What is Inferential Statistics? Definition, Types, Examples
Appinio Research · 18.01.2024 · 47min read
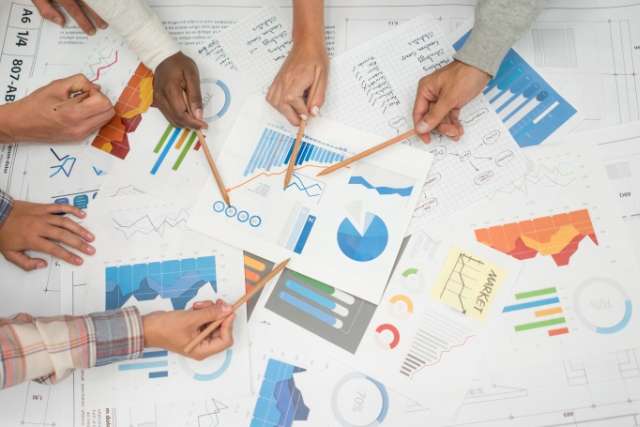
Content
Ever wondered how we can make confident predictions and informed decisions about large populations with just a limited sample of data? Inferential statistics holds the answer. In this, we'll unravel the world of inferential statistics, equipping you with the knowledge and tools to unlock valuable insights from data, test hypotheses, and navigate the fascinating realm where numbers illuminate the bigger picture.
Whether you're a student, researcher, or professional, this guide will demystify the complexities, making inferential statistics an accessible and powerful tool in your analytical arsenal.
What is Inferential Statistics?
Inferential statistics is a branch of statistics that enables us to make inferences and draw conclusions about a population based on data collected from a sample. It serves as a bridge between the data we have and the broader insights or hypotheses we want to explore about a larger group or population. Inferential statistics plays a crucial role in research, decision-making, and problem-solving across various fields.
The Purpose of Inferential Statistics
The primary purpose of inferential statistics is to provide a framework for making informed judgments about a population by analyzing a representative subset of that population—known as a sample. This framework allows us to:
- Make Predictions: Inferential statistics helps us predict or estimate population parameters (e.g., population mean, proportion) based on sample data. For example, we can estimate the average income of all households in a country using income data from a sample of households.
- Test Hypotheses: It enables us to test hypotheses and make decisions about whether observed differences or relationships in the data are statistically significant or merely due to chance. For instance, based on clinical trial data, we can assess whether a new drug is more effective than an existing one.
- Draw Generalizations: Inferential statistics helps us draw generalizations about a population based on sample characteristics. This is essential when collecting data from an entire population is impractical or impossible. For instance, election polls use samples to predict the voting behavior of an entire electorate.
In essence, inferential statistics provides the tools and techniques to make sense of data and reach meaningful conclusions while accounting for uncertainty and variability.
Key Concepts in Inferential Statistics
In inferential statistics, several key concepts form the foundation for making accurate inferences and valid conclusions:
- Population: The entire group or collection of individuals, items, or data points that are of interest in a study. It represents the broader scope for which inferences are to be made.
- Sample: A subset of the population selected for data collection and analysis. The sample should be representative of the population to ensure the validity of inferences.
- Parameter: A numerical characteristic or summary measure that describes a population. Common parameters include the population mean, variance, and proportion.
- Statistic: A numerical characteristic or summary measure that describes a sample. Statistics are calculated from sample data and are used to estimate population parameters.
- Sampling Distribution: The distribution of a statistic (e.g., sample mean) over repeated random samples from the same population. It provides insights into the variability of sample statistics.
- Hypothesis Testing: A systematic procedure used to assess whether there is enough evidence in the data to support a particular hypothesis or claim about a population.
- Confidence Intervals: A range of values constructed around a sample statistic that is likely to contain the true population parameter with a specified level of confidence.
- Margin of Error: The margin by which a point estimate (e.g., sample mean) can deviate from the true population parameter while still maintaining a specified level of confidence.
- P-Value: A probability that measures the strength of evidence against a null hypothesis in hypothesis testing. Smaller p-values indicate stronger evidence against the null hypothesis.
These key concepts form the framework for conducting inferential statistics, allowing us to make reasoned and data-driven decisions about populations based on the information contained within samples. Understanding these concepts is fundamental to conducting valid and meaningful inferential analyses.
Descriptive vs. Inferential Statistics
When it comes to statistics, two fundamental branches emerge: descriptive statistics and inferential statistics. These two approaches serve distinct purposes in the realm of data analysis, providing valuable insights into different aspects of your data.
