Weighting Survey Data: Methods, Process, Examples
Appinio Research · 29.11.2023 · 36min read
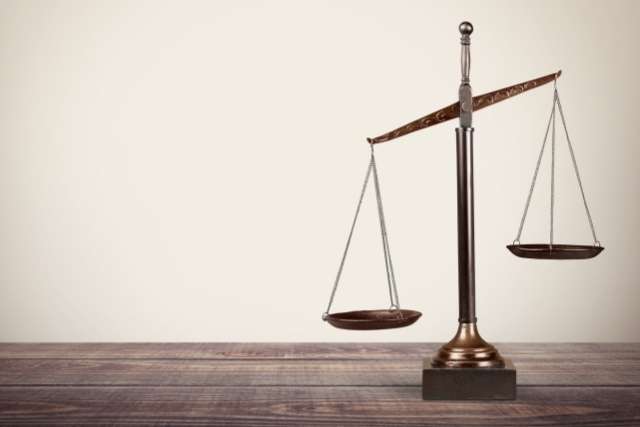
Content
Are your survey results telling the whole story or missing crucial insights due to sample biases and skewed representations? In the realm of survey research, the art of data weighting holds the key to unlocking the true potential of your data. It's a practice that allows you to recalibrate your survey findings, making them not only accurate but also reflective of the broader population you seek to understand.
In this guide, we delve deep into the world of data weighting, unraveling its principles, techniques, and the pivotal role it plays in ensuring your survey research yields actionable and reliable insights.
What is Data Weighting?
Data weighting is a fundamental process in survey research that involves adjusting survey data to account for sample biases and discrepancies between the survey sample and the target population. It aims to make the survey sample more representative of the population by assigning specific weights to survey responses based on certain characteristics or variables. These weights reflect the relative importance of each respondent's data in achieving representativeness.
Importance of Data Weighting
Data weighting holds paramount importance in survey research for several compelling reasons:
- Reducing Sampling Bias: Survey samples are rarely perfectly representative of the population they aim to study. Weighting helps reduce bias by giving more weight to underrepresented groups or demographics and less weight to overrepresented ones. This correction mitigates the impact of bias on survey results.
- Enhancing Generalizability: The primary goal of many surveys is to draw conclusions about a broader population based on a smaller sample. Data weighting improves the generalizability of survey findings, allowing researchers to make more accurate inferences about the entire population.
- Improving Data Quality: Weighting techniques help minimize errors and discrepancies in survey data. By adjusting the weights of individual responses, researchers can create a dataset with higher data quality, reducing the risk of drawing incorrect conclusions.
- Meeting Research Objectives: Researchers often have specific objectives, such as understanding the opinions of certain demographic groups. Data weighting ensures that these objectives are met by aligning the sample with the population's distribution of characteristics.
- Correcting Non-Response Bias: In cases where not all selected respondents participate in the survey (non-response), data weighting can address non-response bias. It adjusts the data to account for the differences between respondents and non-respondents.
Data weighting is a crucial step in survey research that enables researchers to obtain reliable and accurate insights. By accounting for sample biases and ensuring representativeness, data weighting enhances the validity and usefulness of survey results.
Purpose of Weighting Survey Data
The application of data weighting in survey research serves specific purposes that are instrumental in achieving research objectives:
- Mitigating Sample Biases: Survey samples are often subject to various biases, including selection bias, non-response bias, and coverage bias. Data weighting addresses these biases by adjusting the importance of each response to reflect the true distribution of the population. This correction ensures that the composition of the sample does not skew survey results.
- Achieving Representativeness: One of the primary goals of survey research is to make inferences about a target population based on a sample. Data weighting is pivotal in attaining representativeness by aligning the survey sample with the population's demographic or characteristic distribution. This alignment is essential for drawing accurate conclusions about the broader population.
- Improving Data Accuracy: Weighting techniques enhance the accuracy of survey data by minimizing errors and discrepancies. By adjusting the weights of individual responses, researchers can create a dataset that closely reflects the actual population distribution. This accuracy is critical for making informed decisions and policy recommendations.
- Supporting Subgroup Analysis: Many research studies aim to analyze specific subgroups within the population. Data weighting allows researchers to ensure that these subgroups are adequately represented in the survey sample, facilitating meaningful subgroup analyses and insights.
