less than or equal to your chosen significance level (α), typically 0.05, then the results are considered statistically significant. This suggests that the differences between variations A and B are likely not due to chance.
Example: If α = 0.05 and your p-value is 0.03, the results are statistically significant. - Non-Significant Results: If the p-value is greater than α, the results are not statistically significant, indicating that the differences observed could be due to random variation.
- Effect Size: Consider the effect size, which measures the practical significance of the differences between variations. A small effect size may be statistically significant but not practically meaningful.
- Sample Size: Larger sample sizes can detect smaller effects and are more likely to yield statistically significant results.
Understanding statistical significance is crucial because it helps you determine whether the changes you made in variation B had a real impact or were merely the result of chance.
Interpreting the Data
Interpreting the data involves digging deeper into the numbers and understanding the implications of your A/B test results. Here's how to approach data interpretation:
- Focus on Key Metrics: Start by examining the key metrics you identified during the planning phase. Look at how they have changed between variations A and B.
- Visualize Data: Create visual representations of your data, such as charts and graphs, to identify patterns and trends. Visualizations can make it easier to grasp the significance of the differences.
- Segment Data: Consider segmenting your data by different user characteristics or behaviors. This can reveal insights into how specific groups respond to the variations.
Example: If you're running an e-commerce A/B test, segmenting by first-time visitors and returning customers may show different behavior patterns. - Time-Based Analysis: Analyze the data over time to see if the impact of the changes is consistent or varies over the test period.
- Compare with Objectives: Compare the results against the clear objectives you set at the beginning of the test. Did the changes align with your goals?
- Qualitative Feedback: If available, gather qualitative feedback from users to gain insights into their preferences and reasons behind their actions.
- Consider User Experience: Don't just focus on quantitative metrics. Assess whether the changes have improved or worsened the overall user experience.
- Look for Unexpected Insights: Sometimes, A/B testing can reveal unexpected insights that go beyond your initial objectives. Be open to these discoveries.
Drawing Conclusions
Drawing conclusions is the final step in your A/B testing journey, where you make decisions based on the results:
- Identify the Winning Variation: If the results are statistically significant and align with your objectives, identify the winning variation (either A or B).
- Consider Practical Significance: Take into account the practical significance of the changes. Even if the results are statistically significant, ask yourself if the observed differences are meaningful in the context of your goals.
- Document Learnings: Document the insights gained from the A/B test, whether positive or negative. These learnings can inform future experiments and optimization efforts.
- Implement Changes: If variation B is the winner and the changes are deemed practically significant, implement the changes on your website, app, or marketing campaign.
- Continuous Improvement: Remember that A/B testing is an iterative process. Continue to test and optimize to achieve ongoing improvements.
- Share Insights: Share the results and insights with your team or stakeholders, fostering a data-driven culture within your organization.
By analyzing the results, interpreting the data effectively, and drawing meaningful conclusions, you can ensure that your A/B tests lead to informed decisions and continuous improvement in your digital assets.
How to Implement A/B Testing Changes?
After successfully conducting your A/B test and analyzing the results, it's time to implement the changes that will optimize your digital assets. Let's go through the process of choosing the winning variation, scaling successful changes, and documenting your learnings for future reference.
1. Choose the Winning Variation
Selecting the winning variation is a critical step in the A/B testing process. Here's how to make an informed decision:
- Consider Objectives: Revisit the clear objectives you set at the beginning of the A/B test. Choose the variation that best aligns with those objectives.
- Statistical Significance: Ensure that the chosen variation is the one that achieved statistical significance and outperformed the other variation(s) in terms of key metrics.
- Practical Significance: While statistical significance is essential, also consider the practical significance of the changes. Ask yourself whether the observed improvements are meaningful for your business or project.
- User Experience: Assess how the changes impact the overall user experience. Choose the variation that not only meets your objectives but also provides a better user journey.
- Long-Term Impact: Think about the long-term impact of your decision. Consider whether the changes can be sustained and whether they align with your broader strategy.
- Stakeholder Alignment: Ensure your decision is communicated and aligned with stakeholders, including team members, managers, and clients.