Descriptive Statistics
Descriptive statistics are your go-to tool for summarizing and presenting data in a clear and meaningful way. They help you make sense of a dataset by condensing it into a few key measures and visuals.
Use Cases:
- Descriptive statistics are ideal for getting an initial understanding of your data. They allow you to create visualizations and summaries that provide insights into your dataset's characteristics.
- These statistics are often used for reporting and communication, as they simplify complex data into easily understandable formats.
Inferential Statistics
Inferential statistics, on the other hand, go beyond mere data description. They are all about making predictions, drawing conclusions, and testing hypotheses based on sample data.
Key Features:
- Hypothesis Testing: Inferential statistics involve hypothesis tests, where you assess whether observed differences or relationships in your sample are likely to exist in the broader population.
- Sampling: They consider the concept of sampling distributions, allowing you to infer population parameters from sample statistics.
- Probability: Inferential statistics heavily rely on probability theory to quantify uncertainty and make informed decisions.
Use Cases:
- Inferential statistics are crucial when you want to generalize your findings from a sample to an entire population. For example, in medical trials, you may use inferential statistics to determine if a new treatment is effective for a larger patient population.
- They are used to test hypotheses about cause-and-effect relationships, make predictions, and assess the significance of observed patterns in your data.
The Interplay
In practice, descriptive and inferential statistics often work hand in hand. Descriptive statistics lay the groundwork by helping you understand your data's basic characteristics. Once you have that understanding, inferential statistics step in to help you make informed decisions, test hypotheses, and draw broader conclusions about populations.
Descriptive statistics provide the "what" and "how" of your data, while inferential statistics dive into the "why" and "what's next." Both are indispensable tools in the statistician's toolkit, offering complementary insights to unlock the full potential of your data analysis.
Probability Distributions
Probability distributions lie at the heart of inferential statistics, guiding our understanding of how data is spread out and helping us make informed decisions based on that data. We'll explore two fundamental probability distributions: the Normal Distribution and Sampling Distributions. These distributions are foundational in inferential statistics, providing the framework for various statistical analyses and hypothesis testing.
The Normal Distribution
The normal distribution, also known as the Gaussian distribution or the bell curve, is a foundational concept in inferential statistics. Understanding the normal distribution is crucial because many real-world phenomena follow this pattern, making it a fundamental tool for statistical analysis.
Key Characteristics of the Normal Distribution
The normal distribution is characterized by several essential features:
- Symmetry: The curve is perfectly symmetrical around the mean, which is the center of the distribution.
- Bell-shaped: The curve forms a bell shape, with a peak at the mean and tails that extend indefinitely in both directions.
- Mean and Standard Deviation: The mean (μ) determines the center of the distribution, while the standard deviation (σ) controls the spread or width of the curve. Smaller standard deviations result in narrower, taller curves, while larger standard deviations lead to wider, flatter curves.
Z-Scores and Standardization
Z-scores, also known as standard scores, are a way to standardize values from different normal distributions, allowing for easy comparison. To calculate the Z-score for a given data point (X) in a normal distribution:
Z = (X - μ) / σ
Where:
- Z is the Z-score.
- X is the data point.
- μ is the mean of the distribution.
- σ is the standard deviation of the distribution.
A Z-score tells you how many standard deviations a particular data point is from the mean. A positive Z-score indicates that the data point is above the mean, while a negative Z-score indicates it's below the mean.
Practical Use of the Normal Distribution
The normal distribution is used in various real-world scenarios:
- Quality Control: It helps analyze manufacturing processes and product quality by examining the distribution of measurements.
- Education: In standardized testing, such as the SAT or GRE, scores are often assumed to follow a normal distribution.
- Economics: Many economic indicators, like income or stock returns, approximately follow a normal distribution.
- Biological Measurements: Traits like height and weight in a population often exhibit a normal distribution.
Understanding the normal distribution and how to work with it is a fundamental skill in inferential statistics.
Sampling Distributions
Sampling distributions are a cornerstone of inferential statistics because they provide insights into how sample statistics behave when repeatedly drawn from a population. This knowledge is essential for making inferences about population parameters.
The Concept of Sampling Distribution
A sampling distribution is the distribution of a statistic, such as the sample mean or sample proportion, calculated from multiple random samples of the same size from a population. It's crucial to distinguish between the population and the sampling distribution:
- Population: This refers to the entire group of individuals or elements you want to study.
- Sampling Distribution: It represents the distribution of a statistic (e.g., sample mean) calculated from multiple random samples of the same size from the population.