- Enhancing Data Quality: Weighting procedures are an integral part of data quality assurance in survey research. They help researchers identify and address data anomalies, such as non-response or over-representation of certain groups, which can lead to unreliable results if left uncorrected.
The purpose of data weighting in survey research is to address sample biases, achieve representativeness, improve data accuracy, support subgroup analysis, and enhance data quality. These objectives collectively contribute to the validity and reliability of survey findings, making data weighting an essential practice in survey research.
Data Weighting Principles
In survey research, understanding the fundamental principles of data weighting is crucial. Let's delve deeper into these principles to provide you with a solid foundation for effective data weighting.
Understanding Sample Biases
Sample bias is an inherent challenge in survey research. It occurs when the individuals who participate in your survey differ in some significant way from the broader population you're trying to study. These differences can skew your survey results, leading to inaccurate and misleading conclusions.
- Non-Response Bias: When a subset of your selected respondents does not participate in the survey, their absence can lead to non-response bias. This is particularly concerning when those who choose not to respond have distinct characteristics that differ from those who do.
- Selection Bias: If your sampling method disproportionately selects certain groups over others, you may introduce selection bias. For example, if you only survey people who are easily accessible, you might miss out on less accessible but essential segments of the population.
- Coverage Bias: Coverage bias arises when the sampling frame (the list of potential survey participants) does not accurately represent the target population. This can happen if certain groups are underrepresented or excluded from the frame.
Understanding these sources of bias is the first step in effective data weighting. By identifying potential biases in your survey, you can develop weighting strategies to mitigate their impact and obtain more accurate results.
Types of Weighting Factors
In data weighting, different types of weighting factors are used to adjust survey data. Each type of weighting factor serves a specific purpose and is applied in different scenarios.
- Probability Weights: Probability weights are assigned to each respondent based on the likelihood of their inclusion in the survey. This type of weighting helps correct for over- or under-representation of specific individuals or groups. For instance, if some segments of the population are more likely to respond to surveys, their responses may be given lower weights to balance their influence.
- Non-Response Weights: Non-response weights address the issue of respondents who choose not to participate in the survey. By assigning higher weights to respondents who are similar to those who did not respond, you can reduce non-response bias. These weights are crucial for ensuring that your sample is representative of the entire target population.
- Post-Stratification Weights: Post-stratification involves dividing your survey sample into subgroups or strata based on specific characteristics, such as age, gender, or region. Weighting factors are then applied to each stratum to align the distribution of these strata in your sample with that of the population. This technique is effective when you want to ensure that your survey accurately represents diverse demographic groups.
- Calibration Weighting: Calibration weighting, also known as raking ratio estimation, adjusts the weights based on known population totals for certain key variables. By comparing the weighted sample to external data sources, such as census data, you can ensure that your survey results align with the actual population distribution. This method is particularly useful for complex surveys with multiple weighting variables.
- Propensity Score Weighting: Propensity score weighting is commonly used in observational studies to balance groups based on their likelihood of being part of the survey. It calculates the probability of each individual responding to the survey and assigns weights accordingly. This approach reduces selection bias and helps create a more representative sample.
Considerations for Weighting Survey Data
When applying data weighting to your survey data, several critical considerations should guide your approach:
- Data Quality: Data weighting cannot correct for errors or inconsistencies in your survey data. Ensure that your data is clean, accurate, and free from biases before applying any weighting techniques.
- Transparency: Transparency is essential in survey research. Document your weighting methodology thoroughly, making it easy for others to understand and replicate your process. This transparency builds credibility and trust in your findings.
- Ethical Considerations: Data weighting involves adjusting individuals' responses, which can have ethical implications. Be sure to apply weighting methods fairly and without introducing unintended bias. Ethical considerations may also extend to privacy concerns, so handle survey data with care and in compliance with relevant regulations.
By understanding these principles and considerations, you'll be better equipped to navigate the intricacies of data weighting and ensure that your survey results are both accurate and ethically sound.
Common Data Weighting Techniques
In survey research, various weighting techniques are employed to adjust survey data and make it more representative of the target population.
