What is Cluster Sampling? Definition, Methods, Examples
Appinio Research · 03.04.2024 · 29min read
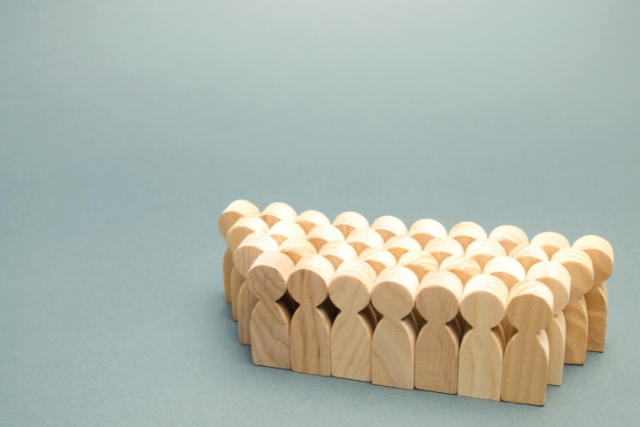
Content
Have you ever wondered how researchers can study extensive and diverse populations without having to survey every single individual? That's where cluster sampling comes into play. Imagine trying to survey every household in a city or every student in a school – it would be time-consuming, costly, and impractical.
Cluster sampling offers a solution by dividing the population into manageable clusters, such as neighborhoods or classrooms, and then selecting a sample of these clusters to represent the entire population. It's like taking a mini-snapshot of the population instead of trying to capture every single detail. This method not only saves time and resources but also allows researchers to draw accurate conclusions about the entire population based on a smaller subset.
In this guide, we'll explore the ins and outs of cluster sampling, from its basic principles to practical implementation, and equip you with the knowledge and skills to understand and apply this sampling technique effectively in your research endeavors.
What is Cluster Sampling?
Cluster sampling is a widely used sampling technique in research methodology. It involves dividing a population into clusters or groups, selecting a sample of clusters, and then sampling individuals or units within those clusters. The primary purpose of cluster sampling is to simplify the sampling process while still ensuring a representative sample of the population.
Importance of Cluster Sampling in Research
Cluster sampling holds significant importance in research methodology due to its versatility and practicality. Here are some key reasons why cluster sampling is widely used:
- Efficiency: Cluster sampling is often more efficient than other sampling methods, particularly when the population is large or geographically dispersed. By sampling entire clusters instead of individual units, researchers can save time and resources.
- Cost-Effectiveness: Sampling clusters instead of individual units can lead to cost savings, reducing the need for extensive fieldwork, travel, and data collection efforts. This makes cluster sampling particularly suitable for studies with limited budgets.
- Logistical Feasibility: Cluster sampling simplifies the sampling process by grouping individuals or units into clusters based on certain characteristics. This makes it easier to manage and execute sampling plans, especially in large-scale or complex research studies.
- Representativeness: Despite its simplification, cluster sampling can still provide a representative sample of the population if clusters are selected properly. This allows researchers to make valid inferences about the entire population based on the sampled clusters.
Cluster Sampling Principles
Understanding the basic principles of cluster sampling is essential for its effective implementation. Here are some fundamental principles:
- Cluster Formation: Clusters are formed based on specific characteristics relevant to the research study, such as geographical location, administrative boundaries, or organizational structure.
- Random Selection of Clusters: Clusters should be randomly selected from the population to ensure unbiased representation. Randomization helps minimize selection bias and ensures that every cluster has an equal chance of being included in the sample.
- Homogeneity within Clusters: Clusters should ideally be internally homogeneous, meaning that individuals or units within the same cluster are similar or alike in relevant characteristics. This helps improve the sampling efficiency and enhances the representativeness of the sample.
- Independence of Clusters: Clusters should be independent of each other to avoid duplication of sampling and ensure that each cluster contributes unique information to the sample. Overlapping or dependent clusters can lead to biased estimates and undermine the validity of the findings.
Adhering to these basic principles can help you design and implement cluster sampling studies that yield reliable and valid results, thereby advancing knowledge and understanding in your respective fields.
