How to Calculate Statistical Significance? (+ Examples)
Appinio Research · 05.12.2023 · 39min read
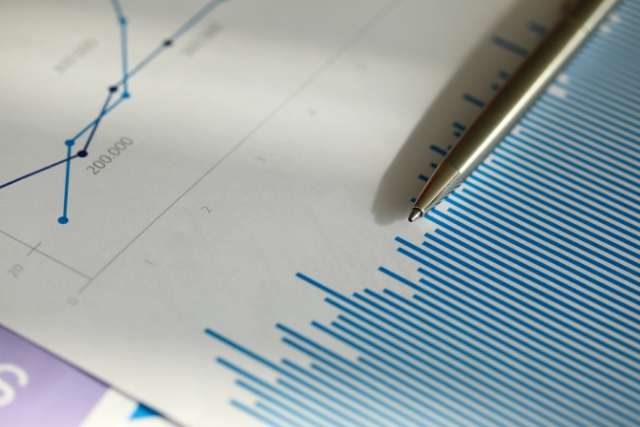
Content
Have you ever wondered how to distinguish between mere chance and genuine insights when analyzing data? Statistical significance holds the key to unlocking the true importance of your findings.
In this guide, we will delve deep into statistical significance, uncovering its definition, importance, practical applications, advanced concepts, and the art of effectively communicating your results. Whether you're a researcher, data analyst, or decision-maker, understanding statistical significance is a vital skill for making informed choices and drawing meaningful conclusions from data.
What is Statistical Significance?
Statistical significance is a critical concept in data analysis and research that helps determine whether the observed results are likely due to a real effect or merely the result of chance variation. It quantifies the likelihood that an observed difference or relationship in data is not a random occurrence.
Statistical significance is typically expressed in terms of p-values or confidence intervals, allowing researchers to make informed decisions based on data.
The Importance of Statistical Significance
Statistical significance serves several essential purposes:
- Validating Hypotheses: It helps researchers assess whether the findings support or contradict their hypotheses, enabling them to draw meaningful conclusions.
- Informed Decision-Making: It provides a basis for decision-making in various fields, from healthcare to business, by distinguishing between genuine effects and random fluctuations.
- Reducing Uncertainty: Statistical significance reduces uncertainty in research and data-driven decision-making, enhancing the reliability of results.
- Scientific Discovery: In scientific research, it guides scientists in identifying and investigating relationships, trends, and phenomena.
Why Statistical Significance Matters in Data Analysis
Statistical significance is crucial in data analysis because it:
- Separates Signal from Noise: It helps differentiate between patterns or differences in data that are likely meaningful and those that may occur by chance.
- Aids in Inference: By assessing statistical significance, data analysts can make inferences about populations based on sample data.
- Supports Generalization: It enables the generalization of findings from samples to larger populations, extending the relevance of research.
- Enhances Credibility: In both scientific research and practical decision-making, statistical significance adds credibility and rigor to the analysis.
Common Statistical Significance Applications
Statistical significance is widely used across various fields and applications, including:
- Clinical Trials: Assessing the efficacy of new medical treatments.
- Market Research: Analyzing consumer behavior and preferences.
- Quality Control: Ensuring product quality and consistency.
- A/B Testing: Comparing the effectiveness of different marketing strategies.
- Social Sciences: Investigating social phenomena and behaviors.
- Environmental Studies: Assessing the impact of environmental factors on ecosystems.
Statistical significance is a versatile tool that empowers professionals and researchers to make data-driven decisions and draw reliable conclusions across diverse domains.
Fundamentals of Hypothesis Testing
Hypothesis testing is a critical aspect of statistical significance analysis, helping you determine the validity of your findings. We'll start by delving deeper into the fundamental concepts and components of hypothesis testing.
Formulating Hypotheses
Formulating clear and testable hypotheses is the first step in hypothesis testing. You start with two hypotheses: the null hypothesis (H0) and the alternative hypothesis (H1 or Ha).
Null Hypothesis and Alternative Hypothesis
The null hypothesis (H0) suggests that there is no significant difference or effect in your data. It represents the status quo or the absence of an effect. The alternative hypothesis (H1 or Ha), on the other hand, asserts that there is a significant difference or effect in your data, challenging the null hypothesis.