Post-Stratification
Post-stratification is a robust weighting technique used to align your survey sample with the population's demographic or characteristic distribution. It involves several key steps:
- Stratification: Initially, divide your survey sample into strata or subgroups based on specific characteristics. Stratification variables include age, gender, income, education, or geographic region.
- Collect Stratum Data: Obtain data on the distribution of the chosen stratification variables in the actual population. This data typically comes from external sources like census reports.
- Calculate Weights: Calculate weights for each respondent based on their stratum's share of the population. Respondents in strata that are underrepresented in the survey sample receive higher weights, while those in overrepresented strata receive lower weights.
- Apply Weights: Multiply each respondent's data by their assigned weight to create a weighted dataset. This adjusted data reflects the distribution of the target population more accurately.
Post-stratification is particularly effective when a survey sample doesn't closely mirror the population's characteristics. It helps reduce bias and ensures that your survey results are more reliable and representative.
Raking
Raking is a versatile and iterative weighting technique commonly used in complex survey designs. It is especially suitable when you have multiple variables that need adjustment simultaneously. Here's how raking works:
- Select Weighting Variables: Identify the variables you want to use for weighting. These can include demographic factors like age, gender, race, or any other relevant characteristics.
- Obtain Population Data: Gather external data representing the distribution of your selected weighting variables in the target population. This data often comes from census reports or other authoritative sources.
- Iterative Weight Adjustment: In an iterative process, adjust the weights of survey respondents to match the distribution of the population data for each weighting variable. The process repeats until the weighted sample closely aligns with the population distribution for all variables.
- Create Weighted Dataset: Once the raking process is complete, you'll have a weighted dataset that accurately reflects the target population's characteristics.
Raking is a flexible approach that can handle complex survey designs with numerous weighting variables. It's advantageous when you need to account for various demographic factors simultaneously to ensure representativeness.
Rim Weighting
Rim weighting is a technique employed when your survey sample does not adequately cover all possible combinations of multiple variables. It redistributes weights to ensure that the weighted sample approximates the population distribution more closely. Here's how it works:
- Identify Key Variables: Determine the essential variables that are crucial for achieving representativeness. These variables could be combinations of demographic factors, such as age, gender, and ethnicity.
- Calculate Initial Weights: Initially, assign weights to respondents based on the sample's distribution of key variables.
- Iterative Adjustment: In an iterative process, adjust the weights to match the population distribution of the key variables. This adjustment continues until the weighted sample closely aligns with the population.
- Create Weighted Data: The final output is a weighted dataset that more accurately represents the population, accounting for variations in the combinations of key variables.
Rim weighting is particularly helpful when your survey has complex interactions between variables, and no single variable can adequately represent the population's diversity. It ensures that your weighted data reflects the intricate characteristics of your target population.
Calibration Weighting
Calibration weighting, also known as ranking ratio estimation, is a method that adjusts survey weights based on known population totals for specific variables. This technique ensures that your survey results are in harmony with external data sources, such as census data. Here's how it's done:
- Select Calibration Variables: Identify the key variables that you want to calibrate your survey to, such as population totals for age groups, gender, or other demographic factors.
- Obtain Population Totals: Gather reliable population totals for the selected calibration variables from authoritative sources like census data.
- Calculate Calibration Ratios: Divide the population totals by the corresponding survey sample totals for each calibration variable. These ratios represent how much your survey sample deviates from the population.
- Apply Calibration Weights: Adjust the weights of survey respondents by multiplying their initial weights by the calibration ratios. This process aligns the survey results with the known population totals for the calibration variables.
Calibration weighting is particularly useful when you need your survey results to match external data sources precisely. It's a robust technique for ensuring your survey findings are consistent with established population statistics.
Propensity Score Weighting
Propensity score weighting is a valuable technique in observational studies where you want to balance groups based on their likelihood of being part of the survey. It reduces selection bias and helps create a more representative sample. Here's how it's applied:
- Estimate Propensity Scores: Develop a model that estimates the probability (propensity score) of each individual participating in the survey. This model considers various factors, such as demographics and behavioral characteristics.
- Calculate Propensity Weights: For each respondent, calculate the propensity weight as the inverse of their estimated propensity score. Individuals with a high probability of being surveyed receive lower weights, while those with a low probability receive higher weights.