Understanding Cluster Sampling
Cluster sampling is a research method that simplifies data collection by dividing the population into clusters or groups. Let's explore the intricacies of cluster sampling to understand its significance and implications.
Cluster sampling involves dividing a population into clusters or groups based on certain characteristics, such as geographical location, socioeconomic status, or organizational structure. Instead of sampling individuals directly from the population, researchers randomly select entire clusters and then sample individuals within those clusters. This approach is particularly useful when it's impractical or prohibitively expensive to sample a group of people individually.
Cluster Sampling Components
Understanding the key components and terminology associated with cluster sampling is essential for effective implementation and interpretation.
- Population: The entire group of individuals or units you aim to study.
- Clusters: Groups of individuals or units that share common characteristics and are naturally occurring or artificially defined.
- Sampling Frame: A list or representation of all clusters in the population from which the sample will be drawn.
- Primary Sampling Units (PSUs): The clusters selected for inclusion in the sample.
- Secondary Sampling Units (SSUs): The individuals or units within the selected clusters sampled for data collection.
Types of Clusters
Clusters can be categorized into two main types based on their characteristics and composition:
Homogeneous Clusters
Homogeneous clusters consist of individuals or units that are similar or alike in relevant characteristics. For example, suppose a researcher is studying the effectiveness of a new teaching method in schools. In that case, they might consider schools as homogeneous clusters based on factors such as student demographics, academic performance, or teaching approach.
Heterogeneous Clusters
Heterogeneous clusters, on the other hand, comprise individuals or units that are diverse or dissimilar in relevant characteristics. In some cases, you may intentionally select heterogeneous clusters to capture a broad range of experiences or perspectives. For instance, in a study on healthcare access, communities with varying levels of socioeconomic status may be considered heterogeneous clusters.
Advantages and Disadvantages
Cluster Sampling Advantages
- Efficiency: Cluster sampling is often more efficient than other sampling methods, especially when the population is large or geographically dispersed.
- Cost-Effectiveness: By sampling entire clusters instead of individual units, you can reduce costs associated with recruitment, data collection, and analysis.
- Logistical Feasibility: Cluster sampling simplifies the sampling process, making it easier to manage and execute, particularly in field-based or community-based research.
Cluster Sampling Disadvantages
- Increased Variability: Due to the clustering of individuals within clusters, there is a risk of increased variability in the sample estimates compared to simple random sampling.
- Cluster Effects: Individuals within the same cluster may be more similar to each other than individuals in different clusters, leading to cluster effects that can bias the results.
- Complex Analysis: Analyzing cluster sampling data requires specialized statistical techniques to account for the clustered nature of the sample, which can be more complicated than analyzing data from simple random samples.
Now that you have a solid understanding of cluster sampling, let's examine how to design an effective cluster sampling plan.
How to Create a Cluster Sampling Plan?
Designing a robust cluster sampling plan is essential to ensure the validity and reliability of your research findings. We'll explore the key steps to develop a cluster sampling plan and discuss considerations for each step.
Selection of Clusters
The selection of clusters is a critical aspect of cluster sampling design. Here, you need to identify clusters that adequately represent the population of interest. Randomization is essential to ensure that every cluster has an equal chance of being included in the sample. However, practical constraints such as geographical boundaries or administrative divisions may influence the selection process.
When selecting clusters, consider factors such as:
- Homogeneity: Ensure that clusters are internally homogeneous to minimize variability within clusters.
- Representativeness: Select clusters that are representative of the population in terms of relevant characteristics.
- Feasibility: Consider logistical factors such as accessibility, cost, and resources required for sampling and data collection.
Determining Cluster Size
Determining the size of each selected cluster involves balancing the trade-off between precision and efficiency. Larger clusters may provide more statistical power but can also introduce more variability into the estimates. Conversely, smaller clusters may yield less precise estimates but can be more manageable in terms of data collection and analysis.
When determining cluster size:
- Variability within Clusters: Assess the variability of the outcome variable within clusters to determine the appropriate cluster size.
- Desired Level of Precision: Consider the desired level of precision for your estimates and how it impacts the required cluster size.