Central Limit Theorem (CLT)
The Central Limit Theorem (CLT) is a fundamental concept in inferential statistics that plays a vital role in understanding sampling distributions. It states:
"As the sample size increases, the sampling distribution of the sample mean approaches a normal distribution, regardless of the population's distribution."
- The CLT applies to the distribution of sample means, not the distribution of individual data points.
- It's a powerful concept because it allows us to make inferences about population means, even when we don't know the population's distribution.
Practical Implications of the CLT
The CLT has significant practical implications in inferential statistics:
- Estimation: When estimating population parameters, such as the population mean, the sample mean becomes a good estimator, especially for large sample sizes.
- Hypothesis Testing: The CLT underpins many hypothesis tests, enabling us to use the normal distribution for test statistics.
- Confidence Intervals: The CLT supports the construction of confidence intervals, providing a range of values within which we can reasonably expect the population parameter to lie.
- Sample Size Determination: It helps determine the minimum sample size needed for reliable inferential analysis.
Understanding the Central Limit Theorem and the concept of sampling distributions empowers you to make robust statistical inferences based on sample data, even when dealing with populations of unknown distribution.
Estimation
In inferential statistics, estimation is the art of using sample data to gain insights into population parameters and make educated guesses. It allows us to go beyond mere data collection and venture into informed decision-making.
Point Estimation
Point estimation is a critical concept in inferential statistics, allowing you to make educated guesses about population parameters based on sample data. Instead of providing a range of values like confidence intervals, point estimation provides a single value, or point estimate, as the best guess for the population parameter.
The Role of Point Estimation
Point estimation serves as the foundation for inferential statistics. It involves using sample statistics to estimate population parameters. The sample mean (x̄) is the most common point estimate for estimating the population mean (μ).
Example: Suppose you want to estimate the average time customers spend on your website. You take a random sample of 100 visitors and find that the sample mean time spent is 5 minutes. In this case, 5 minutes serves as the point estimate for the population mean.
Properties of Good Point Estimates
A good point estimate should possess the following properties:
- Unbiasedness: The expected value of the point estimate should equal the true population parameter. In other words, on average, the estimate should be correct.
- Efficiency: The estimate should have minimal variability or spread. An efficient estimate has a smaller mean squared error (MSE).
- Consistency: As the sample size increases, the point estimate should converge to the true population parameter.
- Sufficiency: The point estimate should contain sufficient information to make accurate inferences.
Point estimation provides a single value summarizing your data, making it a valuable tool for decision-making and hypothesis testing.
Confidence Intervals
Confidence intervals provide a range of values within which you can reasonably expect the population parameter to fall. They offer a more comprehensive view than point estimates, as they account for the inherent uncertainty in estimation.
Building Confidence Intervals
Constructing a confidence interval involves two main components:
- Point Estimate: You start with a point estimate (such as the sample mean) as the center of the interval.
- Margin of Error: The margin of error quantifies the uncertainty associated with the point estimate. It depends on the desired confidence level and the standard error of the statistic.
Interpreting Confidence Intervals
When you construct a confidence interval, it's typically associated with a confidence level, often expressed as a percentage (e.g., 95% confidence interval). This means that if you were to take many samples and construct intervals in the same way, approximately 95% of those intervals would contain the true population parameter.
Example: Let's say you calculate a 95% confidence interval for the average weight of a certain species of fish as 200 grams ± 10 grams. This means you are 95% confident that the true average weight of this fish population falls within the range of 190 grams to 210 grams.
Practical Use of Confidence Intervals
Confidence intervals have numerous practical applications:
- Market Research: Estimating average customer satisfaction scores with a known degree of confidence.
- Healthcare: Predicting the range of values for a patient's blood pressure based on a sample.
- Finance: Estimating the future returns on investments.
Confidence intervals provide a more informative and robust way to estimate population parameters compared to point estimates alone.
Margin of Error
The margin of error is a crucial concept tied closely to confidence intervals. It quantifies the uncertainty associated with a point estimate. Understanding the margin of error is essential for interpreting the reliability of an estimate.
Factors Affecting the Margin of Error
The margin of error depends on several key factors:
- Sample Size (n): A larger sample size reduces the margin of error. With more data points, you have more information to estimate the population parameter accurately.
- Standard Deviation (σ): A higher standard deviation in the population increases the margin of error. This reflects greater variability in the data.
- Confidence Level: As you aim for higher confidence levels (e.g., 99% instead of 90%), the margin of error also increases as you want a more precise estimate.