Significance Level (Alpha) and P-Values
The significance level, often denoted as alpha (α), plays a critical role in hypothesis testing. It determines the threshold at which you consider a result statistically significant. Commonly used significance levels are 0.05 and 0.01.
- P-Value: The p-value quantifies the strength of evidence against the null hypothesis. A lower p-value indicates stronger evidence against H0, suggesting that you should reject it in favor of the alternative hypothesis.
Type I and Type II Errors
In hypothesis testing, two types of errors can occur: Type I and Type II errors.
- Type I Error: This error occurs when you incorrectly reject a true null hypothesis. In other words, you conclude there's an effect when there isn't one.
- Type II Error: Type II errors happen when you fail to reject a false null hypothesis. In this case, you conclude there's no effect when there actually is.
Understanding these error types is crucial for making informed decisions and interpreting the results of hypothesis tests.
Power of a Statistical Test
The power of a statistical test measures its ability to correctly reject a false null hypothesis. It's influenced by several factors:
- Sample Size: A larger sample size generally increases the power of a test, making it more likely to detect true effects.
- Effect Size: A larger effect size, which represents the magnitude of the difference or effect, also enhances the power of a test.
- Significance Level (Alpha): Lowering the significance level (α) increases the chance of making a Type II error but decreases the chance of making a Type I error, affecting the test's power.
- Variability in the Data: Higher variability in the data may reduce the power of a test because it can make it harder to detect an effect.
Understanding and manipulating the power of a statistical test is crucial for designing experiments and studies that can effectively detect meaningful effects or differences.
Sampling and Data Collection
Sampling and data collection are crucial steps in the statistical significance analysis process. These steps ensure that your data is representative and free from bias, laying the foundation for reliable results.
Random Sampling
Random sampling is the process of selecting a subset of individuals or items from a larger population in a way that each member has an equal chance of being chosen. This technique helps minimize bias and ensure that your sample fairly represents the entire population.
- Simple Random Sampling: In this method, each member of the population has an equal probability of being selected. It can be accomplished using random number generators or drawing lots.
- Stratified Sampling: Stratified sampling divides the population into subgroups (strata) based on specific characteristics (e.g., age, gender). Samples are then randomly selected from each stratum to ensure representation.
- Cluster Sampling: Cluster sampling involves dividing the population into clusters and randomly selecting a few clusters for sampling. It's particularly useful when it's difficult to create a complete list of the population.
Sample Size Determination
Determining the appropriate sample size is a critical consideration in statistical significance analysis. An insufficient sample size can lead to unreliable results, while an excessively large sample may be resource-intensive without providing much additional benefit.
Factors influencing sample size determination include:
- Population Variability: Higher variability in the population typically requires a larger sample size to detect significant differences.
- Desired Confidence Level: Increasing the desired confidence level (e.g., 95% or 99%) necessitates a larger sample size.
- Margin of Error: Smaller margins of error require larger sample sizes.
- Expected Effect Size: The magnitude of the effect you want to detect influences sample size; larger effects require smaller samples.
Various statistical formulas and software tools are available to calculate sample sizes based on these factors. It's essential to strike a balance between the precision of your results and the practicality of obtaining the required sample.
Data Collection Methods
Selecting the appropriate data collection method is essential to gather accurate and relevant information. The choice of method depends on your research objectives and the nature of the data.
Popular data collection methods include:
- Surveys and Questionnaires: Surveys involve asking individuals a set of structured questions to collect data on their opinions, attitudes, or behaviors.
- Experiments: Experimental studies involve controlled interventions to examine cause-and-effect relationships. They are common in scientific research.
- Observational Studies: Observational studies involve observing and recording data without intervening. They are often used in fields like psychology and sociology.
- Secondary Data Analysis: Secondary data analysis involves using existing data sources, such as databases or publicly available datasets, to answer research questions.
Each data collection method has its strengths and limitations, and the choice should align with your research objectives and resources.
Data Preprocessing and Cleaning
Data preprocessing and cleaning are essential steps to ensure the quality and reliability of your data before conducting statistical significance tests.
