What is Meta-Analysis? Definition, Research & Examples
Appinio Research · 01.02.2024 · 39min read
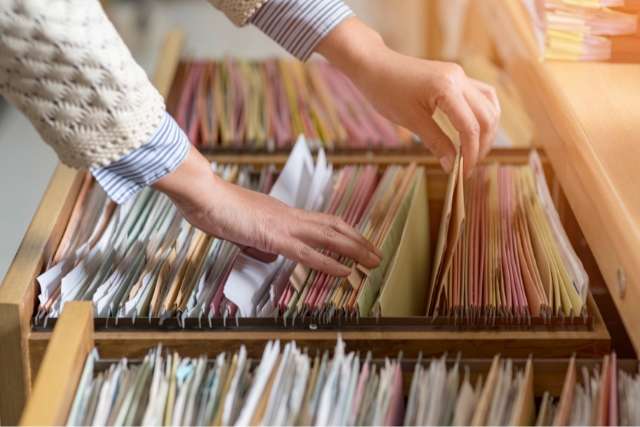
Content
Are you looking to harness the power of data and uncover meaningful insights from a multitude of research studies? In a world overflowing with information, meta-analysis emerges as a guiding light, offering a systematic and quantitative approach to distilling knowledge from a sea of research.
This guide will demystify the art and science of meta-analysis, walking you through the process, from defining your research question to interpreting the results. Whether you're an academic researcher, a policymaker, or a curious mind eager to explore the depths of data, this guide will equip you with the tools and understanding needed to undertake robust and impactful meta-analyses.
What is a Meta Analysis?
Meta-analysis is a quantitative research method that involves the systematic synthesis and statistical analysis of data from multiple individual studies on a particular topic or research question. It aims to provide a comprehensive and robust summary of existing evidence by pooling the results of these studies, often leading to more precise and generalizable conclusions.
The primary purpose of meta-analysis is to:
- Quantify Effect Sizes: Determine the magnitude and direction of an effect or relationship across studies.
- Evaluate Consistency: Assess the consistency of findings among studies and identify sources of heterogeneity.
- Enhance Statistical Power: Increase the statistical power to detect significant effects by combining data from multiple studies.
- Generalize Results: Provide more generalizable results by analyzing a more extensive and diverse sample of participants or contexts.
- Examine Subgroup Effects: Explore whether the effect varies across different subgroups or study characteristics.
Importance of Meta-Analysis
Meta-analysis plays a crucial role in scientific research and evidence-based decision-making. Here are key reasons why meta-analysis is highly valuable:
- Enhanced Precision: By pooling data from multiple studies, meta-analysis provides a more precise estimate of the effect size, reducing the impact of random variation.
- Increased Statistical Power: The combination of numerous studies enhances statistical power, making it easier to detect small but meaningful effects.
- Resolution of Inconsistencies: Meta-analysis can help resolve conflicting findings in the literature by systematically analyzing and synthesizing evidence.
- Identification of Moderators: It allows for the identification of factors that may moderate the effect, helping to understand when and for whom interventions or treatments are most effective.
- Evidence-Based Decision-Making: Policymakers, clinicians, and researchers use meta-analysis to inform evidence-based decision-making, leading to more informed choices in healthcare, education, and other fields.
- Efficient Use of Resources: Meta-analysis can guide future research by identifying gaps in knowledge, reducing duplication of efforts, and directing resources to areas with the most significant potential impact.
Types of Research Questions Addressed
Meta-analysis can address a wide range of research questions across various disciplines. Some common types of research questions that meta-analysis can tackle include:
- Treatment Efficacy: Does a specific medical treatment, therapy, or intervention have a significant impact on patient outcomes or symptoms?
- Intervention Effectiveness: How effective are educational programs, training methods, or interventions in improving learning outcomes or skills?
- Risk Factors and Associations: What are the associations between specific risk factors, such as smoking or diet, and the likelihood of developing certain diseases or conditions?