Interpretation of Margin of Error
The margin of error is typically presented alongside a point estimate. For example, if you have a sample mean of 50 with a margin of error of 5, you would express this as "50 ± 5." This means you are confident that the true population parameter falls within the range of 45 to 55.
Understanding the margin of error helps you assess the reliability and precision of your estimates. A smaller margin of error indicates a more precise estimate, while a larger one suggests more uncertainty.
Sample Size Determination
Determining the appropriate sample size is a critical step in the process of data collection for inferential statistics. The sample size directly impacts the accuracy and reliability of your estimates and hypothesis tests.
Factors Affecting Sample Size
Several factors influence the required sample size:
- Desired Margin of Error (E): A smaller margin of error requires a larger sample size to achieve the same level of confidence.
- Confidence Level (1 - α): Higher confidence levels, such as 99% instead of 95%, demand larger sample sizes.
- Population Variability (σ): Greater variability in the population data necessitates larger samples to achieve the same level of precision.
Sample Size Calculation
To determine the required sample size for a desired margin of error (E) at a specific confidence level (1 - α), you can use the following formula:
n = [(Z^2 * σ^2) / E^2]
Where:
- n is the required sample size.
- Z is the critical value from the appropriate statistical distribution for the desired confidence level.
- σ is the population standard deviation (or an estimate if unknown).
- E is the desired margin of error.
Calculating the sample size ensures that your study has the necessary statistical power to make accurate inferences and achieve the desired level of confidence in your results.
Hypothesis Testing
Hypothesis testing is the compass that guides us through the wilderness of uncertainty, allowing us to uncover hidden truths about populations using sample data. It's not just about crunching numbers; it's a structured process of inquiry and decision-making that plays a pivotal role in inferential statistics.
What is Hypothesis Testing?
Hypothesis testing is a fundamental process in inferential statistics that allows you to draw conclusions about a population based on sample data. It's a structured method for making decisions, evaluating claims, and testing assumptions using statistical evidence.
The Purpose of Hypothesis Testing
The primary goal of hypothesis testing is to assess whether a claim or hypothesis about a population parameter is supported by the available data. It involves the following key steps:
- Formulate Hypotheses: Begin by defining a null hypothesis (H0) representing the status quo or no effect and an alternative hypothesis (Ha) representing the effect you want to test.
- Collect Data: Gather relevant sample data from the population of interest.
- Analyze Data: Use statistical methods to evaluate the data and calculate test statistics and p-values.
- Make a Decision: Based on the evidence from the data, decide whether to reject the null hypothesis in favor of the alternative hypothesis.
Key Concepts in Hypothesis Testing
Before diving into the specific aspects of hypothesis testing, it's crucial to understand the following essential concepts:
- Test Statistic: A numerical value calculated from sample data used to assess the evidence against the null hypothesis.
- P-Value: A probability that measures the strength of evidence against the null hypothesis. A smaller p-value suggests stronger evidence against H0.
- Significance Level (α): The predetermined threshold that determines when you should reject the null hypothesis. Common choices include 0.05 and 0.01.
Hypothesis testing is employed across various fields, from medical research to marketing, to determine the validity of claims and inform decision-making.
Null and Alternative Hypotheses
In hypothesis testing, you start by defining two competing hypotheses: the null hypothesis (H0) and the alternative hypothesis (Ha). These hypotheses represent opposing viewpoints regarding the population parameter being studied.
Null Hypothesis (H0)
The null hypothesis represents the default or status quo assumption. It states that there is no effect, no difference, or no change in the population parameter. It is often symbolized as H0 and is what you aim to test against.
Example: If you are testing a new drug's effectiveness, the null hypothesis might state that the drug has no effect compared to a placebo.
Alternative Hypothesis (Ha)
The alternative hypothesis represents the claim or effect you want to test. It states that there is a significant difference, effect, or change in the population parameter. It is symbolized as Ha.
Example: In the drug effectiveness study, the alternative hypothesis would state that the new drug has a significant effect compared to a placebo.
Making a Decision
The outcome of a hypothesis test depends on the evidence provided by the sample data. If the evidence strongly supports the alternative hypothesis, you may reject the null hypothesis. If the evidence is insufficient, you fail to reject the null hypothesis.
Understanding the null and alternative hypotheses is crucial because they frame the entire hypothesis testing process, guiding your analysis and decision-making.
Significance Level and P-Values
In hypothesis testing, the significance level (α) and p-values play pivotal roles in determining whether to reject the null hypothesis. They help define the criteria for making informed decisions based on the evidence from the sample data.