Key tasks in data preprocessing and cleaning include:
- Data Validation: Check for accuracy and completeness of data. Identify and handle missing values, outliers, and errors.
- Data Transformation: Transform data as needed, such as normalizing or standardizing variables, to meet the assumptions of statistical tests.
- Data Imputation: If there are missing values, consider imputation techniques to fill in the gaps, maintaining the integrity of your dataset.
- Data Encoding: Encode categorical variables into numerical formats, as many statistical tests require numerical inputs.
- Data Scaling: Scale or normalize variables to ensure they have the same units or magnitudes, especially when working with different measurement scales.
Investing time in data preprocessing and cleaning can enhance the accuracy and reliability of your statistical analysis results, ultimately leading to more robust conclusions.
How to Calculate Statistical Significance?
Calculating statistical significance involves several key steps and depends on the type of data and hypothesis you're testing. Here, we'll provide a general overview of the process and highlight standard methods for different scenarios.
1. Formulate Your Hypotheses
Before calculating statistical significance, you need to define your null hypothesis (H0) and alternative hypothesis (H1). The null hypothesis typically represents the absence of an effect, while the alternative hypothesis states what you're trying to prove or find evidence for.
2. Choose the Appropriate Statistical Test
Select the statistical test that matches your research question and data type. Common tests include t-tests for comparing means, chi-square tests for independence, ANOVA for comparing multiple groups, and correlation tests for assessing relationships.
3. Collect and Organize Data
Collect your data in a systematic and structured manner. Ensure you have a clear plan for data collection, data entry, and data cleaning to minimize errors and biases.
4. Perform the Statistical Test
The specific steps for performing a statistical test depend on the chosen method. However, the general process involves:
- Calculating Test Statistics: Compute the test statistic (e.g., t, chi-square, F) based on your data and the chosen formula for the test.
- Determining Degrees of Freedom: Calculate the degrees of freedom associated with your test, which is critical for finding critical values from tables or statistical software.
- Finding Critical Values: Determine the critical values for your chosen significance level (alpha) from statistical tables or use statistical software to find them.
- Calculating p-Values: For many tests, calculate the p-value associated with the test statistic. The p-value represents the probability of observing the results under the null hypothesis.
5. Compare Results to Alpha Level
Compare the calculated p-value to your predetermined significance level (alpha). If the p-value is less than or equal to alpha (p ≤ α), you reject the null hypothesis in favor of the alternative hypothesis, indicating statistical significance.
6. Interpret the Results
Interpret the results in the context of your research question. If your findings are statistically significant, it suggests that the observed effect or relationship is unlikely to occur by random chance. If not significant, it implies that there's insufficient evidence to reject the null hypothesis.
7. Report the Findings
In your research report or analysis, clearly state the statistical test you used, the calculated test statistic, degrees of freedom, p-value, and whether the results were statistically significant. Additionally, provide context, effect size measures, and practical implications.
8. Use Statistical Software
Many statistical tests and calculations are complex and require specialized software like R, Python, SPSS, or Excel. These tools can automate calculations, provide critical values, and generate p-values, making the process more efficient and accurate.
Remember that the specific steps and equations vary based on the chosen statistical test. It's crucial to consult relevant statistical resources or seek assistance from a statistician when dealing with complex analyses or unfamiliar tests. Calculating statistical significance correctly ensures the validity and reliability of your research findings.
Basic Statistical Tests for Significance
In statistical significance analysis, various tests are used to assess the significance of differences or relationships within data. Here, we explore five fundamental tests: the t-Test, Chi-Square Test, ANOVA (Analysis of Variance), Z-Test, and Mann-Whitney U Test and Wilcoxon Signed-Rank Test.
t-Test
The t-Test is used to compare the means of two groups and determine if the difference between them is statistically significant. There are three main types of t-tests.
Independent Samples t-Test
Used when comparing the means of two independent groups or samples. The formula for the t-statistic is:
t = (x̄1 - x̄2) / √(s^2 / n1 + s^2 / n2)
where:
- x̄1 and x̄2 are the sample means of the two groups.