- Apply Propensity Weights: Multiply each respondent's data by their corresponding propensity weight to create a weighted dataset. This adjustment ensures that groups with different propensities to participate in the survey are adequately represented.
Propensity score weighting is particularly useful when accounting for self-selection bias in observational studies. It balances the characteristics of participants and non-participants, leading to more accurate and generalizable results.
By understanding and choosing the appropriate weighting technique for your survey research, you can ensure that your data accurately reflects the characteristics and distribution of the target population. Each method has its strengths and is suited to different survey designs and objectives.
Weighting Survey Data: How It Works?
Now that you have a clear understanding of the principles and weighting techniques, let's delve into the practical steps for effectively implementing data weighting. These steps will guide you through the process of adjusting your survey data to ensure it accurately represents your target population.
1. Clean and Prepare Your Data
Data cleaning and preparation are fundamental steps in the data weighting process. Before you can apply any weighting techniques, it's essential to ensure that your survey data is of high quality and free from errors or biases. Here's how to approach this critical phase:
- Identify Data Issues: Begin by identifying any issues in your survey data. This may include missing responses, outliers, inconsistent formatting, or any other data anomalies.
- Data Validation: Perform thorough data validation to check for accuracy and completeness. This may involve running data integrity checks and verifying that responses are within expected ranges.
- Address Missing Data: Develop a strategy for handling missing data. You can choose to impute missing values using statistical methods or make informed decisions about how to handle missing responses based on the context.
- Outlier Handling: Identify and address outliers that may distort the data. Depending on the nature of the outliers, you can choose to retain, transform, or remove them.
- Data Standardization: Standardize data formats and coding schemes to ensure consistency across all variables. This step is crucial for accurate analysis and weighting.
- Document Data Cleaning Procedures: Keep detailed records of the steps you've taken to clean and prepare your data. Transparent documentation is essential for reproducibility and research integrity.
By thoroughly cleaning and preparing your survey data, you lay the foundation for accurate and reliable results after applying weighting techniques.
2. Identify Weighting Variables
Identifying the appropriate variables for weighting is a critical decision in the data weighting process. These variables should be chosen strategically to address sample biases and align your survey sample with the population. Here's how to identify weighting variables:
- Review Survey Objectives: Start by revisiting the objectives of your survey research. Understanding what you aim to achieve will guide your choice of weighting variables.
- Examine Demographic Factors: Consider demographic variables such as age, gender, race, income, education, and geographic location. These are often essential factors for ensuring representativeness.
- Evaluate Survey Design: Review your survey design and sampling methodology. Identify the most relevant variables for adjusting your sample to match the population distribution.
- Consult External Data Sources: If available, consult external data sources, such as census data or government statistics, to gain insights into the distribution of key characteristics in the population.
- Consider Research Context: Take into account the specific context of your research. Some surveys may require unique weighting variables based on the research objectives.
- Consult Experts: When in doubt, consult with survey research experts or statisticians who can provide guidance on selecting appropriate weighting variables.
The choice of weighting variables should align with your research goals and the characteristics most relevant to achieving representativeness in your survey.
3. Calculate Weighting Factors
Once you've identified the weighting variables, the next step is to calculate the weighting factors. These factors determine how much each respondent's data should be adjusted to match the population distribution accurately. Here's how to calculate weighting factors:
- Stratification-Based Weights (Post-Stratification): If you're using post-stratification, calculate the weights for each respondent based on their stratum's share of the population. This involves dividing the population count for each stratum by the sample count for the same stratum.
- Raking-Based Weights: For raking, calculate weights iteratively by comparing the sample distribution of each weighting variable with the population distribution. Adjust the weights until they converge to a stable solution that closely matches the population distribution.
- Calibration-Based Weights: In calibration weighting, calculate weights by dividing the population totals for the calibration variables by the corresponding survey sample totals. These ratios serve as calibration weights.
- Propensity Score Weights: For propensity score weighting, estimate the propensity score for each respondent using a statistical model. Then, calculate the propensity weight as the inverse of the estimated propensity score.
Ensure that your calculations are accurate and consistent to avoid introducing errors during the weighting process.
