What is a Control Group? Definition, Uses, Examples
Appinio Research · 06.03.2024 · 33min read
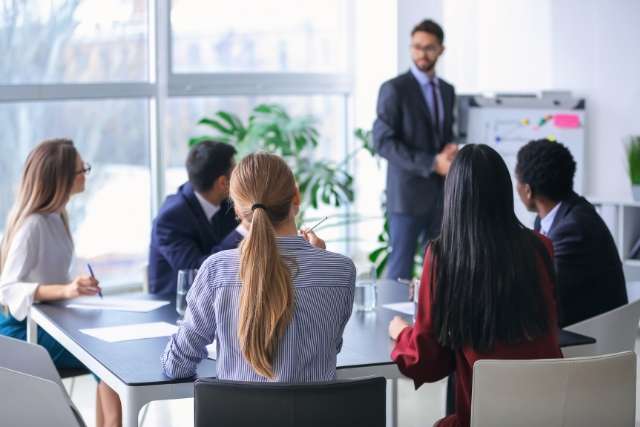
Content
Have you ever wondered how researchers determine if a new medication is effective, or how businesses evaluate the impact of their marketing campaigns? The answer lies in control groups. Control groups serve as a benchmark for comparison in research studies and business experiments, allowing researchers and businesses to assess the true effects of interventions or strategies. By carefully designing control groups and comparing outcomes between treatment and control groups, researchers can draw valid conclusions about causality, while businesses can make informed decisions about resource allocation and strategy development.
In this guide, we'll explore everything you need to know about control groups, from their definition and purpose to their implementation and analysis. Whether you're a researcher conducting experiments or a business owner testing new initiatives, understanding control groups is essential for achieving reliable results and driving evidence-based decision-making.
What is a Control Group?
Control groups are a fundamental concept in research methodology, serving as a critical component in experimental design. Understanding their definition and purpose is essential for researchers aiming to conduct rigorous and valid studies.
A control group is a standardized reference group against which the effects of a treatment or intervention are compared. They provide a baseline for assessing the effectiveness of an intervention by allowing researchers to isolate the effects of the treatment from other variables that may influence the outcome.
The primary purpose of control groups is to minimize bias and confounding variables, thereby enabling researchers to draw valid conclusions about the causal relationship between the independent variable (the treatment) and the dependent variable (the outcome). By comparing outcomes between the treatment group (those receiving the intervention) and the control group (those not receiving the intervention), researchers can determine whether any observed changes are truly due to the intervention or simply occur by chance.
Importance of Control Groups in Research and Business
Control groups play a crucial role in both research and business contexts, offering valuable insights and driving evidence-based decision-making. Here are some key reasons why control groups are important:
- Establishing Causality: Control groups are essential for establishing causality in research studies by providing a basis for comparison. They allow researchers to determine whether changes in the outcome variable can be attributed to the intervention itself rather than to other factors.
- Validating Results: Control groups help validate the results of a study by providing a standard against which the effects of the intervention can be evaluated. Comparing outcomes between the treatment and control groups allows researchers to assess the effectiveness and efficacy of the intervention.
- Minimizing Bias: By controlling for extraneous variables and confounding factors, control groups help minimize bias in research studies. This enhances the internal validity of the study and increases confidence in the findings.
- Informing Decision-Making: In business settings, control groups are used to evaluate the impact of marketing campaigns, product launches, and other business initiatives. By comparing outcomes between exposed and unexposed groups, businesses can make data-driven decisions about resource allocation and strategy development.
- Optimizing Resource Allocation: Control groups help businesses optimize resource allocation by identifying which interventions or strategies are most effective in achieving desired outcomes. This allows businesses to allocate resources more efficiently and maximize return on investment.
- Improving Customer Experience: Control groups can be used to test changes to products, services, or processes before implementation, helping businesses identify potential risks and opportunities to improve the customer experience.
Overall, control groups serve as a cornerstone of rigorous research and evidence-based decision-making in both academic and business settings. By providing a standard for comparison and minimizing bias, control groups enable researchers and businesses to draw meaningful conclusions, optimize resource allocation, and drive innovation and growth.