- Impact of Policies: What is the effect of government policies, regulations, or interventions on social, economic, or environmental outcomes?
- Psychological Constructs: How do psychological constructs, such as self-esteem, anxiety, or motivation, influence behavior or mental health outcomes?
- Comparative Effectiveness: Which of two or more competing interventions or treatments is more effective for a particular condition or population?
- Dose-Response Relationships: Is there a dose-response relationship between exposure to a substance or treatment and the likelihood or severity of an outcome?
Meta-analysis is a versatile tool that can provide valuable insights into a wide array of research questions, making it an indispensable method in evidence synthesis and knowledge advancement.
Meta-Analysis vs. Systematic Review
In evidence synthesis and research aggregation, meta-analysis and systematic reviews are two commonly used methods, each serving distinct purposes while sharing some similarities. Let's explore the differences and similarities between these two approaches.
Meta-Analysis
- Purpose: Meta-analysis is a statistical technique used to combine and analyze quantitative data from multiple individual studies that address the same research question. The primary aim of meta-analysis is to provide a single summary effect size that quantifies the magnitude and direction of an effect or relationship across studies.
- Data Synthesis: In meta-analysis, researchers extract and analyze numerical data, such as means, standard deviations, correlation coefficients, or odds ratios, from each study. These effect size estimates are then combined using statistical methods to generate an overall effect size and associated confidence interval.
- Quantitative: Meta-analysis is inherently quantitative, focusing on numerical data and statistical analyses to derive a single effect size estimate.
- Main Outcome: The main outcome of a meta-analysis is the summary effect size, which provides a quantitative estimate of the research question's answer.
Systematic Review
- Purpose: A systematic review is a comprehensive and structured overview of the available evidence on a specific research question. While systematic reviews may include meta-analysis, their primary goal is to provide a thorough and unbiased summary of the existing literature.
- Data Synthesis: Systematic reviews involve a meticulous process of literature search, study selection, data extraction, and quality assessment. Researchers may narratively synthesize the findings, providing a qualitative summary of the evidence.
- Qualitative: Systematic reviews are often qualitative in nature, summarizing and synthesizing findings in a narrative format. They do not always involve statistical analysis.
- Main Outcome: The primary outcome of a systematic review is a comprehensive narrative summary of the existing evidence. While some systematic reviews include meta-analyses, not all do so.
Key Differences
- Nature of Data: Meta-analysis primarily deals with quantitative data and statistical analysis, while systematic reviews encompass both quantitative and qualitative data, often presenting findings in a narrative format.
- Focus on Effect Size: Meta-analysis focuses on deriving a single, quantitative effect size estimate, whereas systematic reviews emphasize providing a comprehensive overview of the literature, including study characteristics, methodologies, and key findings.
- Synthesis Approach: Meta-analysis is a quantitative synthesis method, while systematic reviews may use both quantitative and qualitative synthesis approaches.
Commonalities
- Structured Process: Both meta-analyses and systematic reviews follow a structured and systematic process for literature search, study selection, data extraction, and quality assessment.
- Evidence-Based: Both approaches aim to provide evidence-based answers to specific research questions, offering valuable insights for decision-making in various fields.
- Transparency: Both meta-analyses and systematic reviews prioritize transparency and rigor in their methodologies to minimize bias and enhance the reliability of their findings.
While meta-analysis and systematic reviews share the overarching goal of synthesizing research evidence, they differ in their approach and main outcomes. Meta-analysis is quantitative, focusing on effect sizes, while systematic reviews provide comprehensive overviews, utilizing both quantitative and qualitative data to summarize the literature. Depending on the research question and available data, one or both of these methods may be employed to provide valuable insights for evidence-based decision-making.
How to Conduct a Meta-Analysis?
Planning a meta-analysis is a critical phase that lays the groundwork for a successful and meaningful study. We will explore each component of the planning process in more detail, ensuring you have a solid foundation before diving into data analysis.