4. Apply Weighting to Survey Data
The final step in implementing data weighting is applying the calculated weighting factors to your survey data. This adjustment ensures that the weighted data accurately reflects the distribution of your target population. Here's how to apply weighting to your survey data:
- Create a Weighted Variable: Add a new variable to your dataset to store the calculated weighting factors. This variable will be used to adjust the data for each respondent.
- Multiply Data by Weights: Multiply each respondent's data by their corresponding weighting factor. This process scales the responses to align with the population distribution.
- Generate Weighted Dataset: Create a new dataset or save the adjusted data with the applied weights. This dataset is now ready for analysis and reporting.
- Document Weighting Procedure: Document the weighting procedure you've used, including the choice of weighting variables and the calculations involved. Transparency is vital for research integrity.
5. Leverage Market Research Tools
In today's fast-paced world, where timely and accurate insights are paramount, feedback tools and market research platforms come to the forefront as invaluable tools for survey research. These platforms not only streamline data collection but also offer robust features for data weighting, ensuring that your survey results are impeccable from start to finish.
- Automated Data Collection: These tools automate the data collection process, making it quick and efficient. Say goodbye to manual data entry and welcome real-time responses.
- Built-in Weighting Tools: Appinio comes equipped with built-in weighting tools. These tools simplify the weighting process, ensuring your survey data accurately represents your target population.
- Immediate Insights: With a platform like Appinio, you can access insights within minutes, eliminating the need for time-consuming data processing. Get a better picture of your results and real insights in a fraction of the time.
Efficiently implement data weighting with the help of advanced market research platforms like Appinio. Appinio offers built-in weighting tools that streamline the process, ensuring your survey data accurately reflects your target population. With automated data collection and real-time insights, you can make data-driven decisions faster and more effectively.
Elevate your survey research and experience the difference in the quality and timeliness of your results. Book a demo now to see how Appinio can enhance your survey research process!
Examples of Weighting Survey Data
To provide a clearer understanding of how data weighting is applied in survey research, let's explore some real-world examples where weighting techniques have been used to enhance the accuracy and representativeness of survey findings:
Political Polling
Example: Imagine a political polling organization conducting a survey to gauge public opinion on an upcoming election. The survey results show that respondents aged 18-24 are significantly overrepresented in the sample, while respondents aged 65 and above are underrepresented.
Application of Data Weighting: To ensure that the survey results accurately reflect the demographic distribution of the voting population, the organization applies data weighting. They assign higher weights to responses from the 65+ age group and lower weights to responses from the 18-24 age group, aligning the sample with the actual voter demographics. This weighting process helps produce election predictions that are more reliable and representative of the entire voting population.
Customer Satisfaction Surveys
Example: A multinational corporation conducts a customer satisfaction survey across its diverse customer base. After collecting responses, they notice that certain geographic regions, such as rural areas, are underrepresented in the survey results.
Application of Data Weighting: To accurately assess overall customer satisfaction and address regional variations, the corporation applies data weighting. They assign higher weights to responses from underrepresented regions, ensuring that each region's views carry the appropriate influence in the analysis. This weighting technique enables the corporation to make informed decisions about improving customer experiences in all areas.
Healthcare Surveys
Example: A healthcare research institute conducts a national survey to understand healthcare access and utilization. They find that respondents with lower incomes and less education are less likely to participate in the survey, leading to potential bias in the results.
Application of Data Weighting: To correct for non-response bias and achieve a representative sample, the research institute employs data weighting. They assign higher weights to respondents with lower incomes and less education, compensating for their underrepresentation in the sample. This weighting ensures that the survey findings accurately reflect the healthcare experiences of diverse socioeconomic groups, making the results more informative for policymakers and healthcare providers.
Employee Engagement Surveys
Example: A company conducts an annual employee engagement survey, but the response rate varies among departments. Some departments have high participation, while others have lower participation rates.
Application of Data Weighting: To ensure that the survey results are representative of the entire workforce, the company applies data weighting. They assign higher weights to responses from departments with lower participation rates, allowing for a fair representation of the views and concerns of all employees. This weighting practice helps the company identify areas of improvement in employee engagement across the organization.