How to Design a Control Group?
Now that we understand the importance of control groups, let's explore how to design them effectively to ensure robust research outcomes.
Identifying Variables
Identifying variables is the foundational step in designing a control group. It involves clearly defining the independent, dependent, and potentially confounding variables in your study.
- Independent Variable: This is the variable that you manipulate or change in your experiment. For example, if you're investigating the effect of a new medication on blood pressure, the independent variable would be the administration of the medication.
- Dependent Variable: The dependent variable is the outcome you measure to assess the impact of the independent variable. In our medication example, the dependent variable would be the change in blood pressure after administering the medication.
- Confounding Variables: These are variables that could potentially influence the outcome of your study but are not the focus of your investigation. Identifying and controlling for these variables is essential to ensure that any observed effects are genuinely due to the independent variable.
Randomization Techniques
Randomization is a critical aspect of control group design that helps minimize bias and ensure the comparability of treatment and control groups.
- Simple Randomization: In simple randomization, participants are randomly assigned to either the treatment or control group. This approach helps distribute potential confounding variables evenly across groups, reducing the risk of bias.
- Stratified Randomization: This technique involves dividing participants into homogeneous subgroups based on specific characteristics (e.g., age, gender, severity of condition) and then randomly assigning them to treatment or control groups within each subgroup. Stratified randomization helps ensure that each group is balanced in terms of important variables, enhancing the validity of the study results.
- Blocked Randomization: Blocked randomization involves grouping participants into blocks of equal size and then randomly assigning them to treatment or control groups within each block. This method ensures that each group maintains balance throughout the study, even if the sample size is small.
Matching Methods
Matching methods are another approach to control group design that involves pairing participants in the treatment and control groups based on specific characteristics.
- Propensity Score Matching: Propensity score matching involves calculating a propensity score for each participant, which represents the likelihood of being assigned to the treatment group based on observed characteristics. Participants in the control group are then matched to participants in the treatment group based on similar propensity scores, ensuring balance between the groups.
- Exact Matching: Exact matching involves pairing participants in the treatment and control groups with identical or very similar characteristics. This method is particularly useful when there are only a few variables to match on and when a precise match is feasible.
Stratified Sampling
Stratified sampling involves dividing the population into homogeneous subgroups (or strata) based on certain characteristics and then randomly selecting participants from each stratum to form the treatment and control groups.
- Proportional Stratified Sampling: In proportional stratified sampling, the number of participants selected from each stratum is proportional to the size of the stratum in the overall population. This ensures that each subgroup is adequately represented in the sample.
- Disproportional Stratified Sampling: Disproportional stratified sampling involves intentionally oversampling specific subgroups to ensure an adequate sample size for analysis. This approach is handy when certain subgroups are of particular interest or when there are small subgroups that need to be represented adequately.
Stratified sampling helps ensure that each population subgroup is represented in the study sample, allowing for more accurate comparisons between the treatment and control groups. By controlling for potential confounding variables through randomization, matching, and stratification, researchers can enhance the internal validity of their studies and draw more reliable conclusions about the effects of the independent variable.
Types of Control Groups
When designing a study, you have several options for the type of control group to use. Each type serves a specific purpose and offers unique advantages and limitations.
Placebo Control Group
A placebo control group is a standard against which the effects of a treatment are compared. Participants in this group receive a placebo—an inactive substance or treatment that resembles the active treatment but has no therapeutic effect. Placebo control groups are instrumental in clinical trials for evaluating the efficacy of new medications or interventions.
Purpose: The primary purpose of a placebo control group is to assess the extent to which any observed effects are due to the treatment itself rather than to placebo effects or other extraneous factors.
Example: In a study evaluating the effectiveness of a new pain medication, participants in the placebo control group would receive a sugar pill or inert substance instead of the active medication. By comparing outcomes between the treatment and placebo groups, researchers can determine whether the medication has a significant effect beyond placebo.