How to Formulate Research Questions?
Your research questions are the guiding compass of your meta-analysis. They should be precise and tailored to the topic you're investigating. To craft effective research questions:
- Clearly Define the Problem: Start by identifying the specific problem or topic you want to address through meta-analysis.
- Specify Key Variables: Determine the essential variables or factors you'll examine in the included studies.
- Frame Hypotheses: If applicable, create clear hypotheses that your meta-analysis will test.
For example, if you're studying the impact of a specific intervention on patient outcomes, your research question might be: "What is the effect of Intervention X on Patient Outcome Y in published clinical trials?"
Eligibility Criteria
Eligibility criteria define the boundaries of your meta-analysis. By establishing clear criteria, you ensure that the studies you include are relevant and contribute to your research objectives. Key considerations for eligibility criteria include:
- Study Types: Decide which types of studies will be considered (e.g., randomized controlled trials, cohort studies, case-control studies).
- Publication Time Frame: Specify the publication date range for included studies.
- Language: Determine whether studies in languages other than your primary language will be included.
- Geographic Region: If relevant, define any geographic restrictions.
Your eligibility criteria should strike a balance between inclusivity and relevance. Excluding certain studies based on valid criteria ensures the quality and relevance of the data you analyze.
Search Strategy
A robust search strategy is fundamental to identifying all relevant studies. To create an effective search strategy:
- Select Databases: Choose appropriate databases that cover your research area (e.g., PubMed, Scopus, Web of Science).
- Keywords and Search Terms: Develop a comprehensive list of relevant keywords and search terms related to your research questions.
- Search Filters: Utilize search filters and Boolean operators (AND, OR) to refine your search queries.
- Manual Searches: Consider conducting hand-searches of key journals and reviewing the reference lists of relevant studies for additional sources.
Remember that the goal is to cast a wide net while maintaining precision to capture all relevant studies.
Data Extraction
Data extraction is the process of systematically collecting information from each selected study. It involves retrieving key data points, including:
- Study Characteristics: Author(s), publication year, study design, sample size, duration, and location.
- Outcome Data: Effect sizes, standard errors, confidence intervals, p-values, and any other relevant statistics.
- Methodological Details: Information on study quality, risk of bias, and potential sources of heterogeneity.
Creating a standardized data extraction form is essential to ensure consistency and accuracy throughout this phase. Spreadsheet software, such as Microsoft Excel, is commonly used for data extraction.
Quality Assessment
Assessing the quality of included studies is crucial to determine their reliability and potential impact on your meta-analysis. Various quality assessment tools and checklists are available, depending on the study design. Some commonly used tools include:
- Newcastle-Ottawa Scale: Used for assessing the quality of non-randomized studies (e.g., cohort, case-control studies).
- Cochrane Risk of Bias Tool: Designed for evaluating randomized controlled trials.
Quality assessment typically involves evaluating aspects such as study design, sample size, data collection methods, and potential biases. This step helps you weigh the contribution of each study to the overall analysis.
How to Conduct a Literature Review?
Conducting a thorough literature review is a critical step in the meta-analysis process. We will explore the essential components of a literature review, from designing a comprehensive search strategy to establishing clear inclusion and exclusion criteria and, finally, the study selection process.
Comprehensive Search
To ensure the success of your meta-analysis, it's imperative to cast a wide net when searching for relevant studies. A comprehensive search strategy involves:
- Selecting Relevant Databases: Identify databases that cover your research area comprehensively, such as PubMed, Scopus, Web of Science, or specialized databases specific to your field.
- Creating a Keyword List: Develop a list of relevant keywords and search terms related to your research questions. Think broadly and consider synonyms, acronyms, and variations.
- Using Boolean Operators: Utilize Boolean operators (AND, OR) to combine keywords effectively and refine your search.
- Applying Filters: Employ search filters (e.g., publication date range, study type) to narrow down results based on your eligibility criteria.