Significance Level (α)
The significance level, often denoted as α, represents the threshold at which you are willing to make a Type I error (incorrectly rejecting a true null hypothesis). Commonly used significance levels include 0.05 (5%) and 0.01 (1%).
- A smaller α indicates a lower tolerance for Type I errors, making the test more stringent.
- A larger α increases the chance of making a Type I error, making the test less stringent.
P-Values
The p-value is a measure of the strength of evidence against the null hypothesis. It quantifies the probability of observing a test statistic as extreme as, or more extreme than, what you obtained from the sample data, assuming that the null hypothesis is true.
- A small p-value (typically less than α) suggests strong evidence against the null hypothesis, leading to its rejection.
- A large p-value implies weak evidence against the null hypothesis, supporting its retention.
Interpreting P-Values
- If p-value ≤ α: You have sufficient evidence to reject the null hypothesis in favor of the alternative hypothesis.
- If p-value > α: You lack sufficient evidence to reject the null hypothesis, and you fail to provide support for the alternative hypothesis.
The choice of significance level α is a trade-off between the risk of making Type I errors and the risk of making Type II errors (incorrectly failing to reject a false null hypothesis).
Type I and Type II Errors
Hypothesis testing involves the possibility of two types of errors: Type I and Type II errors. Understanding these errors is essential for assessing the potential risks associated with hypothesis testing.
Type I Error (False Positive)
A Type I error occurs when you incorrectly reject a true null hypothesis. In other words, you conclude that there is an effect or difference when none exists. The probability of committing a Type I error is equal to the chosen significance level (α).
Type II Error (False Negative)
A Type II error occurs when you incorrectly fail to reject a false null hypothesis. In this case, you conclude that there is no effect or difference when one actually exists. The probability of making a Type II error is denoted as β.
Balancing Type I and Type II Errors
The choice of significance level (α) and sample size directly impacts the likelihood of Type I and Type II errors. A lower α reduces the chance of Type I errors but increases the risk of Type II errors, and vice versa.
Balancing these errors is a crucial consideration when designing hypothesis tests, as the relative importance of these errors varies depending on the context and consequences of the decision.
Hypothesis Tests for Means and Proportions
Hypothesis testing can be applied to a wide range of population parameters, but two common scenarios involve testing means and proportions.
Hypothesis Testing for Means
When you want to compare the mean of a sample to a known or hypothesized population mean, you use hypothesis testing for means. This often involves the use of t-tests or Z-tests, depending on sample size and available information about the population standard deviation.
Example: Testing whether the average IQ of students in a school is different from the national average.
Hypothesis Testing for Proportions
In situations where you want to assess the proportion of a sample that possesses a specific attribute or trait, you employ hypothesis testing for proportions. This typically involves using a z-test for proportions.
Example: Determining whether the proportion of customers who prefer product A over product B significantly differs from a predetermined value.
These specialized hypothesis tests enable you to make specific inferences about means and proportions, helping you draw meaningful conclusions based on sample data.
Parametric Tests
Parametric tests are statistical methods used in hypothesis testing when certain assumptions about the population distribution are met. These tests are powerful tools for comparing means, variances, and proportions across different groups or conditions. We'll delve into three essential parametric tests: t-tests, Analysis of Variance (ANOVA), and Chi-Square tests.
t-Tests
t-Tests are widely used for comparing the means of two groups or conditions. There are three main types of t-tests:
- Independent Samples t-Test: This test compares the means of two independent groups or samples. It helps determine whether the means are significantly different from each other.
Example: Comparing the test scores of students who received a new teaching method versus those who received traditional instruction. - Paired Samples t-Test: Also known as a dependent t-test, this test compares the means of two related groups, such as before and after measurements for the same individuals.
Example: Assessing whether a weight loss program results in a significant reduction in participants' weight. - One-Sample t-Test: This test compares the mean of a sample to a known population mean or a hypothesized value. It helps determine if the sample mean is significantly different from the expected value.
Example: Testing if the average wait time at a restaurant is significantly different from 15 minutes, as advertised.
Analysis of Variance (ANOVA)
Analysis of Variance (ANOVA) is used when you need to compare the means of more than two groups or conditions. ANOVA assesses whether there are significant differences between the group means and helps identify which groups differ from each other.
There are various types of ANOVA, including:
- One-Way ANOVA: Used when you have one categorical independent variable (factor) with more than two levels or groups.
Example: Comparing the average incomes of people from different professions (e.g., doctors, lawyers, engineers).