Active Control Group
Unlike a placebo control group, an active control group receives an established treatment or intervention rather than a placebo. The purpose of an active control group is to compare the effects of the experimental treatment against those of an existing standard of care or alternative intervention.
Purpose: Active control groups help establish whether the experimental treatment is superior, inferior, or equivalent to the active comparator. This design is advantageous when ethical considerations preclude the use of a placebo or when there is an established standard of care for the condition being studied.
Example: In a clinical trial comparing two antidepressant medications, one group of participants would receive the experimental medication, while another group would receive a standard antidepressant that has already been proven effective. By comparing outcomes between the two groups, researchers can assess the relative efficacy and safety of the new medication.
Historical Control Group
A historical control group consists of data or outcomes from previous studies or existing databases that serve as a comparison for the current study. Rather than recruiting new participants, researchers use historical data to assess the effectiveness of a treatment or intervention.
Purpose: Historical control groups are useful when it is impractical, unethical, or cost-prohibitive to conduct a new study with a control group. They allow researchers to leverage existing data to inform current research questions and hypotheses.
Example: Suppose researchers are investigating the effectiveness of a new surgical procedure for treating a particular condition. Instead of enrolling new participants in a control group, they may compare the outcomes of patients who underwent the new procedure to historical data from patients who received standard treatment in previous studies or clinical registries.
No-Treatment Control Group
In a no-treatment control group, participants do not receive any form of treatment or intervention. This type of control group is beneficial for assessing the natural progression of a condition or disease over time.
Purpose: The primary purpose of a no-treatment control group is to determine whether any observed changes in the treatment group are attributable to the intervention itself rather than to spontaneous recovery, regression to the mean, or other external factors.
Example: In a study examining the effectiveness of behavioral therapy for anxiety disorders, participants in the treatment group would receive therapy sessions, while those in the no-treatment control group would not receive any intervention. By comparing outcomes between the two groups, researchers can assess the specific effects of the therapy beyond the natural course of the disorder.
Each type of control group has its own advantages and limitations, and the choice of which to use depends on various factors, including the research question, ethical considerations, and practical constraints.
By carefully selecting and implementing the appropriate type of control group, researchers can strengthen the validity and reliability of their findings, ultimately advancing scientific knowledge and improving patient care.
How to Implement a Control Group?
Now that we've covered the different types of control groups, let's explore the essential steps involved in implementing them effectively within your research study.
Recruitment and Selection Process
The recruitment and selection process for control group participants is crucial to ensure the validity and representativeness of your study sample.
- Clear Eligibility Criteria: Define clear eligibility criteria for inclusion in the control group based on the objectives of your study. Consider factors such as age, gender, medical history, and specific characteristics relevant to your research question.
- Ethical Recruitment Practices: Ensure that the recruitment process adheres to ethical guidelines and principles, such as voluntary participation, informed consent, and confidentiality. Clearly communicate the purpose and procedures of the study to potential participants to facilitate informed decision-making.
- Randomization and Allocation: Use randomization techniques to assign participants to either the treatment or control group. Random assignment helps minimize selection bias and ensures that the groups are comparable in terms of baseline characteristics.
Ethical Considerations
Ethical considerations are paramount when implementing control groups in research studies to protect the rights and well-being of participants.
- Informed Consent: Obtain informed consent from all participants in the control group, ensuring that they fully understand the purpose, risks, benefits, and procedures of the study before agreeing to participate. Provide ample opportunity for participants to ask questions and withdraw consent at any time without penalty.
- Minimizing Harm: Take measures to mitigate any potential harm or discomfort to participants in the control group. Ensure that the control group receives appropriate care and attention, even if they do not receive the experimental treatment.
- Equitable Access: Ensure equitable access to the intervention or treatment being studied, particularly in cases where the control group may be denied access to potentially beneficial interventions. Consider alternative study designs or ethical safeguards to mitigate concerns about fairness and access.
Sample Size Determination
Determining the appropriate sample size for the control group is essential to ensure the statistical power and reliability of your study findings.