Remember that the goal is to leave no relevant stone unturned, as missing key studies can introduce bias into your meta-analysis.
Inclusion and Exclusion Criteria
Clearly defined inclusion and exclusion criteria are the gatekeepers of your meta-analysis. These criteria ensure that the studies you include meet your research objectives and maintain the quality of your analysis. Consider the following factors when establishing criteria:
- Study Types: Determine which types of studies are eligible for inclusion (e.g., randomized controlled trials, observational studies, case reports).
- Publication Time Frame: Specify the time frame within which studies must have been published.
- Language: Decide whether studies in languages other than your primary language will be included or excluded.
- Geographic Region: If applicable, define any geographic restrictions.
- Relevance to Research Questions: Ensure that selected studies align with your research questions and objectives.
Your inclusion and exclusion criteria should strike a balance between inclusivity and relevance. Rigorous criteria help maintain the quality and applicability of the studies included in your meta-analysis.
Study Selection Process
The study selection process involves systematically screening and evaluating each potential study to determine whether it meets your predefined inclusion criteria. Here's a step-by-step guide:
- Screen Titles and Abstracts: Begin by reviewing the titles and abstracts of the retrieved studies. Exclude studies that clearly do not meet your inclusion criteria.
- Full-Text Assessment: Assess the full text of potentially relevant studies to confirm their eligibility. Pay attention to study design, sample size, and other specific criteria.
- Data Extraction: For studies that meet your criteria, extract the necessary data, including study characteristics, effect sizes, and other relevant information.
- Record Exclusions: Keep a record of the reasons for excluding studies. This transparency is crucial for the reproducibility of your meta-analysis.
- Resolve Discrepancies: If multiple reviewers are involved, resolve any disagreements through discussion or a third-party arbitrator.
Maintaining a clear and organized record of your study selection process is essential for transparency and reproducibility. Software tools like EndNote or Covidence can facilitate the screening and data extraction process.
By following these systematic steps in conducting a literature review, you ensure that your meta-analysis is built on a solid foundation of relevant and high-quality studies.
Data Extraction and Management
As you progress in your meta-analysis journey, the data extraction and management phase becomes paramount. We will delve deeper into the critical aspects of this phase, including the data collection process, data coding and transformation, and how to handle missing data effectively.
Data Collection Process
The data collection process is the heart of your meta-analysis, where you systematically extract essential information from each selected study. To ensure accuracy and consistency:
- Create a Data Extraction Form: Develop a standardized data extraction form that includes all the necessary fields for collecting relevant data. This form should align with your research questions and inclusion criteria.
- Data Extractors: Assign one or more reviewers to extract data from the selected studies. Ensure they are familiar with the form and the specific data points to collect.
- Double-Check Accuracy: Implement a verification process where a second reviewer cross-checks a random sample of data extractions to identify discrepancies or errors.
- Extract All Relevant Information: Collect data on study characteristics, participant demographics, outcome measures, effect sizes, confidence intervals, and any additional information required for your analysis.
- Maintain Consistency: Use clear guidelines and definitions for data extraction to ensure uniformity across studies.
To optimize your data collection process and streamline the extraction and management of crucial information, consider leveraging innovative solutions like Appinio. With Appinio, you can effortlessly collect real-time consumer insights, ensuring your meta-analysis benefits from the latest data trends and user perspectives.
Ready to learn more? Book a demo today and unlock a world of data-driven possibilities!
Data Coding and Transformation
After data collection, you may need to code and transform the extracted data to ensure uniformity and compatibility across studies. This process involves:
- Coding Categorical Variables: If studies report data differently, code categorical variables consistently. For example, ensure that categories like "male" and "female" are coded consistently across studies.
- Standardizing Units of Measurement: Convert all measurements to a common unit if studies use different measurement units. For instance, if one study reports height in inches and another in centimeters, standardize to one unit for comparability.
