What is Sampling Error? Definition, Types, Examples
Appinio Research · 28.03.2024 · 28min read
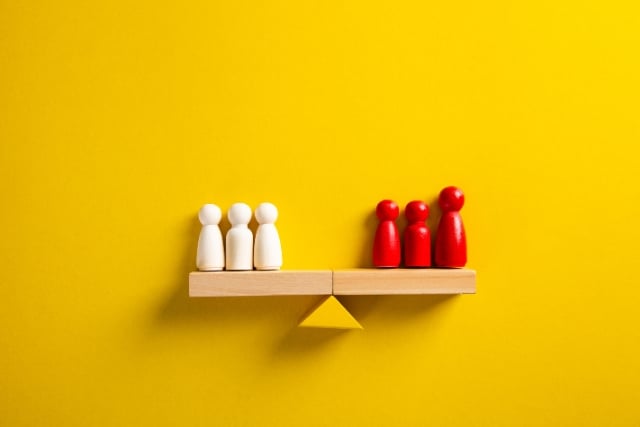
Content
Have you ever wondered why survey results sometimes differ from reality or why estimates based on a sample don't always match the true characteristics of a population? Sampling error holds the answer. In statistics, sampling error is like a hidden force that influences the accuracy of research findings and the reliability of data analysis. It's the unavoidable discrepancy that arises when we analyze data from a subset of a larger population rather than the entire population itself. Understanding sampling error is crucial for anyone working with data, whether you're a researcher, a policymaker, or a business analyst.
What is Sampling Error?
Sampling error is a fundamental concept in statistics that refers to the discrepancy between a sample statistic and the true population parameter it aims to estimate. It arises from the fact that we are observing only a subset of the population rather than the entire population itself. Understanding the nature and implications of sampling error is essential for researchers, analysts, and decision-makers across various fields.
Sampling error encompasses random fluctuations that occur when different samples are drawn from the same population. It reflects the variability inherent in the sampling process and impacts the accuracy and reliability of research findings. By recognizing and quantifying sampling errors, you can assess the precision of their estimates and make informed decisions based on the reliability of the data.
Importance of Understanding Sampling Error
Understanding sampling error is crucial for several reasons:
- Validity of Inferences: Sampling error directly affects the validity of statistical inferences drawn from sample data. Researchers must recognize the potential for error and assess its impact on the reliability of research findings.
- Precision of Estimates: Sampling error quantifies the uncertainty associated with sample estimates of population parameters. Recognizing the magnitude of sampling error helps researchers gauge the accuracy of their estimates and establish confidence intervals around them.
- Data Quality Assurance: Awareness of sampling error prompts researchers to implement appropriate sampling techniques and validation procedures to minimize error and ensure the quality and integrity of research data.
- Decision-Making Confidence: Decision-makers rely on accurate and reliable data to make informed decisions. Understanding sampling error provides decision-makers with insights into data reliability and enhances their confidence in using research findings to inform policies, strategies, and actions.
By grasping the concept of sampling error and its implications, you can navigate the complexities of data analysis with greater confidence and rigor. It empowers you to produce robust research outcomes and contribute to evidence-based decision-making processes across diverse domains.
Types of Sampling Error
Sampling error can take various forms, each with unique characteristics and implications for data analysis. Understanding these types is crucial for effectively addressing and mitigating their impact on research outcomes.
Random Sampling Error
Random sampling error occurs when the sample selected for analysis is not perfectly representative of the entire population due to chance. Despite careful selection procedures, there is always a degree of randomness inherent in sampling. This randomness can lead to fluctuations in sample characteristics compared to the population parameters.
Example:
Suppose you're conducting a survey on voting preferences in a city. You randomly select 500 individuals from the voter registry to participate. However, due to chance, your sample ends up with slightly more young voters compared to the population. This discrepancy is a result of a random sampling error.
To minimize random sampling error:
- Increase Sample Size: Larger samples reduce the impact of random fluctuations, leading to more reliable estimates of population parameters.
- Randomization Techniques: Employ randomization methods such as simple random sampling or stratified random sampling to ensure every member of the population has an equal chance of being included in the sample.
Systematic Sampling Error
Systematic sampling error occurs when there is a consistent bias in the selection process, leading to results that consistently overestimate or underestimate the true population parameters. Unlike random sampling error, which is due to chance, systematic error arises from flaws in the sampling methodology or data collection process.