- Power Analysis: Conduct a power analysis to determine the minimum sample size required to detect a meaningful effect size with adequate statistical power. Consider factors such as the desired level of significance, effect size, and expected variability in the outcome measure.
- Consideration of Dropouts: Anticipate potential dropout rates and adjust the sample size accordingly to maintain adequate statistical power throughout the study. Implement strategies to minimize dropout rates, such as providing incentives for participation and maintaining regular communication with participants.
- Balancing Groups: Ensure that the control group is sufficiently large to provide a reliable comparison to the treatment group. Aim for a balanced distribution of participants across groups to enhance the study's internal validity.
Allocation Concealment
Allocation concealment is a critical aspect of control group implementation that helps prevent selection bias and ensures the integrity of the randomization process.
- Blinding Procedures: Implement blinding procedures to conceal the allocation of participants to the treatment or control group from both researchers and participants. Blinding helps minimize conscious or unconscious biases that could influence study outcomes.
- Use of Sequentially Numbered, Opaque Sealed Envelopes: Employ sequentially numbered, opaque sealed envelopes (SNOSE) or similar methods to conceal the allocation sequence until after participants have been recruited and enrolled in the study. This helps maintain the integrity of the randomization process and prevents potential manipulation or bias.
- Centralized Randomization: Consider using centralized randomization procedures administered by an independent third party to ensure unbiased allocation of participants to treatment and control groups. Centralized randomization minimizes the risk of inadvertent bias or manipulation by study personnel.
By carefully implementing control groups and adhering to ethical principles and best practices, you can enhance the validity, reliability, and ethical integrity of your research study. Paying close attention to recruitment and selection processes, ethical considerations, sample size determination, and allocation concealment will help ensure that your research yields meaningful and trustworthy results.
How to Manage Control Groups?
Once your control groups are established, it's essential to manage and monitor them effectively throughout the course of your research study. Let's explore the key aspects of managing and monitoring control groups.
Data Collection Procedures
Robust data collection procedures are essential to ensure the accuracy and reliability of your study findings. Here's how to effectively manage data collection in control groups:
- Standardized Protocols: Develop standardized protocols for data collection to ensure consistency across participants and time points. Clearly define variables, measurement instruments, and procedures to minimize variability and bias.
- Trained Personnel: Train research personnel thoroughly on data collection procedures to ensure competency and reliability. Provide ongoing supervision and support to maintain adherence to protocols and minimize errors.
- Quality Assurance Measures: Implement quality assurance measures, such as regular data audits and inter-rater reliability assessments, to monitor data collection accuracy and identify any discrepancies or inconsistencies.
- Participant Engagement: Foster strong rapport with control group participants to enhance engagement and compliance with data collection procedures. Provide clear instructions and support to facilitate accurate reporting and minimize missing data.
As you embark on the journey of managing and monitoring your control groups, remember the crucial role that robust data collection plays in ensuring the success of your research study. By implementing standardized protocols, training personnel effectively, and maintaining quality assurance measures, you can elevate the integrity of your study findings and unlock valuable insights. At Appinio, we understand the importance of data accuracy and reliability, which is why our platform empowers you to gather real-time consumer insights effortlessly.
Ready to take your research to the next level? Book a demo today and discover how Appinio can streamline your data collection process and drive informed decision-making!
Blinding and Double-Blinding
Blinding, or masking, is a critical methodological technique used to minimize bias in research studies. Let's explore how blinding and double-blinding can be effectively implemented in control groups:
- Single-Blinding: In single-blinding, either the participants or the researchers are unaware of the participants' group assignments (treatment or control). Single-blinding helps minimize performance bias and subjective influence on outcomes.
- Double-Blinding: Double-blinding involves keeping both the participants and the researchers unaware of group assignments. This approach helps minimize both performance bias and detection bias, enhancing the internal validity of the study.
- Blinding Procedures: Implement robust blinding procedures, such as using identical placebo treatments for control group participants or employing independent assessors who are unaware of group assignments to collect outcome data.