- Calculating Effect Sizes: Calculate effect sizes and their standard errors or variances if they are not directly reported in the studies. Common effect size measures include Cohen's d, odds ratio (OR), and hazard ratio (HR).
- Data Transformation: Transform data if necessary to meet assumptions of statistical tests. Common transformations include log transformation for skewed data or arcsine transformation for proportions.
- Heterogeneity Adjustment: Consider using transformation methods to address heterogeneity among studies, such as applying the Freeman-Tukey double arcsine transformation for proportions.
The goal of data coding and transformation is to make sure that data from different studies are compatible and can be effectively synthesized during the analysis phase. Spreadsheet software like Excel or statistical software like R can be used for these tasks.
Handling Missing Data
Missing data is a common challenge in meta-analysis, and how you handle it can impact the validity and precision of your results. Strategies for handling missing data include:
- Contact Authors: If feasible, contact the authors of the original studies to request missing data or clarifications.
- Imputation: Consider using appropriate imputation methods to estimate missing values, but exercise caution and report the imputation methods used.
- Sensitivity Analysis: Conduct sensitivity analyses to assess the impact of missing data on your results by comparing the main analysis to alternative scenarios.
Remember that transparency in reporting how you handled missing data is crucial for the credibility of your meta-analysis.
By following these steps in data extraction and management, you will ensure the integrity and reliability of your meta-analysis dataset.
Meta-Analysis Example
Meta-analysis is a versatile research method that can be applied to various fields and disciplines, providing valuable insights by synthesizing existing evidence.
Example 1: Analyzing the Impact of Advertising Campaigns on Sales
Background: A market research agency is tasked with assessing the effectiveness of advertising campaigns on sales outcomes for a range of consumer products. They have access to multiple studies and reports conducted by different companies, each analyzing the impact of advertising on sales revenue.
Meta-Analysis Approach:
- Study Selection: Identify relevant studies that meet specific inclusion criteria, such as the type of advertising campaign (e.g., TV commercials, social media ads), the products examined, and the sales metrics assessed.
- Data Extraction: Collect data from each study, including details about the advertising campaign (e.g., budget, duration), sales data (e.g., revenue, units sold), and any reported effect sizes or correlations.
- Effect Size Calculation: Calculate effect sizes (e.g., correlation coefficients) based on the data provided in each study, quantifying the strength and direction of the relationship between advertising and sales.
- Data Synthesis: Employ meta-analysis techniques to combine the effect sizes from the selected studies. Compute a summary effect size and its confidence interval to estimate the overall impact of advertising on sales.
- Publication Bias Assessment: Use funnel plots and statistical tests to assess the potential presence of publication bias, ensuring that the meta-analysis results are not unduly influenced by selective reporting.
Findings: Through meta-analysis, the market research agency discovers that advertising campaigns have a statistically significant and positive impact on sales across various product categories. The findings provide evidence for the effectiveness of advertising efforts and assist companies in making data-driven decisions regarding their marketing strategies.
These examples illustrate how meta-analysis can be applied in diverse domains, from tech startups seeking to optimize user engagement to market research agencies evaluating the impact of advertising campaigns. By systematically synthesizing existing evidence, meta-analysis empowers decision-makers with valuable insights for informed choices and evidence-based strategies.
How to Assess Study Quality and Bias?
Ensuring the quality and reliability of the studies included in your meta-analysis is essential for drawing accurate conclusions. We'll show you how you can assess study quality using specific tools, evaluate potential bias, and address publication bias.
Quality Assessment Tools
Quality assessment tools provide structured frameworks for evaluating the methodological rigor of each included study. The choice of tool depends on the study design. Here are some commonly used quality assessment tools:
For Randomized Controlled Trials (RCTs):
- Cochrane Risk of Bias Tool: This tool assesses the risk of bias in RCTs based on six domains: random sequence generation, allocation concealment, blinding of participants and personnel, blinding of outcome assessment, incomplete outcome data, and selective reporting.