- Feedback Loop: If possible, gather feedback from users or customers regarding the changes. This can provide valuable insights into their preferences and satisfaction.
- Testing Rigor: Reflect on the rigor of your testing process. Ensure that the test was conducted properly, without errors or biases, and that the results are reliable.
By carefully considering these factors, you can confidently choose the winning variation that will lead to the desired improvements in your digital assets.
2. Scale Successful Changes
Scaling successful changes involves applying the lessons learned from your A/B test to other areas of your digital presence for broader impact. Here's how to do it effectively:
- Replicate the Changes: Implement the changes from the winning variation on a larger scale. This might involve updating multiple pages, sections, or campaigns that can benefit from the improvements.
- Consistency: Ensure the changes are consistent with your brand identity and messaging across all relevant areas.
- Testing Iteration: Continue to test and optimize as you scale the successful changes. A/B testing is an ongoing process, and there may be further refinements to make.
- Document Processes: Document the processes and guidelines for implementing successful changes. This can help maintain consistency and ensure that future updates align with what you've learned.
- Collaboration: Collaborate with cross-functional teams, including design, development, and marketing, to ensure a coordinated effort in implementing changes.
- Monitor Performance: Keep a close eye on the performance of the scaled changes. Monitor key metrics to confirm that the improvements observed in the A/B test are sustained over time.
- Feedback Loop: Maintain a feedback loop with users or customers to gather insights and make iterative improvements as needed.
Scaling successful changes not only maximizes the impact of your A/B testing but also promotes a culture of continuous improvement within your organization.
3. Document Learnings
Documenting your A/B testing learnings is essential for ongoing optimization and knowledge sharing. Here's how to effectively capture and utilize these insights:
- Create a Knowledge Repository: Establish a central repository or document where you can record the details of your A/B tests, including objectives, variations, results, and conclusions.
- Include Insights: Document the insights gained from each A/B test, both positive and negative. Describe what worked, what didn't, and why.
- Data and Metrics: Include data and metrics relevant to each test, along with statistical significance and practical significance considerations.
- Visuals and Examples: Use visuals, screenshots, and examples to illustrate the changes and results, making it easier for team members to understand.
- Share with Stakeholders: Share your A/B testing documentation with relevant stakeholders, such as product managers, designers, developers, and marketers, to facilitate knowledge sharing.
- Use for Future Testing: Use your documented learnings as a reference for future A/B tests. Past results can inform your testing hypotheses and strategies.
- Continuous Review: Regularly review and update your documentation as new insights and test results become available. This ensures that your knowledge remains up to date.
- Training and Onboarding: Use your documentation to train new team members and onboard them to your A/B testing process and best practices.
By systematically documenting your A/B testing learnings, you create a valuable resource that supports data-driven decision-making, fosters collaboration, and facilitates continuous improvement in your digital projects.
A/B Testing Tools
A/B testing is a powerful method for optimizing digital assets, but it's only as effective as the tools you use to implement and analyze your tests. A variety of A/B testing tools are available to help you streamline the process and gather meaningful insights. We'll explore the aspects of these tools and what to look for when choosing one, without mentioning specific tools except for Appinio.
What A/B Testing Tools Offer
A/B testing tools provide a range of features and functionalities to support your experimentation process. Here are some common elements you can expect from such tools:
- Variation Creation: Most A/B testing tools offer easy-to-use interfaces for creating and managing different variations of your digital assets.
- Randomization: They ensure that users are assigned to variations randomly to eliminate bias and maintain fairness in the testing process.
- Statistical Analysis: A/B testing tools typically include built-in statistical analysis capabilities to determine the significance of results.
- Conversion Tracking: These tools allow you to track conversions and key metrics accurately, helping you assess the impact of changes.
- Segmentation: Many tools enable you to segment your audience by various criteria, allowing you to gain insights into how different user groups respond to variations.
- Visualizations: Visual representations of data, such as charts and graphs, help you quickly understand the impact of changes.
- Data Export: Exporting raw data and results is essential for in-depth analysis and documentation.