- Resources and Constraints: Take into account practical constraints such as budget, time, and available resources when determining cluster size.
Sampling Techniques within Clusters
Once clusters are selected, you need to decide on the sampling technique to be used within each cluster. Standard sampling techniques include simple random sampling, systematic sampling, and stratified sampling.
Simple Random Sampling
Simple random sampling involves randomly selecting individuals or units within each chosen cluster without any specific criteria. This method ensures that every individual within the cluster has an equal chance of being selected, thus maintaining the principle of randomness.
Systematic Sampling
Systematic sampling involves selecting individuals or units within each cluster at regular intervals, such as every nth individual or unit. This method is straightforward to implement and is useful when the population is ordered in some way, such as by geographical location or time.
Stratified Sampling
Stratified sampling involves dividing the population into subgroups or strata based on characteristics such as age, gender, or income level. Within each stratum, clusters are then randomly selected, and sampling techniques such as simple random sampling or systematic sampling are applied.
How to Calculate Sample Size and Power?
Calculating the sample size for a cluster sampling design involves accounting for the clustered nature of the sample and the potential design effect. The design effect reflects the impact of clustering on the precision of estimates and is calculated based on the average cluster size and the intracluster correlation coefficient.
The formula for calculating the sample size in a cluster sampling design is:
n = n0 / (1 + (m - 1) * ρ)
Where:
- n = required sample size
- n0 = sample size assuming simple random sampling
- m = average cluster size
- ρ = intracluster correlation coefficient
The design effect (DE) can be computed using:
DE = 1 + (m - 1) * ρ
Suppose we're conducting a study on customer satisfaction in a retail chain, and we plan to use cluster sampling. We aim for a 95% confidence level with a margin of error of 5%. Additionally, we estimate the average cluster size to be 20 customers, and the intracluster correlation coefficient is 0.05.
We first need to calculate the sample size assuming simple random sampling (n0). For a 95% confidence level with a margin of error of 5%, we consult a standard normal distribution table and find that the z-value is approximately 1.96.
n0 = (1.96)^2 * (0.25) / (0.05)^2
≈ 384.16
Next, we calculate the design effect (DE):
DE = 1 + (m - 1) * ρ
= 1 + (20 - 1) * 0.05
= 2
Finally, we compute the required sample size (n):
n = 384.16 / 2
≈ 192.08
Rounding up to the nearest whole number, we would need a sample size of approximately 193 customers per cluster to ensure the desired level of precision in our study.
Accounting for the design effect ensures that the sample size is adjusted to accommodate the clustering effect and maintain the desired level of precision. Enhancing your research precision doesn't have to be complicated. By leveraging tools like the Sample Size Calculator, you can effortlessly determine the optimal sample size needed for your study to yield reliable and representative results.
Whether you're assessing variability within clusters, aiming for a specific level of precision, or mindful of resource constraints, the Sample Size Calculator streamlines the process, ensuring your research is both efficient and effective.
How to Implement Cluster Sampling?
Implementing a cluster sampling design requires careful planning and execution to ensure the validity and reliability of your research findings.
How to Conduct Cluster Sampling?
Conducting cluster sampling involves several sequential steps, each crucial for the success of the research study. Let's outline these steps:
- Planning Phase: Define the research objectives, identify the target population, and determine the sampling frame.
- Sampling Phase: Randomly select clusters from the sampling frame and obtain consent or cooperation from cluster leaders or administrators.
- Data Collection Phase: Collect data from individuals within the selected clusters using appropriate sampling techniques.
- Analysis Phase: Analyze the collected data using statistical methods appropriate for clustered data.
- Interpretation and Reporting: Interpret the findings in the context of the research objectives and report the results, including any limitations and recommendations.
Navigating the complexities of cluster sampling can be daunting, especially when aiming for precise and representative data. However, with Appinio, you can bid farewell to the challenges of traditional sampling methods.
By leveraging our platform, you eliminate the need for cluster sampling altogether and gain the power to specify your exact sampling criteria, reaching your target audience in minutes. Say goodbye to lengthy planning phases and laborious data collection efforts. With Appinio, conducting market research becomes a seamless and efficient process, empowering you to make data-driven decisions with ease.