Controlling for Bias
Controlling for bias is essential to ensure the validity and reliability of study findings. Here are strategies to minimize bias in control groups:
- Randomization: Use randomization techniques to assign participants to treatment and control groups, minimizing selection bias and ensuring comparability between groups.
- Matching: Employ matching methods to pair participants in the treatment and control groups based on relevant characteristics, such as age, gender, or disease severity, to minimize selection bias and confounding variables.
- Statistical Analysis: Use appropriate statistical techniques, such as analysis of covariance (ANCOVA) or propensity score matching, to control for potential confounding variables and minimize bias in outcome comparisons.
Handling Dropouts and Attrition
Dropouts and attrition can pose challenges to the integrity of control groups. Here's how to effectively manage and address these issues:
- Retention Strategies: Implement proactive retention strategies, such as regular follow-up communication, incentives for participation, and flexible scheduling, to minimize dropout rates and enhance participant retention.
- Intent-to-Treat Analysis: Conduct intent-to-treat analysis, which includes all participants according to their original group assignment, regardless of whether they completed the study. Intent-to-treat analysis helps maintain the integrity of randomization and provides a more conservative estimate of treatment effects.
- Sensitivity Analysis: Conduct sensitivity analysis to assess the impact of missing data or dropout rates on study findings. Explore different scenarios and assumptions to evaluate the robustness of your results.
- Imputation Techniques: Consider using imputation techniques, such as last observation carried forward (LOCF) or multiple imputation, to handle missing data and minimize bias in outcome analyses.
By effectively managing data collection, implementing blinding procedures, controlling for bias, and addressing dropouts and attrition, you can ensure the validity and reliability of your study findings and maximize the impact of your research.
Paying careful attention to these aspects of managing and monitoring control groups will help you draw meaningful conclusions and advance scientific knowledge in your field.
Examples of Control Groups
To better understand the concept of control groups and their application in research and business, let's explore some real-world examples:
Clinical Trials
In clinical trials testing the effectiveness of a new medication, participants are randomly assigned to either the treatment group, which receives the medication, or the control group, which receives a placebo or standard treatment. By comparing outcomes between the two groups, researchers can determine whether the medication has a significant effect beyond placebo or existing treatments.
For example, in a study evaluating a new cholesterol-lowering drug, the control group might receive a placebo pill while the treatment group receives the actual medication. Comparing changes in cholesterol levels between the two groups allows researchers to assess the drug's efficacy.
Marketing Experiments
In marketing experiments, control groups are used to evaluate the impact of marketing campaigns, promotions, or product launches. For instance, suppose a company wants to assess the effectiveness of a new advertising campaign in increasing sales. They may divide their target audience into two groups: one exposed to the advertising campaign (the treatment group) and one not exposed (the control group).
The company can measure the campaign's effectiveness in driving sales by comparing sales data between the two groups over a specific period. This helps businesses make data-driven decisions about marketing strategies and resource allocation.
Product Testing
Control groups are also used in product testing to assess the effectiveness or safety of consumer products. For instance, in a study evaluating the efficacy of a new skincare product in reducing wrinkles, participants may be assigned to either the treatment group, which applies the new product, or the control group, which uses a placebo or standard skincare product.
By comparing changes in wrinkle depth or skin texture between the two groups, researchers can determine the product's effectiveness in achieving its intended purpose.
These examples illustrate the versatility of control groups across various domains, highlighting their importance in facilitating rigorous experimentation, evidence-based decision-making, and advancements in research and business practices.
Whether in healthcare, marketing, education, or product development, control groups serve as invaluable tools for evaluating interventions, measuring effectiveness, and informing impactful outcomes.
How to Analyze Control Group Results?
Now that you have collected data from your control groups, it's time to analyze the results. Effective analysis is crucial for drawing meaningful conclusions and informing decisions based on your research findings. Let's explore the key steps involved in interpreting results from control groups.
Statistical Methods
Statistical methods are essential for analyzing the data collected from control groups. Here are some standard statistical techniques used in analyzing control group data:
- Descriptive Statistics: Begin by computing descriptive statistics, such as means, standard deviations, and frequencies, to summarize the characteristics of the control group and the outcome variables of interest.