Social Research on Attitudes
Example: A social research organization conducts a study on public attitudes toward environmental conservation. They find that respondents with strong environmental interests are overrepresented in the survey, potentially leading to skewed results.
Application of Data Weighting: To account for the overrepresentation of certain attitudes, the research organization applies data weighting. They assign lower weights to responses from individuals with strong environmental interests and higher weights to those with less pronounced interests. This weighting technique ensures that the survey accurately captures the diverse range of attitudes within the population, providing a more comprehensive understanding of public sentiment toward conservation efforts.
In these examples, data weighting serves as a vital tool for addressing sample biases, enhancing representativeness, and improving the accuracy of survey results. Whether in political polling, customer satisfaction research, healthcare studies, employee engagement surveys, or social research, data weighting is pivotal in ensuring that survey findings are reliable and actionable.
How to Evaluate the Effectiveness of Data Weighting?
After applying data weighting to your survey data, it's essential to assess the effectiveness of this process and ensure that your survey results are more accurate and reliable. In this section, we will explore how to evaluate the impact of data weighting and make necessary adjustments when required.
Weighted vs. Unweighted Results
One of the critical steps in evaluating the effectiveness of data weighting is to compare your survey results before and after applying weighting. This comparison allows you to understand how weighting has affected the outcomes and whether it has successfully reduced biases in the data.
- Generate Unweighted Analysis: Begin by analyzing your survey data without applying any weighting factors. This analysis will provide you with the unweighted results.
- Generate Weighted Analysis: Next, perform the same analysis on the weighted dataset. This analysis will give you the weighted results, which account for the population distribution.
- Compare Key Metrics: Compare key metrics and findings between the unweighted and weighted analyses. Look for significant discrepancies and differences in outcomes.
- Identify Changes: Pay attention to changes in proportions, means, or other statistical measures. Assess whether weighting has brought the results closer to what you would expect from the population.
- Examine Bias Reduction: Evaluate the extent to which data weighting has reduced bias in the survey results. Ideally, weighting should minimize the differences between the unweighted and weighted outcomes.
By comparing unweighted and weighted results, you can gain insights into the effectiveness of data weighting in improving the accuracy and representativeness of your survey findings.
Monitoring and Adjusting Weighting
Data weighting is not a one-time process but a dynamic one that may require ongoing monitoring and adjustments. It's crucial to continuously assess the effectiveness of your weighting methodology and make necessary changes when needed.
- Establish Monitoring Procedures: Set up a system for regularly monitoring the effectiveness of your data weighting. This may involve periodic reviews of survey data and weighted outcomes.
- Assess Weighting Impact: Continuously assess the impact of weighting on your survey outcomes. Look for any unexpected shifts or patterns in the data.
- Conduct Sensitivity Analyses: Perform sensitivity analyses to test the robustness of your weighting methods. Evaluate how different weighting approaches or parameters affect the results.
- Consult Statistical Experts: If you encounter challenges or uncertainties in the weighting process, consider consulting with statistical experts or colleagues experienced in survey research.
- Adjust Weighting Methods: Based on your monitoring and sensitivity analyses, be prepared to adjust your weighting methods. You may need to fine-tune the weighting factors or explore alternative weighting techniques.
- Document Changes: Always document any changes made to your weighting methodology. Transparent documentation ensures that your methodology is reproducible and can be validated by others.
Continuous monitoring and adjustment of weighting methods are essential for maintaining the accuracy and reliability of your survey results over time.
Impact of Data Weighting on Survey Outcomes
Data weighting can significantly impact the outcomes and conclusions of your survey. It's essential to thoroughly evaluate how weighting has influenced the survey results and whether it has achieved its intended goals. To assess the impact of data weighting on survey outcomes:
- Compare Weighted vs. Unweighted Findings: Beyond the initial comparison, conduct a detailed analysis of how specific survey questions or variables have been affected by weighting. Look for shifts in distributions and patterns.
- Examine Statistical Significance: Assess whether the differences between weighted and unweighted results are statistically significant. Determine whether these differences are practically meaningful for your research objectives.
- Review Confidence Intervals: Examine the confidence intervals associated with key survey estimates. Weighting can impact the precision of estimates, so ensure that the intervals are appropriately adjusted.