- Two-Way ANOVA: Involves two independent categorical variables (factors) and allows you to assess their individual and interactive effects on the dependent variable.
Example: Analyzing the impact of both gender and education level on income. - Repeated Measures ANOVA: Similar to one-way ANOVA, but for dependent (repeated) measurements within the same subjects over time or conditions.
Example: Evaluating the effects of a drug treatment at different time points for the same individuals.
Chi-Square Tests
Chi-Square tests are used to assess the association between categorical variables. These tests help determine whether there is a significant relationship between two or more categorical variables.
There are two main types of Chi-Square tests:
- Chi-Square Test for Independence: This test examines whether two categorical variables are independent of each other or if there is an association between them.
Example: Investigating whether there is an association between smoking habits (smoker or non-smoker) and the development of a specific disease (yes or no). - Chi-Square Goodness-of-Fit Test: Used to compare observed categorical data with expected data to check if they follow the same distribution.
Example: Determining whether the distribution of blood types in a population follows the expected distribution based on genetic frequencies.
Parametric tests like t-tests, ANOVA, and Chi-Square tests are valuable tools for hypothesis testing when certain assumptions about the data distribution are met. They allow you to make informed decisions and draw meaningful conclusions in various research and analytical contexts.
Nonparametric Tests
Nonparametric tests, also known as distribution-free tests, are a class of statistical methods used when the assumptions of parametric tests (such as normality and homogeneity of variances) are not met, or when dealing with data that do not follow a specific distribution. We will explore several nonparametric tests that are valuable tools for hypothesis testing and data analysis.
What are Nonparametric Tests?
Nonparametric tests are a versatile alternative to parametric tests and are especially useful when:
- The data do not meet the assumptions of normality or homogeneity of variances.
- The data are measured on an ordinal or nominal scale.
- The sample size is small.
Nonparametric tests make fewer assumptions about the data distribution and are, therefore, robust in various situations. They are often used in fields like psychology, social sciences, and medicine.
Mann-Whitney U Test
The Mann-Whitney U Test, also known as the Wilcoxon rank-sum test, is used to compare the distributions of two independent samples to determine if one sample tends to have higher values than the other. It does not assume that the data are normally distributed.
Example: Comparing the exam scores of two different groups of students (e.g., students who received tutoring vs. those who did not) to see if there is a significant difference in performance.
Wilcoxon Signed-Rank Test
The Wilcoxon Signed-Rank Test compares the distribution of paired (dependent) data or matched samples. It assesses whether there is a significant difference between two related groups.
Example: Analyzing whether there is a significant change in blood pressure before and after a new medication within the same group of patients.
Kruskal-Wallis Test
The Kruskal-Wallis Test is a nonparametric alternative to one-way ANOVA, used when comparing the means of three or more independent groups or conditions. It assesses whether there are significant differences between the groups.
Example: Comparing the effectiveness of three different treatments for pain relief in patients with the same medical condition.
Chi-Square Test of Independence
The Chi-Square Test of Independence is used to assess whether there is a significant association between two categorical variables. It helps determine if the variables are independent or if there is a relationship between them.
Example: Investigating whether there is a relationship between gender (male or female) and voting preference (candidate A, candidate B, or undecided) in a political survey.
Nonparametric tests are valuable tools in situations where parametric assumptions cannot be met or when dealing with categorical data. They provide robust alternatives for hypothesis testing, allowing researchers and analysts to draw meaningful conclusions from their data.
Regression Analysis
Regression analysis is a powerful statistical method used to model the relationship between one or more independent variables (predictors) and a dependent variable (outcome). It helps us understand how changes in the predictors relate to changes in the outcome, enabling us to make predictions and draw insights from data.
The three fundamental types of regression analysis are Simple Linear Regression, Multiple Linear Regression, and Logistic Regression.
Simple Linear Regression
Simple Linear Regression is the most basic form of regression analysis and is used when there is a single independent variable (predictor) and a single dependent variable (outcome). It models the linear relationship between these two variables using a straight line.
Key Components:
- Dependent Variable (Y): The variable we want to predict or explain.
- Independent Variable (X): The variable that serves as the predictor.
- Regression Equation: In simple linear regression, the relationship is expressed as a linear equation: Y = α + βX + ε, where α represents the intercept, β is the slope, and ε is the error term.
Use Cases: Simple Linear Regression is applied in scenarios where we want to understand the linear relationship between two variables, such as predicting sales based on advertising spending or estimating the impact of years of education on income.