- Inferential Statistics: Use inferential statistics to test hypotheses and determine whether any observed differences between the treatment and control groups are statistically significant. Common inferential tests include t-tests, analysis of variance (ANOVA), chi-square tests, and regression analysis.
- Power Analysis: Conduct a power analysis to determine whether your study had sufficient statistical power to detect meaningful differences between the treatment and control groups. Power analysis helps ensure that your study results are reliable and interpretable.
Comparing Treatment and Control Groups
Once you have analyzed the data, it's essential to compare the outcomes between the treatment and control groups to assess the effectiveness of the intervention. Here's how to compare treatment and control groups effectively:
- Quantitative Comparison: Compare the means or proportions of outcome variables between the treatment and control groups using appropriate statistical tests. Pay attention to effect sizes and confidence intervals to assess the magnitude and precision of any observed differences.
- Qualitative Comparison: In addition to quantitative comparisons, consider qualitative factors such as clinical significance, practical relevance, and contextual factors that may influence the interpretation of study findings.
- Subgroup Analysis: Conduct subgroup analyses to explore whether the treatment effect varies across different subgroups of participants. This can help identify potential moderators or effect modifiers that may influence the effectiveness of the intervention.
Interpreting Findings
Interpreting the findings from control group analysis requires careful consideration of various factors and contextual information. Here are some tips for interpreting study findings effectively:
- Contextual Understanding: Consider the broader context in which the study was conducted, including patient population characteristics, study design, intervention protocol, and implementation fidelity.
- Clinical Relevance: Evaluate the clinical relevance of any observed differences between the treatment and control groups. Consider factors such as the magnitude of effect, the practical significance of findings, and implications for clinical practice.
- Strengths and Limitations: Discuss the strengths and limitations of the study, including potential sources of bias, confounding variables, and constraints of the study design or methodology. Transparency about the study's limitations enhances the credibility and trustworthiness of the findings.
Reporting Results
Finally, it's crucial to communicate your study findings clearly and effectively through written or oral presentations. Here are some tips for reporting results from control group analysis:
- Structured Format: Organize your results section in a clear and structured format, following the guidelines of your target journal or audience. Present key findings, including descriptive statistics, inferential results, and comparisons between treatment and control groups.
- Visual Aids: Use tables, graphs, and figures to visually represent your data and highlight key findings. Visual aids enhance the readability and interpretability of your results and facilitate comparison across different study groups or variables.
- Discussion of Implications: Discuss the implications of your findings in the context of existing literature, clinical practice, and theoretical frameworks. Consider how your results contribute to understanding the research question and identify directions for future research or clinical practice.
- Transparency and Reproducibility: Ensure transparency and reproducibility by providing sufficient detail about the study methods, data analysis procedures, and results interpretation. This facilitates peer review, replication, and further validation of your findings by other researchers.
By following these steps and guidelines for analyzing results from control groups, you can effectively evaluate the impact of your intervention, draw meaningful conclusions, and contribute to advancing knowledge in your field. Effective analysis and reporting of control group results are essential for informing evidence-based decision-making and improving patient outcomes.
Control Group Challenges and Solutions
Navigating control groups in research can be complex, and researchers often encounter various challenges along the way. Here are some common challenges and practical solutions to address them:
- Recruitment Difficulties: Difficulty in recruiting participants for the control group can arise due to various factors such as stringent eligibility criteria, lack of participant motivation, or limited access to target populations. To overcome this challenge, researchers can expand recruitment strategies, collaborate with community organizations, offer incentives for participation, or adjust eligibility criteria to increase inclusivity.
- Ethical Dilemmas: Ethical considerations are paramount in research involving control groups, especially when participants in the control group may not receive the intervention being studied. To navigate ethical dilemmas, researchers should prioritize participant well-being, ensure informed consent, minimize harm, and uphold principles of justice and goodwill. Consulting with ethics committees or institutional review boards can provide guidance on ethical issues.