- s^2 is the pooled variance of the two groups.
- n1 and n2 are the sample sizes of the two groups.
Paired Samples t-Test
Used when comparing the means of two related groups (e.g., before and after measurements on the same subjects). The formula is similar to the independent samples t-test but accounts for the paired nature of the data.
One-Sample t-Test
Used when comparing the mean of a single sample to a known population mean. The formula is:
t = (x̄ - μ) / (s / √n)
where:
- x̄ is the sample mean.
- μ is the population mean.
- s is the sample standard deviation.
- n is the sample size.
Example: Suppose you want to determine if there is a significant difference in the test scores of two groups of students, Group A and Group B. You can use an independent samples t-test to analyze the data and calculate the t-statistic.
Chi-Square Test
The Chi-Square Test is used to assess the association between categorical variables and determine if the observed frequencies differ significantly from the expected frequencies. There are two main types of Chi-Square tests.
Chi-Square Test for Independence
Used to test the independence of two categorical variables in a contingency table. The formula for the Chi-Square statistic is:
χ² = Σ [(O - E)² / E]
where:
- O is the observed frequency.
- E is the expected frequency.
Chi-Square Goodness-of-Fit Test
Used to determine if the observed categorical data fits a specific expected distribution (e.g., a uniform distribution). The formula is similar to the Chi-Square test for independence.
Example: Imagine you have data on the preferences of two age groups (under 30 and 30 and above) for three different types of beverages (coffee, tea, and juice). You can use a Chi-Square Test for Independence to assess if there is a significant association between age group and beverage preference.
ANOVA (Analysis of Variance)
ANOVA is used when you have more than two groups to compare means and determine if there are significant differences among them. One-way ANOVA is used for a single categorical independent variable, while two-way ANOVA involves two independent variables.
The formula for the one-way ANOVA F-statistic is:
F = (MSB / MSW)
where:
- MSB is the mean square between groups (explained variance).
- MSW is the mean square within groups (unexplained variance).
Example: Suppose you have data on students' test scores from three different schools. You can use one-way ANOVA to test if there are significant differences in the mean test scores among the schools.
Z-Test
The Z-Test is similar to the t-Test but is often used when dealing with larger sample sizes or when the population standard deviation is known. It is used to compare a sample mean to a known population mean.
The formula for the Z-Test statistic is:
Z = (x̄ - μ) / (σ / √n)
where:
- x̄ is the sample mean.
- μ is the population mean.
- σ is the population standard deviation.
- n is the sample size.
Example: If you want to determine if the mean height of a sample of individuals differs significantly from the known population mean height, you can use a Z-Test.
Mann-Whitney U Test and Wilcoxon Signed-Rank Test
These non-parametric tests are used when your data doesn't meet the assumptions of parametric tests like the t-Test.
- Mann-Whitney U Test: Used to compare two independent groups or samples to assess if one group has significantly higher values than the other. It ranks all data points and calculates the U statistic.
- Wilcoxon Signed-Rank Test: Used to compare two related groups, typically when dealing with paired data. It ranks the differences between paired observations and calculates the test statistic.
Example: When you have ordinal or non-normally distributed data and want to determine if there's a significant difference between two groups, you can use either the Mann-Whitney U Test (for independent samples) or the Wilcoxon Signed-Rank Test (for paired samples).
Understanding Confidence Intervals
Confidence intervals (CIs) are essential tools in statistical significance analysis. They provide a range of values within which a population parameter is likely to fall.
What is a Confidence Interval?
A confidence interval is a range of values calculated from your sample data that likely contains the true population parameter with a specified level of confidence. It quantifies the uncertainty associated with estimating a population parameter from a sample.
The formula for calculating a confidence interval for the population mean (μ) using a t-distribution is:
CI = x̄ ± (t * (s / √n))
where:
- CI is the confidence interval.
- x̄ is the sample mean.
- t is the critical value from the t-distribution corresponding to your chosen confidence level and degrees of freedom.
- s is the sample standard deviation.
- n is the sample size.
How to Calculate Confidence Intervals?
To calculate a confidence interval:
- Choose a confidence level (e.g., 95% or 99%) and determine the corresponding critical value from the t-distribution table or use statistical software.