Ready to revolutionize your research approach? Book a demo and discover the possibilities with Appinio today!
Practical Considerations
When implementing cluster sampling, several practical considerations should be taken into account to ensure the smooth execution of the sampling plan. These include:
- Sampling Frame: Ensure that the sampling frame accurately represents the target population and is updated regularly to account for changes.
- Cluster Boundaries: Define clear boundaries for clusters to avoid overlap and ensure that each individual or unit belongs to only one cluster.
- Logistics and Resources: Allocate sufficient resources, including personnel, time, and budget, to carry out the sampling and data collection activities effectively.
- Data Collection Tools: Select appropriate data collection tools and methodologies, taking into consideration the cultural and linguistic context of the study population.
- Ethical Considerations: Obtain informed consent from participants and adhere to ethical guidelines and regulations governing human subjects research.
Addressing Potential Biases
Like any sampling method, cluster sampling is susceptible to various biases that can affect the validity and generalizability of the research findings. Some common biases associated with cluster sampling include:
- Selection Bias: Occurs when specific clusters are systematically excluded from the sampling frame, leading to an underrepresentation of particular population segments.
- Non-Response Bias: Arises when individuals or clusters selected for inclusion in the sample fail to respond or participate in the study, leading to a biased sample.
- Cluster Effects: Stem from similarities among individuals within the same cluster, which can inflate or deflate estimates of population parameters.
To address these biases, you can employ strategies such as:
- Randomization: Randomly select clusters from the sampling frame to minimize selection bias and ensure a representative sample.
- Enhanced Recruitment Efforts: Implement strategies to maximize participation and reduce non-response bias, such as offering incentives or providing multiple modes of data collection.
- Statistical Adjustment: Use statistical techniques, such as weighting or adjustment for cluster effects, to account for biases and improve the accuracy of estimates.
By keeping practical considerations in mind, such as sampling frame, logistics, and ethical considerations, and implementing strategies to address potential biases, you can conduct cluster sampling studies that yield valid and reliable results.
Cluster Sampling Examples
Understanding cluster sampling is easier with real-world examples illustrating its application across various fields. Here are a few scenarios where cluster sampling is commonly used.
Public Health Surveys
In public health research, cluster sampling is often employed to assess health outcomes, behaviors, and access to healthcare services within communities. For instance, a study might aim to evaluate the prevalence of a particular disease among residents of urban neighborhoods.
Instead of surveying every individual in each neighborhood, researchers can select a sample of neighborhoods (clusters) and then randomly sample households within those neighborhoods. This approach allows researchers to obtain representative data on health indicators while minimizing costs and logistical challenges associated with individual-level sampling.
Market Research
In market research, cluster sampling is utilized to study consumer behavior, preferences, and purchasing patterns within specific market segments. For instance, a company may wish to conduct a survey to understand consumer attitudes toward a new product in different regions of the country.
Instead of surveying individuals across the entire population, researchers can select a sample of geographic regions (clusters) and then survey households or individuals within those regions. This approach allows companies to gather market insights while optimizing resources and targeting specific consumer demographics.
Environmental Studies
In environmental research, cluster sampling can be employed to assess environmental quality, biodiversity, and ecological processes across different ecosystems or geographic regions. For example, a study might aim to investigate the impact of land use changes on wildlife populations in a particular region.
Instead of conducting field surveys across the entire landscape, researchers can select a sample of study sites (clusters) and then collect data on species abundance and habitat characteristics within those sites. Using cluster sampling, researchers can efficiently monitor environmental changes while accounting for spatial variation and ecosystem diversity.
These examples demonstrate the versatility and practicality of cluster sampling in various research contexts, highlighting its effectiveness in obtaining representative data while minimizing costs and logistical challenges. Whether in public health, education, market research, or environmental studies, cluster sampling offers a valuable tool for studying large populations and making informed decisions based on reliable data.
How to Analyze Cluster Sampling Data?
Analyzing cluster sampling data requires careful attention to detail and the use of appropriate statistical methods to draw valid conclusions from the collected data.