- Jadad Scale: A simpler tool specifically for RCTs, the Jadad Scale focuses on randomization, blinding, and the handling of withdrawals and dropouts.
For Observational Studies:
- Newcastle-Ottawa Scale (NOS): The NOS assesses the quality of cohort and case-control studies based on three categories: selection, comparability, and outcome.
- ROBINS-I: Designed for non-randomized studies of interventions, the Risk of Bias in Non-randomized Studies of Interventions tool evaluates bias in domains such as confounding, selection bias, and measurement bias.
- MINORS: The Methodological Index for Non-Randomized Studies (MINORS) assesses non-comparative studies and includes items related to study design, reporting, and statistical analysis.
Bias Assessment
Evaluating potential sources of bias is crucial to understanding the limitations of the included studies. Common sources of bias include:
- Selection Bias: Occurs when the selection of participants is not random or representative of the target population.
- Performance Bias: Arises when participants or researchers are aware of the treatment or intervention status, potentially influencing outcomes.
- Detection Bias: Occurs when outcome assessors are not blinded to the treatment groups.
- Attrition Bias: Results from incomplete data or differential loss to follow-up between treatment groups.
- Reporting Bias: Involves selective reporting of outcomes, where only positive or statistically significant results are published.
To assess bias, reviewers often use the quality assessment tools mentioned earlier, which include domains related to bias, or they may specifically address bias concerns in the narrative synthesis.
We'll move on to the core of meta-analysis: data synthesis. We'll explore different effect size measures, fixed-effect versus random-effects models, and techniques for assessing and addressing heterogeneity among studies.
Data Synthesis
Now that you've gathered data from multiple studies and assessed their quality, it's time to synthesize this information effectively.
Effect Size Measures
Effect size measures quantify the magnitude of the relationship or difference you're investigating in your meta-analysis. The choice of effect size measure depends on your research question and the type of data provided by the included studies. Here are some commonly used effect size measures:
Continuous Outcome Data:
- Cohen's d: Measures the standardized mean difference between two groups. It's suitable for continuous outcome variables.
- Hedges' g: Similar to Cohen's d but incorporates a correction factor for small sample sizes.
Binary Outcome Data:
- Odds Ratio (OR): Used for dichotomous outcomes, such as success/failure or presence/absence.
- Risk Ratio (RR): Similar to OR but used when the outcome is relatively common.
Time-to-Event Data:
- Hazard Ratio (HR): Used in survival analysis to assess the risk of an event occurring over time.
- Risk Difference (RD): Measures the absolute difference in event rates between two groups.
Selecting the appropriate effect size measure depends on the nature of your data and the research question. When effect sizes are not directly reported in the studies, you may need to calculate them using available data, such as means, standard deviations, and sample sizes.
Formula for Cohen's d:
d = (Mean of Group A - Mean of Group B) / Pooled Standard Deviation
Fixed-Effect vs. Random-Effects Models
In meta-analysis, you can choose between fixed-effect and random-effects models to combine the results of individual studies:
Fixed-Effect Model:
- Assumes that all included studies share a common true effect size.
- Accounts for only within-study variability (sampling error).
- Appropriate when studies are very similar or when there's minimal heterogeneity.
Random-Effects Model:
- Acknowledges that there may be variability in effect sizes across studies.
- Accounts for both within-study variability (sampling error) and between-study variability (real differences between studies).
- More conservative and applicable when there's substantial heterogeneity.
The choice between these models should be guided by the degree of heterogeneity observed among the included studies. If heterogeneity is significant, the random-effects model is often preferred, as it provides a more robust estimate of the overall effect.
Forest Plots
Forest plots are graphical representations commonly used in meta-analysis to display the results of individual studies along with the combined summary estimate. Key components of a forest plot include:
- Vertical Line: Represents the null effect (e.g., no difference or no effect).