- Calculate the sample mean (x̄) and sample standard deviation (s) from your data.
- Determine the sample size (n).
- Plug these values into the formula for the confidence interval.
How to Interpret Confidence Intervals?
Interpreting confidence intervals involves understanding that they provide a range of plausible values for the population parameter. Here are key points to consider:
- Confidence Level: If you calculate a 95% confidence interval, it means that in repeated sampling, you would expect the true population parameter to fall within the interval in 95% of cases.
- Overlap of Intervals: If you have two groups with non-overlapping confidence intervals for their means, it suggests a statistically significant difference between the groups.
- Width of the Interval: A narrower confidence interval indicates a more precise estimate, while a wider interval indicates more uncertainty.
Relationship Between Confidence Intervals and Significance Testing
Confidence intervals and significance testing are closely related. In fact, the concepts of confidence intervals and hypothesis testing share similarities.
- Null Hypothesis Rejection: If a confidence interval does not include a particular value, it suggests that the null hypothesis is rejected for that value in significance testing.
- Effect Size: The width of a confidence interval provides information about the effect size. A narrow interval indicates a larger effect size, while a wide interval suggests a smaller effect.
Example: Suppose you want to estimate the average time it takes for customers to complete a specific task on your website. You collect a sample of data and calculate a 95% confidence interval, which turns out to be (12.5, 15.2) seconds. This means you are 95% confident that the true population average time falls within this interval. If a competitor claims their website's task completion time is 10 seconds, and this value is outside your confidence interval, you have evidence to reject their claim in favor of your own data.
Advanced Topics in Significance Testing
In significance testing, several advanced topics and techniques can help you navigate complex scenarios and draw more nuanced conclusions.
Multiple Comparisons Problem
When you conduct multiple hypothesis tests on the same dataset, you increase the likelihood of making Type I errors (false positives). This issue is known as the multiple comparisons problem.
Solution: To address this problem, you can employ various methods, such as the Bonferroni correction or the False Discovery Rate (FDR) correction. These methods adjust the significance level (alpha) for individual tests to control the overall familywise error rate.
Example: Imagine you're testing the effectiveness of several drug treatments on a specific condition. If you perform separate tests for each drug without adjusting for multiple comparisons, you might mistakenly conclude that some drugs are effective when, in reality, they are not.
Bonferroni Correction
The Bonferroni correction is a widely used method to control the familywise error rate in multiple comparisons. It adjusts the significance level (alpha) for individual tests to maintain an overall alpha level.
The Bonferroni-corrected alpha (α_corrected) is calculated as:
α_corrected = α / k
where:
- α_corrected is the corrected significance level.
- α is the desired overall significance level (e.g., 0.05).
- k is the number of comparisons or tests.
Example: If you are conducting 5 hypothesis tests and want to maintain an overall significance level of 0.05, the Bonferroni-corrected significance level for each test would be 0.05 / 5 = 0.01.
Effect Size and Practical Significance
While statistical significance tells you if an effect exists, effect size measures the magnitude of that effect. Practical significance, on the other hand, considers whether the effect is meaningful in a real-world context.
Effect Size Metrics: Common effect size metrics include Cohen's d for comparing means, odds ratios for binary data, and correlation coefficients for relationships between variables.
Example: If a new drug reduces blood pressure by 1 mmHg, it may be statistically significant with a large sample size, but it might not be practically substantial for clinical purposes.
Non-Parametric Tests
Non-parametric tests are used when your data doesn't meet the assumptions of parametric tests, such as normal distribution or homogeneity of variances.
Non-parametric tests include:
- Mann-Whitney U Test: Used for comparing two independent groups when the assumptions for the t-test are not met.
- Wilcoxon Signed-Rank Test: Used for comparing two related groups or paired samples when assumptions for the t-test are violated.
- Kruskal-Wallis Test: An analog of one-way ANOVA for comparing more than two independent groups with non-normally distributed data.
- Chi-Square Test of Independence: Used for testing the independence of categorical variables when parametric assumptions are not met.