Data Cleaning and Preparation
Before conducting statistical analysis, cleaning and preparing the data to ensure its quality and reliability is essential. Data cleaning involves identifying and rectifying any errors, inconsistencies, or missing values in the dataset. Some key steps in data cleaning and preparation include:
- Identifying Outliers: Identify any extreme values or outliers in the dataset that may skew the results and assess whether they should be removed or adjusted.
- Handling Missing Data: Address missing data by imputing values using appropriate methods, such as mean imputation, regression imputation, or multiple imputation.
- Standardizing Variables: Standardize variables to ensure comparability and facilitate interpretation of results, especially when combining data from various sources or studies.
- Checking Data Quality: Perform checks for data quality, including assessing data completeness, consistency, and accuracy, and addressing any issues that may affect the validity of the analysis.
Statistical Analysis Methods
Cluster sampling data often exhibit complex structures due to the hierarchical nature of the sampling design. Therefore, specialized statistical methods are required to analyze clustered data appropriately. Popular statistical analysis methods used in cluster sampling studies include:
- Descriptive Statistics: Calculate summary statistics, such as means, standard deviations, and proportions, to describe the characteristics of the sample and assess the distribution of variables within and across clusters.
- Inferential Statistics: Use inferential statistics, such as hypothesis testing and confidence interval estimation, to make inferences about population parameters based on sample data.
- Multilevel Modeling: Employ multilevel or hierarchical modeling techniques to account for the nested structure of clustered data and analyze relationships at both the cluster and individual levels.
- Complex Survey Analysis: Apply specialized survey analysis methods, such as weighted regression or survey-weighted estimation, to adjust for the complex sampling design and produce unbiased estimates of population parameters.
Interpreting Results and Drawing Conclusions
Interpreting the results of cluster sampling analysis involves synthesizing findings and drawing conclusions based on the data collected. It's essential to consider the context of the research objectives, the limitations of the study design, and the implications of the findings for theory, practice, and policy.
- Comparing Results: Compare the analysis results with existing literature, theoretical frameworks, or prior research findings to contextualize the findings and identify areas of agreement or divergence.
- Assessing Generalizability: Evaluate the generalizability of the findings to the broader population and consider potential sources of bias or uncertainty that may affect the validity and reliability of the conclusions.
- Addressing Limitations: Acknowledge and address any limitations of the study, such as sampling biases, measurement errors, or methodological constraints, and discuss their implications for the interpretation of results.
- Identifying Future Directions: Identify opportunities for future research, such as exploring additional variables, refining sampling methods, or conducting follow-up studies, to further elucidate the research topic and contribute to knowledge advancement.
By following these steps and utilizing appropriate statistical analysis methods, you can effectively analyze cluster sampling data and derive meaningful insights to inform decision-making.
Cluster Sampling Challenges
Implementing cluster sampling can pose various challenges that must be addressed to ensure the validity and reliability of their study findings. Here are some common challenges encountered in cluster sampling, along with potential solutions:
- Cluster Heterogeneity: Clusters may exhibit heterogeneity in terms of characteristics relevant to the research study, leading to variability in outcomes within clusters. To address this challenge, you can employ stratified sampling techniques within clusters or adjust for cluster-level covariates in the analysis to account for heterogeneity.
- Cluster Size Disparity: Unequal cluster sizes can impact the efficiency and precision of the sampling design. You can mitigate this challenge by weighting observations based on cluster size or by using methods such as probability proportional to size (PPS) sampling to ensure that larger clusters contribute proportionally more to the sample.
- Sampling Frame Inadequacy: Inaccurate or incomplete sampling frames may result in the exclusion of eligible clusters or the inclusion of ineligible clusters, leading to sampling bias. This challenge can be addressed by conducting thorough assessments of the sampling frame's coverage and validity and implementing strategies such as frame expansion or frame adjustment to improve its representativeness.
- Cluster Non-Response: Non-response at the cluster level, such as refusal to participate or inability to access certain clusters, can introduce bias and affect the generalizability of the findings. To mitigate cluster non-response, researchers can implement proactive recruitment strategies, establish rapport with cluster leaders or gatekeepers, and provide incentives to encourage participation.