- Sample Size Limitations: Determining an appropriate sample size for control groups can be challenging, particularly in studies with limited resources or rare populations. Researchers can address sample size limitations by conducting power analyses to estimate the required sample size based on effect size and desired statistical power. Collaboration with other research groups or leveraging existing datasets may also help increase sample size and enhance study power.
- Measurement Bias: Measurement bias can occur when outcome measures are not valid, reliable, or sensitive to detect changes accurately. To mitigate measurement bias, researchers should use validated instruments, implement standardized assessment protocols, train research personnel thoroughly, and conduct pilot testing to ensure the reliability and validity of outcome measures. Additionally, blinding assessors to group assignments can help minimize subjective bias in data collection.
- Loss to Follow-Up: Attrition or loss to follow-up of participants in the control group can threaten the internal validity and generalizability of study findings. Researchers can mitigate loss to follow-up by maintaining regular contact with participants, offering incentives for retention, employing multiple contact methods (e.g., phone calls, emails, text messages), and tracking participants' progress systematically. Additionally, conducting sensitivity analyses to assess the impact of attrition on study results can help evaluate the robustness of findings.
- Confounding Variables: Controlling for confounding variables is essential to accurately isolate the independent variable's effects. However, identifying and accounting for all potential confounders can be challenging. Researchers can address confounding variables by using randomization techniques, matching methods, or statistical adjustment (e.g., regression analysis) to control for known confounders. Sensitivity analyses can also help evaluate the robustness of study findings to potential confounding.
- Publication Bias: Publication bias occurs when studies with positive or significant results are more likely to be published than those with null or negative results, leading to an incomplete and biased representation of the evidence base. Researchers can mitigate publication bias by preregistering study protocols, submitting study findings to preprint servers, publishing results regardless of statistical significance, and participating in meta-analyses or systematic reviews to provide a comprehensive synthesis of the literature.
- Resource Constraints: Limited resources, including funding, time, and personnel, can pose challenges in conducting research with control groups. Researchers can optimize resource utilization by prioritizing research objectives, seeking external funding opportunities, collaborating with interdisciplinary teams or community partners, leveraging existing infrastructure or datasets, and implementing efficient study designs and procedures. Additionally, documenting resource allocation and justifying budgetary decisions can enhance transparency and accountability in research management.
By proactively identifying and addressing these common challenges, researchers can enhance the quality, rigor, and impact of their research involving control groups, ultimately advancing scientific knowledge and improving outcomes in various fields.
Conclusion for Control Groups
Control groups are indispensable tools in both research and business settings, enabling us to assess the true impact of interventions and strategies. By providing a baseline for comparison, control groups help us determine causality and make informed decisions. Whether we're evaluating the effectiveness of a new medication in a clinical trial or assessing the impact of a marketing campaign in a business experiment, control groups ensure that our findings are valid and reliable.
Incorporating control groups into our studies and experiments requires careful planning and implementation, but the benefits they provide are invaluable. From minimizing bias to optimizing resource allocation, control groups empower us to draw meaningful conclusions and drive evidence-based decision-making. By understanding and utilizing control groups effectively, we can advance knowledge, improve outcomes, and ultimately make a positive impact in our respective fields.
How to Run a Control Group in Minutes?
Introducing Appinio, your ultimate companion in the world of control groups and real-time market research! As a leading real-time market research platform, Appinio empowers companies to gain instant consumer insights, revolutionizing decision-making processes. With Appinio, conducting your own market research becomes a breeze, allowing you to make fast, data-driven decisions for your business using real-time consumer insights.
Here's why you should choose Appinio:
- Rapid Insights: From posing questions to uncovering valuable insights, Appinio delivers results in minutes, ensuring you have the information you need when you need it most.
- Intuitive Platform: No need for a PhD in research here! Appinio's user-friendly platform is designed for everyone, making market research accessible and enjoyable for all.
- Global Reach: With access to over 90 countries and the ability to define target groups based on 1200+ characteristics, Appinio enables you to reach your desired audience effortlessly, no matter where they are located.
Get facts and figures 🧠
Want to see more data insights? Our free reports are just the right thing for you!