Example: Non-parametric tests are valuable in scenarios where data distributional assumptions are not met, such as when dealing with ordinal or skewed data.
Understanding and applying these advanced topics in significance testing can significantly enhance the quality and reliability of your statistical analyses, especially in complex research or decision-making contexts.
Common Statistical Significance Mistakes and Pitfalls
Avoiding common errors and pitfalls in significance testing is crucial for obtaining accurate and meaningful results.
Misinterpreting P-Values
One of the most common mistakes in significance testing is misinterpreting p-values. A p-value represents the probability of observing a result as extreme as, or more extreme than, the one obtained under the null hypothesis. Common pitfalls include:
- P-Hacking: Repeatedly testing multiple hypotheses until a significant result is found, increasing the risk of Type I errors.
- Overemphasis on Small P-Values: Assuming that a small p-value (e.g., p < 0.05) implies a strong practical or scientific effect.
Mitigation: Understand that p-values alone do not indicate the size or importance of an effect. Always consider effect size, confidence intervals, and practical significance alongside p-values.
Not Considering Sample Size
Sample size plays a critical role in the reliability of your results. Insufficient sample sizes can lead to underpowered tests, making it challenging to detect real effects. Common pitfalls include:
- Ignoring Power Analysis: Failing to perform power analysis to determine the required sample size before conducting a study.
- Drawing Conclusions from Small Samples: Making solid claims based on small samples, which can lead to spurious results.
Mitigation: Conduct power analysis to determine the appropriate sample size for your study and avoid drawing meaningful conclusions from small samples.
Ignoring Assumptions of Tests
Many statistical tests rely on specific assumptions about the data, such as normal distribution or homogeneity of variances. Ignoring these assumptions can lead to inaccurate results. Common pitfalls include:
- Applying Parametric Tests to Non-Normal Data: Using parametric tests like t-tests or ANOVA on data that do not follow a normal distribution.
- Assumption Violations in ANOVA: Not checking for homogeneity of variances in one-way or two-way ANOVA.
Mitigation: Always assess whether your data meets the assumptions of the chosen statistical test. If assumptions are violated, consider non-parametric alternatives or transformations to meet the assumptions.
Data Snooping and Overfitting
Data snooping, or data dredging, occurs when you explore your data extensively, increasing the risk of finding spurious patterns. Overfitting happens when a model is too complex and fits the sample data closely, leading to poor generalization of new data. Common pitfalls include:
- Testing Multiple Hypotheses Without Correction: Conducting numerous tests without adjusting alpha levels for multiple comparisons.
- Complex Models with Many Parameters: Fitting models with too many parameters to limited data.
Mitigation: Use appropriate correction methods for multiple comparisons, collect new data for model validation, or use simpler models to avoid overfitting.
By recognizing and mitigating these common mistakes and pitfalls, you can ensure more robust and reliable results in your significance testing endeavors.
How to Report and Communicate Significance?
Effectively presenting and communicating your results is essential in significance testing to convey your findings clearly and facilitate decision-making. In this section, we'll delve into various aspects of reporting and communication.
Presenting Results Effectively
Presenting your results in a clear and organized manner is crucial for others to understand and interpret your findings. Consider the following tips:
- Use Clear Language: Avoid jargon and complex terminology. Explain statistical concepts in plain language.
- Provide Context: Explain the context and relevance of your findings. How do they relate to the research question or problem?
- Highlight Key Results: Focus on the most important results. Use concise and informative headings and subheadings to guide the reader.
Creating Visualizations
Visualizations, such as charts and graphs, are powerful tools for conveying complex statistical results in an understandable way. Choose the right type of visualization for your data:
- Histograms: Display the distribution of data.
- Bar Charts: Compare categories or groups.
- Line Charts: Show trends or changes over time.
- Scatter Plots: Display relationships between variables.
- Box Plots: Visualize the spread and central tendency of data.
- Decision Trees: Illustrate decision-making processes and classification outcomes.
Ensure your visualizations are well-labeled, have clear legends, and are easy to interpret.
Writing a Results Section
A well-structured results section in a research paper or report is crucial for presenting your findings effectively. Follow these guidelines:
- Start with a Summary: Begin with a brief summary of the main results.