- Horizontal Lines: Represent the confidence intervals for each study's effect size estimate.
- Diamond or Square: Represents the summary effect size estimate, with its width indicating the confidence interval around the summary estimate.
- Study Names: Listed on the left side of the plot, identifying each study.
Forest plots help visualize the distribution of effect sizes across studies and provide insights into the consistency and direction of the findings.
Heterogeneity Assessment
Heterogeneity refers to the variability in effect sizes among the included studies. It's important to assess and understand heterogeneity as it can impact the interpretation of your meta-analysis results. Standard methods for assessing heterogeneity include:
- Cochran's Q Test: A statistical test that assesses whether there is significant heterogeneity among the effect sizes of the included studies.
- I² Statistic: A measure that quantifies the proportion of total variation in effect sizes that is due to heterogeneity. I² values range from 0% to 100%, with higher values indicating greater heterogeneity.
Assessing heterogeneity is crucial because it informs your choice of meta-analysis model (fixed-effect vs. random-effects) and whether subgroup analyses or sensitivity analyses are warranted to explore potential sources of heterogeneity.
How to Interpret Meta-Analysis Results?
With the data synthesis complete, it's time to make sense of the results of your meta-analysis.
Meta-Analytic Summary
The meta-analytic summary is the culmination of your efforts in data synthesis. It provides a consolidated estimate of the effect size and its confidence interval, combining the results of all included studies. To interpret the meta-analytic summary effectively:
- Effect Size Estimate: Understand the primary effect size estimate, such as Cohen's d, odds ratio, or hazard ratio, and its associated confidence interval.
- Significance: Determine whether the summary effect size is statistically significant. This is indicated when the confidence interval does not include the null value (e.g., 0 for Cohen's d or 1 for odds ratio).
- Magnitude: Assess the magnitude of the effect size. Is it large, moderate, or small, and what are the practical implications of this magnitude?
- Direction: Consider the direction of the effect. Is it in the hypothesized direction, or does it contradict the expected outcome?
- Clinical or Practical Significance: Reflect on the clinical or practical significance of the findings. Does the effect size have real-world implications?
- Consistency: Evaluate the consistency of the findings across studies. Are most studies in agreement with the summary effect size estimate, or are there outliers?
Subgroup Analyses
Subgroup analyses allow you to explore whether the effect size varies across different subgroups of studies or participants. This can help identify potential sources of heterogeneity or assess whether the intervention's effect differs based on specific characteristics. Steps for conducting subgroup analyses:
- Define Subgroups: Clearly define the subgroups you want to investigate based on relevant study characteristics (e.g., age groups, study design, intervention type).
- Analyze Subgroups: Calculate separate summary effect sizes for each subgroup and compare them to the overall summary effect.
- Assess Heterogeneity: Evaluate whether subgroup differences are statistically significant. If so, this suggests that the effect size varies significantly among subgroups.
- Interpretation: Interpret the subgroup findings in the context of your research question. Are there meaningful differences in the effect across subgroups? What might explain these differences?
Subgroup analyses can provide valuable insights into the factors influencing the overall effect size and help tailor recommendations for specific populations or conditions.
Sensitivity Analyses
Sensitivity analyses are conducted to assess the robustness of your meta-analysis results by exploring how different choices or assumptions might affect the findings. Common sensitivity analyses include:
- Exclusion of Low-Quality Studies: Repeating the meta-analysis after excluding studies with low quality or a high risk of bias.
- Changing Effect Size Measure: Re-running the analysis using a different effect size measure to assess whether the choice of measure significantly impacts the results.
- Publication Bias Adjustment: Applying methods like the trim-and-fill procedure to adjust for potential publication bias.
- Subsample Analysis: Analyzing a subset of studies based on specific criteria or characteristics to investigate their impact on the summary effect.
Sensitivity analyses help assess the robustness and reliability of your meta-analysis results, providing a more comprehensive understanding of the potential influence of various factors.