What to Consider When Choosing an A/B Testing Tool?
Selecting the right A/B testing tool is crucial for the success of your experiments. Here are some factors to consider when making your choice:
- Ease of Use: Look for a tool with an intuitive interface that your team can navigate easily, even without a deep technical background.
- Statistical Rigor: Ensure the tool provides robust statistical analysis to accurately determine the significance of your results.
- Integration: Consider whether the tool integrates seamlessly with your existing tech stack, including analytics platforms and marketing tools.
- Audience Targeting: If your audience varies significantly, choose a tool that allows for precise audience segmentation.
- Budget: A/B testing tools come with varying pricing structures. Evaluate whether the tool's cost aligns with your budget and expected ROI.
- Support and Training: Check if the tool offers customer support, training resources, and documentation to assist you in getting the most out of it.
Remember that the choice of an A/B testing tool should align with your specific needs and goals. While there are many tools available, each may offer unique features and capabilities. The right tool can make your A/B testing process more efficient and insightful, ultimately leading to data-driven improvements in your digital assets.
Appinio is an excellent platform for obtaining real-time consumer insights, which can complement your A/B testing efforts by providing valuable feedback and input directly from your target audience.
A/B Testing Examples
To gain a deeper understanding of how A/B testing works in practice, let's explore some real-world examples of A/B tests conducted by businesses and organizations across different industries:
E-Commerce: Testing Product Page Layout
Scenario: An e-commerce company wants to optimize its product page layout to increase conversions and sales.
A/B Test: The company creates two variations of its product page:
- Version A: The control group with the existing product page layout.
- Version B: The experimental group with a redesigned layout that highlights product reviews and features a prominent call-to-action button.
Objective: Increase the click-through rate (CTR) on the "Add to Cart" button and ultimately boost sales.
Results: After running the A/B test, Version B shows a statistically significant 20% increase in the CTR compared to Version A. Users found the new layout more engaging and conversion-friendly, leading to a substantial revenue increase.
Content Marketing: Testing Headlines
Scenario: A news website aims to improve user engagement and click-through rates on its articles.
A/B Test: The news website conducts an A/B test on article headlines:
- Version A: Features the original headlines.
- Version B: Employs new, attention-grabbing headlines.
Objective: Increase the click-through rate (CTR) on article links and encourage more readers to explore the content.
Results: Version B outperforms Version A, showing a statistically significant 15% increase in CTR. The engaging headlines draw more readers to click on articles, resulting in higher page views and longer session durations.
Email Marketing: Testing Subject Lines
Scenario: An email marketing team wants to improve their newsletters' open rates and click-through rates.
A/B Test: The team conducts an A/B test on email subject lines:
- Version A: Features the original subject lines.
- Version B: Utilizes new, concise, and personalized subject lines.
Objective: Increase the open rate and click-through rate (CTR) of email newsletters to drive more traffic to the website.
Results: Version B achieves a statistically significant 10% increase in open rates and a 12% increase in CTR compared to Version A. The personalized and concise subject lines capture subscribers' attention more effectively.
Software: Testing Feature Adoption
Scenario: A software company aims to improve user engagement with a new feature in their application.
A/B Test: The company conducts an A/B test for the feature introduction:
- Version A: Features a brief tutorial upon the user's first encounter with the new feature.
- Version B: Offers tooltips and in-app guidance for users as they explore the feature.
Objective: Increase user adoption and engagement with the new feature.
Results: Version B shows a statistically significant 25% increase in feature adoption compared to Version A. Users find the in-app guidance more helpful, leading to increased usage of the new feature.
These examples highlight the versatility of A/B testing across various domains. Whether you're optimizing e-commerce websites, content marketing strategies, email campaigns, or software features, A/B testing empowers you to make data-driven decisions and continuously enhance user experiences and business outcomes. Remember that the specific variables, objectives, and metrics will vary depending on the unique goals of each A/B test.