Multiple Linear Regression
Multiple Linear Regression extends simple linear regression to situations where there are numerous independent variables (predictors) influencing a single dependent variable (outcome). It allows us to model complex relationships and account for multiple factors simultaneously.
Key Components:
- Dependent Variable (Y): The variable we want to predict or explain.
- Multiple Independent Variables (X1, X2, ... Xn): Several predictors affecting the outcome.
- Regression Equation: The relationship is expressed as: Y = α + β1X1 + β2X2 + ... + βnXn + ε, where α represents the intercept, β1, β2, ... βn are the slopes, and ε is the error term.
Use Cases: Multiple Linear Regression is applied in scenarios where multiple factors can influence an outcome, such as predicting a house's price based on features like square footage, number of bedrooms, and neighborhood.
Logistic Regression
Logistic Regression is used when the dependent variable is binary (two possible outcomes, usually 0 and 1), and the relationship between the independent variables and the outcome needs to be modeled. Instead of predicting a continuous value, logistic regression models the probability of an event occurring.
Key Components:
- Dependent Variable (Y): Binary outcome (0 or 1).
- Independent Variables (X1, X2, ... Xn): Predictors that influence the probability of the event.
- Logistic Function: The relationship is modeled using the logistic function, which transforms linear combinations of predictors into probabilities. The logistic regression equation is: P(Y=1) = 1 / (1 + e^-(α + β1X1 + β2X2 + ... + βnXn)), where P(Y=1) is the probability of the event occurring.
Use Cases: Logistic Regression is commonly used in scenarios such as predicting whether a customer will churn (leave) a subscription service based on factors like customer age, usage patterns, and customer service interactions.
ANOVA and Experimental Design
Analysis of Variance (ANOVA) is a powerful statistical technique used to analyze the differences among group means in experimental and research settings. It allows researchers to assess whether variations in a dependent variable can be attributed to differences in one or more independent variables.
One-Way ANOVA
One-Way ANOVA, also known as single-factor ANOVA, is used when there is one categorical independent variable (factor) with more than two levels or groups. It assesses whether there are significant differences in the means of these groups.
Key Components:
- Dependent Variable (Y): The variable we want to analyze or compare among groups.
- One Categorical Independent Variable (Factor): The grouping variable with more than two levels.
- Null Hypothesis (H0): Assumes that all group means are equal.
- Alternative Hypothesis (Ha): Suggests that at least one group mean differs from the others.
Use Cases: One-Way ANOVA is applied in scenarios where you want to determine if there are significant differences among multiple groups, such as comparing the effectiveness of three different teaching methods on student test scores.
Two-Way ANOVA
Two-Way ANOVA extends the concept of One-Way ANOVA to situations where there are two independent categorical variables (factors) affecting a single dependent variable. It evaluates the main effects of each factor and their interaction.
Key Components:
- Dependent Variable (Y): The variable under investigation.
- Two Independent Variables (Factor 1 and Factor 2): Categorical variables, each with multiple levels.
- Main Effects: The impact of each factor on the dependent variable.
- Interaction Effect: The combined effect of both factors on the dependent variable.
Use Cases: Two-Way ANOVA is employed when you need to assess the effects of two independent variables simultaneously, such as studying how both gender and age affect the performance of students on an exam.
Factorial Designs
Factorial Designs are experimental designs that involve manipulating and studying multiple factors simultaneously to understand their individual and interactive effects on the dependent variable. These designs can include One-Way or Two-Way ANOVA, but they expand to more complex scenarios.
Key Concepts:
- Factorial Notation: A factorial design is typically represented as A × B, where A and B are the two factors under investigation.
- Main Effects: Assess the impact of each factor on the dependent variable, considering all other factors.
- Interaction Effects: Examine how the combined influence of two or more factors differs from what would be expected by considering each factor separately.
- Factorial Matrix: A table that displays all the combinations of factor levels and allows researchers to perform multiple comparisons.
Use Cases: Factorial designs are used when you want to study the joint effects of multiple factors on an outcome. For example, in psychology, a factorial design could examine how both the type of therapy and the frequency of therapy sessions affect patients' mental health outcomes.
ANOVA and experimental design are essential tools in research and experimentation, allowing researchers to explore the impact of various factors and make informed conclusions about the factors' effects on a dependent variable. These techniques find wide applications in fields such as psychology, biology, engineering, and social sciences.
Statistical Software and Tools
Statistical software and tools play a pivotal role in modern data analysis and research. They facilitate data collection, manipulation, visualization, and statistical analysis, making it easier for researchers and analysts to derive valuable insights from data.