Reporting and Publication
The final stages of your meta-analysis involve preparing your findings for publication.
Manuscript Preparation
When preparing your meta-analysis manuscript, consider the following:
- Structured Format: Organize your manuscript following a structured format, including sections such as introduction, methods, results, discussion, and conclusions.
- Clarity and Conciseness: Write your findings clearly and concisely, avoiding jargon or overly technical language. Use tables and figures to enhance clarity.
- Transparent Methods: Provide detailed descriptions of your methods, including eligibility criteria, search strategy, data extraction, and statistical analysis.
- Incorporate Tables and Figures: Present your meta-analysis results using tables and forest plots to visually convey key findings.
- Interpretation: Interpret the implications of your findings, discussing the clinical or practical significance and limitations.
Transparent Reporting Guidelines
Adhering to transparent reporting guidelines ensures that your meta-analysis is transparent, reproducible, and credible. Some widely recognized guidelines include:
- PRISMA (Preferred Reporting Items for Systematic Reviews and Meta-Analyses): PRISMA provides a checklist and flow diagram for reporting systematic reviews and meta-analyses, enhancing transparency and rigor.
- MOOSE (Meta-analysis of Observational Studies in Epidemiology): MOOSE guidelines are designed for meta-analyses of observational studies and provide a framework for transparent reporting.
- ROBINS-I: If your meta-analysis involves non-randomized studies, follow the Risk Of Bias In Non-randomized Studies of Interventions guidelines for reporting.
Adhering to these guidelines ensures that your meta-analysis is transparent, reproducible, and credible. It enhances the quality of your research and aids readers and reviewers in assessing the rigor of your study.
PRISMA Statement
The PRISMA statement is a valuable resource for conducting and reporting systematic reviews and meta-analyses. Key elements of PRISMA include:
- Title: Clearly indicate that your paper is a systematic review or meta-analysis.
- Structured Abstract: Provide a structured summary of your study, including objectives, methods, results, and conclusions.
- Transparent Reporting: Follow the PRISMA checklist, which covers items such as the rationale, eligibility criteria, search strategy, data extraction, and risk of bias assessment.
- Flow Diagram: Include a flow diagram illustrating the study selection process.
By adhering to the PRISMA statement, you enhance the transparency and credibility of your meta-analysis, facilitating its acceptance for publication and aiding readers in evaluating the quality of your research.
Conclusion for Meta-Analysis
Meta-analysis is a powerful tool that allows you to combine and analyze data from multiple studies to find meaningful patterns and make informed decisions. It helps you see the bigger picture and draw more accurate conclusions than individual studies alone. Whether you're in healthcare, education, business, or any other field, the principles of meta-analysis can be applied to enhance your research and decision-making processes.
Remember that conducting a successful meta-analysis requires careful planning, attention to detail, and transparency in reporting. By following the steps outlined in this guide, you can embark on your own meta-analysis journey with confidence, contributing to the advancement of knowledge and evidence-based practices in your area of interest.
How to Elevate Your Meta-Analysis With Real-Time Insights?
Introducing Appinio, the real-time market research platform that brings a new level of excitement to your meta-analysis journey. With Appinio, you can seamlessly collect your own market research data in minutes, empowering your meta-analysis with fresh, real-time consumer insights.
Here's why Appinio is your ideal partner for efficient data collection:
- From Questions to Insights in Minutes: Appinio's lightning-fast platform ensures you get the answers you need when you need them, accelerating your meta-analysis process.
- No Research PhD Required: Our intuitive platform is designed for everyone, eliminating the need for specialized research skills and putting the power of data collection in your hands.
- Global Reach, Minimal Time: With an average field time of less than 23 minutes for 1,000 respondents and access to over 90 countries, you can define precise target groups and gather data swiftly.
Join the loop 💌
Be the first to hear about new updates, product news, and data insights. We'll send it all straight to your inbox.