A/B Testing Best Practices
A/B testing is a powerful tool, but you need to follow best practices to make the most of it. These practices ensure that your tests are accurate, reliable, and yield actionable insights. Here are some essential A/B testing tips to keep in mind:
- Test One Variable at a Time: When creating variations, focus on changing one variable at a time. This isolates the impact of the change, making it easier to understand the results. If you alter multiple elements simultaneously, you won't know which one influenced the outcome.
- Have Clear Objectives: Define clear, specific, and measurable objectives for your A/B tests. Without clear objectives, you risk running tests that don't provide actionable insights or align with your broader goals.
- Randomize and Control Groups: Ensure that you randomize the assignment of visitors to variations and always include a control group (Version A) that remains unchanged. This minimizes bias and provides a baseline for comparison.
- Sufficient Sample Size: Calculate and use an appropriate sample size to ensure statistical significance. Smaller sample sizes can lead to unreliable results, while larger samples are more likely to detect smaller effects.
- Statistical Significance: Understand the concept of statistical significance and choose a significance level (alpha) before conducting tests. Only draw conclusions from results that achieve statistical significance.
- Consider Practical Significance: While statistical significance is crucial, also evaluate the practical significance of changes. Ask whether the observed differences are meaningful and have a tangible impact on your objectives.
- Avoid Biases: Be vigilant about avoiding biases, both in the setup of your tests and the interpretation of results. Selection bias, confirmation bias, and other biases can lead to misleading conclusions.
- Segment Your Data: Segment your data by user demographics, behavior, or other relevant factors to gain insights into how different user groups respond to variations. This can uncover valuable insights that may not be apparent in aggregate data.
- Continuous Testing and Iteration: A/B testing is an ongoing process. Continuously test and optimize your digital assets to stay ahead of changing user preferences and market trends. Regularly revisit and update your tests and variations.
- Document Everything: Maintain detailed records of your A/B tests, including objectives, variations, results, and conclusions. Documentation ensures that you can learn from past tests and share insights with your team.
- Implement Changes Promptly: Once you've identified a winning variation, implement the changes promptly. Delays in implementation can lead to missed opportunities for improvement.
- User Feedback: Incorporate user feedback into your testing process. Collect qualitative insights from users to gain a deeper understanding of their preferences and motivations.
- Data Privacy and Compliance: Adhere to data privacy regulations, such as GDPR or CCPA, when collecting and handling user data. Ensure that your testing processes comply with relevant laws and regulations.
- Educate Your Team: Educate your team members on A/B testing best practices and principles. Encourage a data-driven culture within your organization, where decisions are based on evidence rather than assumptions.
- Share Results and Insights: Share the results and insights from your A/B tests with relevant stakeholders. Transparency and communication help align teams and foster collaboration.
By adhering to these A/B testing best practices, you can maximize the effectiveness of your tests, make informed decisions, and continuously improve your digital assets to better meet your objectives and user needs.
Conclusion
A/B testing is your key to unlocking the potential of data-driven optimization. By conducting systematic experiments and analyzing results, you can make informed decisions, improve user experiences, and drive better outcomes in the digital world. Remember, the journey of continuous improvement through A/B testing never ends; it's a path to ongoing success in the ever-evolving online landscape.
As you embark on your A/B testing journey, keep in mind that success comes from a combination of clear objectives, meticulous planning, unbiased testing, and thoughtful analysis. By following best practices, staying agile, and leveraging the insights gained from each test, you'll be on the path to achieving your digital goals and staying ahead of the competition.
How to Conduct A/B Testing in Minutes?
Imagine conducting A/B tests with lightning speed, obtaining consumer insights in minutes, and making data-driven decisions effortlessly. That's the power of Appinio, the real-time market research platform redefining the game. Here's why you should consider Appinio for your A/B testing endeavors:
- Rapid Insights: Appinio's platform delivers answers in minutes, enabling you to make swift decisions based on real-time consumer feedback.
- User-Friendly: No need for a research PhD. Appinio's intuitive interface empowers anyone to conduct A/B tests with ease.
- Global Reach: Define your target audience from 1,200+ characteristics and survey consumers in over 90 countries, ensuring your tests are truly representative.
Get facts and figures ðŸ§
Want to see more data insights? Our free reports are just the right thing for you!