- Intracluster Correlation: Intracluster correlation, which reflects the degree of similarity among individuals within the same cluster, can inflate standard errors and lead to underestimation of sampling variability. You can mitigate this by estimating the intracluster correlation coefficient (ICC) using pilot data or existing literature and adjusting sample size calculations accordingly to account for clustering effects.
- Logistical Constraints: Logistical constraints such as limited resources, time constraints, and geographical barriers can hinder the implementation of cluster sampling. To overcome this, you must carefully plan and allocate resources, leveraging technology for remote data collection or monitoring and collaborating with local stakeholders to facilitate access to clusters.
Cluster Sampling Best Practices
To maximize the effectiveness and efficiency of cluster sampling, you should adhere to best practices throughout the research process.
- Clear Sampling Frame Definition: Define a clear and comprehensive sampling frame that accurately represents the target population and includes all eligible clusters.
- Random Cluster Selection: Use randomization techniques such as simple random sampling or systematic sampling to select clusters from the sampling frame, ensuring unbiased representation.
- Thorough Pilot Testing: Conduct pilot testing of the sampling plan and data collection procedures to identify and address any potential issues or challenges before full-scale implementation.
- Transparent Reporting: Report all aspects of the cluster sampling design clearly, including sampling methods, sample size calculations, and any deviations from the original plan, to enhance the reproducibility and transparency of the study.
- Ethical Considerations: Obtain informed consent from participants and adhere to ethical guidelines and regulations governing human subjects research, ensuring the protection of participants' rights and confidentiality.
- Continuous Monitoring and Quality Assurance: Implement robust monitoring and quality assurance mechanisms throughout the data collection process to ensure data accuracy, completeness, and reliability.
- Collaboration and Engagement: Foster collaboration and engagement with local communities, stakeholders, and cluster leaders to enhance participation, minimize non-response, and promote the relevance and applicability of the study findings.
By incorporating these best practices into their cluster sampling studies, you can enhance their research findings' validity, reliability, and ethical integrity and contribute to advancements in knowledge and understanding in their respective fields.
Conclusion for Cluster Sampling
Cluster sampling is a powerful tool that simplifies the process of studying large populations by dividing them into manageable clusters. By selecting a sample of clusters rather than individual units, you can save time, resources, and effort while still obtaining representative data. From understanding the basic principles of cluster sampling to implementing it effectively in research studies, this guide has provided you with the necessary knowledge and skills to harness the benefits of this sampling technique. Whether you're conducting research in public health, sociology, education, or any other field, cluster sampling can help you gather valuable insights and make informed decisions based on a sample that accurately reflects the diversity of the population.
In the ever-evolving landscape of research methodology, cluster sampling remains a valuable and widely used technique for sampling diverse populations. By following best practices, addressing potential biases, and utilizing appropriate statistical methods, researchers can ensure the validity and reliability of their findings. As you embark on your research journey, remember the principles and techniques outlined in this guide to apply cluster sampling in your own studies effectively. With a solid understanding of cluster sampling, you can confidently navigate the complexities of sampling large populations and contribute to advancing knowledge and understanding in your field.
How to Perfect Your Research Sampling?
Introducing Appinio, the revolutionary platform reshaping the landscape of market research. As a real-time market research platform, Appinio empowers companies to gain invaluable consumer insights swiftly and effortlessly, transforming decision-making into a seamless and intuitive process. Gone are the days of relying solely on cluster sampling – with Appinio, users can tailor their exact sampling criteria to match their research needs, ensuring precision and accuracy every time.
- Instant Insights: From posing questions to gaining valuable insights, Appinio delivers results at lightning speed, empowering you to make informed decisions in real time.
- Intuitive Platform: No need for a research PhD! Appinio's user-friendly interface ensures that anyone, regardless of their research background, can navigate and utilize the platform effortlessly.
- Global Reach: With access to over 90 countries and the ability to define target groups based on 1200+ characteristics, Appinio offers unparalleled reach and flexibility for your market research endeavors.
Get facts and figures 🧠
Want to see more data insights? Our free reports are just the right thing for you!