- Use Headings: Organize your results using clear headings and subheadings.
- Include Tables and Figures: Present key data in tables and figures for easy reference.
- Report Effect Sizes: Include effect size measures to provide a sense of the practical importance of your results.
- Discuss Statistical Significance: Mention when results are statistically significant, but avoid overemphasizing p-values.
Conveying Practical Implications
It's essential to go beyond statistical significance and discuss the practical implications of your findings:
- Explain Real-World Significance: Discuss how the results can be applied in practice and their implications for decision-making.
- Consider Stakeholders: Consider the perspectives and needs of stakeholders who may use your findings.
- Address Limitations: Acknowledge the limitations of your study and potential sources of bias or error.
- Recommendations: Offer recommendations or suggestions based on your results.
Effectively reporting and communicating significance not only ensures that your findings are understood but also contributes to their meaningful application in various fields and decision-making processes.
Needing a summary for Statistical Significance?
If you still have open questions and need visualizations of statistical significance calculation we got you! Watch our Research Director Louise Leitsch giving an insightful and easy to understand talk on statistical significance in our Webinar!
Statistical Significance Examples
Understanding statistical significance is best achieved through concrete examples illustrating its practical application. Here are a few scenarios where statistical significance plays a crucial role:
Medical Research
In clinical trials, statistical significance determines whether a new drug or treatment is effective. Researchers compare the treatment group to a control group, analyzing outcomes like symptom improvement or recovery rates. If the results show statistical significance, it suggests that the treatment has a real and positive effect on patients' health.
Example: A clinical trial for a new pain-relief medication finds that patients who received the drug reported significantly lower pain levels compared to those who received a placebo. This statistical significance indicates the drug's effectiveness.
Marketing Campaigns
Businesses use statistical significance in A/B testing to evaluate the impact of different marketing strategies. By randomly assigning customers to two groups—one exposed to the new strategy and one to the old—the company can determine if the new strategy leads to statistically significant improvements in metrics like click-through rates, conversions, or revenue.
Example: An e-commerce company tests two different email subject lines for a promotional campaign. The subject line with a higher open rate, statistically significant over a larger sample size, is chosen for the main campaign.
Quality Control
Manufacturers use statistical significance to ensure product quality and consistency. Through process control charts and hypothesis testing, they can detect significant deviations from established quality standards, leading to timely corrective actions.
Example: A car manufacturer measures the tensile strength of steel used in car frames. If a batch of steel shows a statistically significant drop in strength, the manufacturer investigates and addresses the issue to maintain safety standards.
These real-world examples showcase the diverse applications of statistical significance in various fields, highlighting its importance in making data-driven decisions, conducting meaningful research, and achieving desired outcomes.
Conclusion for Statistical Significance
Statistical significance is a powerful tool that helps us separate meaningful insights from random noise in data. It plays a crucial role in scientific research, decision-making, and various fields like medicine, business, and social sciences. By understanding its definition, importance, and applications, you can make more informed choices and draw reliable conclusions based on data.
Remember, statistical significance is just one piece of the puzzle. It should always be considered alongside effect sizes, practical implications, and contextual factors to make well-rounded decisions. So, whether you're analyzing data, conducting experiments, or interpreting research findings, keep the principles of statistical significance in mind to enhance the credibility and validity of your results.
How to Determine Statistical Significance in Minutes?
Introducing Appinio, the real-time market research platform that makes statistical significance analysis a breeze. Appinio empowers businesses to obtain instant consumer insights, enabling lightning-fast, data-driven decisions.
Forget the heavy lifting in research and tech; with Appinio, you can focus on what truly matters – making rapid, informed choices for your business, backed by real-time consumer data. Say goodbye to the stigma of dull, intimidating, or expensive market research.
- Swift Insights: From questions to insights in minutes, Appinio accelerates your path to statistical significance.
- User-Friendly: No need for a Ph.D. in research – our intuitive platform is designed for everyone.
- Global Reach: Reach your target group with precision from 1200+ characteristics, and survey them in over 90 countries.
Get facts and figures 🧠
Want to see more data insights? Our free reports are just the right thing for you!