- Consider Subgroup Analysis: If your survey includes subgroup analyses, evaluate how weighting has affected these subpopulations. Assess whether the weighting has improved the representativeness of these groups.
- Interpret Findings in Context: Always interpret your survey findings in the context of the weighting methodology applied. Be transparent in reporting the impact of weighting on your conclusions.
Understanding how data weighting has influenced survey outcomes is essential for drawing valid inferences and making informed decisions based on your research. By carefully evaluating the impact, you can ensure that your weighted results align with your research objectives and accurately represent the target population.
Data Weighting Best Practices
Implementing data weighting effectively requires adherence to best practices to ensure the accuracy, transparency, and ethical soundness of your survey research.
- Transparent Documentation: Thoroughly document your data weighting methodology, including the choice of weighting variables, calculation procedures, and any adjustments made during the process. Transparent documentation enables others to understand and replicate your work, enhancing research integrity.
- Ethical Considerations: Be mindful of ethical considerations when applying data weighting. Weighting involves adjusting individual responses, and it's crucial to do so fairly and without introducing unintended bias. Respect privacy and confidentiality regulations while handling survey data.
- Data Quality Assurance: Ensure your survey data is of high quality before weighting. Address missing data, outliers, and inconsistencies through rigorous data cleaning and validation processes. Weighting cannot correct flawed data, so data quality is paramount.
- Sensitivity Analysis: Conduct sensitivity analyses to assess the robustness of your weighting methodology. Test the impact of different weighting approaches or parameters on survey outcomes to understand the potential variability in results.
- Regular Monitoring: Establish procedures for regularly monitoring the effectiveness of your data weighting. Continuously assess the impact of weighting on survey outcomes and be prepared to make adjustments when needed.
- Expert Consultation: Seek guidance from statistical experts or colleagues with expertise in survey research, especially when faced with complex survey designs or challenges in the weighting process. Expert input can help ensure the validity of your weighting methods.
- Consider Research Context: Take into account the specific context and objectives of your research when selecting weighting variables and methods. Tailor your approach to the unique requirements of your survey.
- Dual Reporting: Consider reporting both weighted and unweighted results in your research findings. This dual reporting allows readers to assess the impact of weighting on the conclusions and better understand the representativeness of the data.
- Educate Stakeholders: If your survey results will be used by decision-makers or the public, provide education on the importance of data weighting and its impact on the reliability of survey findings. Clear communication can prevent misinterpretation.
- Ethical Transparency: When reporting your survey results, be transparent about the weighting methods used. Clearly state the rationale behind weighing decisions and any ethical considerations taken into account during the process.
By adhering to these best practices, you can conduct data weighting responsibly and effectively, ultimately ensuring that your survey results are accurate and trustworthy. Properly weighted data enhances the credibility of your research and supports informed decision-making based on reliable insights.
Conclusion
Data weighting is the compass that helps you navigate the sometimes choppy waters of survey research. It's the tool that allows you to correct course when your data isn't quite on target, making your survey results more accurate and representative of the population you're studying. By understanding the principles, techniques, and best practices of data weighting, you can ensure that your survey findings are reliable and provide a clear and unbiased view of the world you're exploring.
Remember, data weighting is not a mysterious art but a science grounded in solid methodologies. It's a fundamental practice that empowers you to draw meaningful conclusions from your survey research, whether you're probing public opinions, assessing customer satisfaction, or diving into social issues.
How to Automate Weighing Survey Data?
Appinio, the real-time market research platform, is here to revolutionize the way you collect and weigh survey data. Imagine conducting your own market research in minutes, with the confidence that your insights truly represent your target audience. With Appinio, it's not just about speed; it's about accuracy and simplicity. Here's why you should choose Appinio:
- Instant Insights: From formulating questions to gaining actionable insights, Appinio accelerates the entire process, delivering results in minutes.
- User-Friendly: You don't need a research degree to navigate our platform. It's so intuitive that anyone can dive in and start collecting valuable data.
- Global Reach: With access to over 90 countries and 1,200+ characteristics, you can define your target group precisely and gather data from diverse demographics.
Join the loop 💌
Be the first to hear about new updates, product news, and data insights. We'll send it all straight to your inbox.