What is Statistical Software?
Statistical software refers to specialized computer programs designed to handle statistical analysis, modeling, and data visualization. These software applications are essential for researchers, analysts, and data scientists working with data of varying complexities. Statistical software can automate complex calculations, generate visualizations, and perform hypothesis testing with ease.
Key Features:
- Data Import and Management: Statistical software allows users to import, clean, and manipulate datasets efficiently.
- Statistical Analysis: These tools provide a wide range of statistical tests and methods, from basic descriptive statistics to advanced modeling techniques.
- Data Visualization: Statistical software often includes powerful visualization tools to help users effectively explore and communicate their findings.
- Automation: Many tasks, such as generating reports or conducting regression analysis, can be automated to save time and reduce errors.
Using Software for Data Collection
Statistical software is not limited to analysis alone; it is also invaluable in the data collection process. Researchers can use software to design and administer surveys, questionnaires, experiments, and data collection forms. This streamlines the data collection process and helps ensure data accuracy.
- Survey Design: Statistical software allows users to create structured surveys and questionnaires, define response formats, and generate online or paper-based surveys.
- Data Entry: Collected data can be efficiently entered into the software, reducing manual errors.
- Data Validation: Software can check data for errors, inconsistencies, or missing values, improving data quality.
Using Software for Data Analysis
Statistical software provides a comprehensive suite of tools for data analysis. It allows users to explore data, perform hypothesis tests, build predictive models, and quickly generate reports. Some popular data analysis tasks include:
- Descriptive Statistics: Summarizing data through measures like mean, median, and standard deviation.
- Inferential Statistics: Conducting hypothesis tests, confidence intervals, and regression analysis.
- Data Visualization: Creating charts, graphs, and plots to visualize patterns and relationships in data.
- Predictive Modeling: Building predictive models using techniques like linear regression, decision trees, or machine learning algorithms.
Commonly Used Statistical Software
Several statistical software packages are widely used in research, academia, and industry. Some of the most popular options include:
R
R is a free, open-source statistical software and programming language known for its extensive library of statistical packages and data visualization capabilities. It is highly customizable and widely used in data analysis and research.
Python (with libraries like NumPy, Pandas, and SciPy)
Python is a versatile programming language with extensive libraries that include powerful tools for data analysis and statistical modeling. NumPy and Pandas provide data manipulation capabilities, while SciPy offers statistical functions.
SPSS (Statistical Package for the Social Sciences)
SPSS is a user-friendly statistical software often preferred by social scientists and researchers. It offers a graphical interface and a wide range of statistical tests.
SAS (Statistical Analysis System)
SAS is a comprehensive statistical software used in various industries for data analysis, predictive modeling, and statistical reporting. It is known for its robustness and scalability.
Excel
Microsoft Excel is a widely accessible spreadsheet software that includes basic statistical functions and tools for data analysis. It is commonly used for simple analyses and data visualization.
Statistical software and tools empower researchers and analysts to harness the full potential of data by enabling efficient data collection, analysis, and visualization. The choice of software depends on factors such as the project's specific needs, the user's familiarity with the tool, and the complexity of the analysis.
Conclusion for Inferential Statistics
Inferential statistics is your gateway to understanding the bigger picture from a limited set of data. It enables us to predict, test, and generalize with confidence, making informed decisions and uncovering hidden insights. With the knowledge gained from this guide, you now possess a valuable skill set to explore, analyze, and interpret data effectively. Remember, the power of inferential statistics lies in its ability to transform small samples into meaningful conclusions, empowering you in various academic, research, and real-world scenarios.
As you embark on your statistical journey, keep practicing and exploring the diverse applications of inferential statistics.
How to Collect Real-Time Insights for Inferential Statistics?
In inferential statistics, the ability to collect data swiftly and effectively can make all the difference. Imagine having the power to conduct your market research in just minutes. That's where Appinio, the real-time market research platform, steps in.
- Swift Insights: From formulating questions to obtaining actionable insights, Appinio streamlines the entire research process. With an average field time of less than 23 minutes for 1,000 respondents, you'll have the data you need in no time.
- User-Friendly: No need for a PhD in research. Appinio's intuitive platform empowers anyone to conduct market research with ease. You focus on your business goals, while Appinio takes care of the heavy lifting.
- Global Reach: With access to over 90 countries and the ability to define the right target group from 1200+ characteristics, you can tailor your research to fit your specific needs.
Join the loop 💌
Be the first to hear about new updates, product news, and data insights. We'll send it all straight to your inbox.