Example:
Imagine you're conducting a survey on household income levels in a country. Instead of randomly selecting households, you only survey individuals from urban areas, inadvertently excluding rural populations. As a result, your sample systematically underrepresents low-income households, leading to an overestimation of average income levels.
To mitigate systematic sampling error:
- Diversify Sampling Methods: Use a combination of sampling techniques (e.g., stratified sampling, cluster sampling) to ensure a more representative sample.
- Validate Sampling Frame: Thoroughly assess the sampling frame to ensure it accurately reflects the entire population, addressing any biases or omissions.
Non-Sampling Error
Non-sampling error encompasses errors that are not directly related to the sampling process but can still impact the accuracy of research findings. These errors can arise from various sources, including data collection, measurement, and processing.
Non-sampling errors can manifest in different forms:
- Measurement Error: Inaccuracies or inconsistencies in measuring variables of interest, leading to distorted results.
- Selection Bias: Systematic differences between individuals or units included in the sample and those excluded, resulting in biased estimates.
- Non-Response Bias: This occurs when individuals chosen for the sample do not respond to the survey, potentially skewing the results.
- Coverage Error: Arises when certain segments of the population are not adequately represented in the sampling frame, leading to a biased sample.
Understanding and distinguishing between sampling and non-sampling errors is essential for accurately interpreting research findings and implementing appropriate corrective measures. While sampling errors can be minimized through careful sampling techniques, addressing non-sampling errors often requires rigorous validation procedures and data quality checks throughout the research process.
Sources of Sampling Error
Understanding sampling error sources is crucial for conducting reliable research and making informed decisions based on data analysis. Let's delve into the various factors that can contribute to sampling error and explore how they impact the validity of research findings.
Sampling Frame Error
Sampling frame error occurs when the sampling frame, which represents the list of the population from which the sample is drawn, does not accurately reflect the entire population. This discrepancy between the sampling frame and the population can lead to biased or non-representative samples, undermining the validity of research outcomes.
Example:
Suppose you're conducting a study on internet usage habits among adults aged 18-65 in a country. However, your sampling frame consists only of individuals with internet access, excluding those without access. As a result, your sample fails to capture the behaviors and characteristics of the entire population, leading to sampling frame error.
To address sampling frame error:
- Evaluate Sampling Frame: Thoroughly assess the sampling frame to ensure it adequately represents the target population, considering factors such as demographics, geographic location, and accessibility.
- Expand Sampling Frame: If necessary, expand the sampling frame to include overlooked segments of the population, ensuring a more comprehensive representation.
Selection Bias
Selection bias occurs when specific population segments are systematically excluded or underrepresented in the sample, leading to biased estimates of population parameters. This bias can arise from factors such as non-random sampling methods, self-selection, or researcher bias, resulting in distorted research outcomes.
Example:
In a study on the effectiveness of a new educational program, participants are recruited through voluntary sign-ups at schools. However, individuals who choose to participate may have different characteristics or motivations compared to those who do not, leading to selection bias. The resulting sample may not be representative of the entire population of interest, affecting the generalizability of the findings.
To mitigate selection bias:
- Employ Random Sampling: Use random sampling methods such as simple random sampling or stratified random sampling to ensure every member of the population has an equal chance of being included in the sample.
- Minimize Self-Selection: Implement strategies to minimize self-selection bias, such as offering incentives for participation or ensuring anonymity to encourage honest responses.
Measurement Error
Measurement error arises from inaccuracies or inconsistencies in the measurement process, leading to erroneous data. This error can occur due to various factors, including instrument limitations, human error, respondent bias, or ambiguous survey questions.
Example:
In a survey measuring customer satisfaction, respondents may interpret the survey questions differently or provide socially desirable responses, leading to measurement error. Additionally, technical issues with the survey platform or data collection instruments can introduce measurement errors, affecting the reliability of the results.
To minimize measurement error:
- Pilot Test Instruments: Conduct pilot tests or pre-tests of survey instruments to identify and address any ambiguities or misunderstandings in the questions.
- Train Data Collectors: Provide adequate training to data collectors to ensure consistency in administering surveys and recording responses.
- Validate Instruments: Validate measurement instruments through comparison with established measures or through reliability and validity testing.
Non-Response Bias
Non-response bias occurs when individuals selected for the sample do not respond to the survey or study, leading to a biased sample. This bias can arise due to factors such as survey fatigue, lack of interest, or demographic characteristics associated with non-response.
Example:
In a customer satisfaction survey sent via email, only 30% of recipients respond, with older customers being less likely to participate. As a result, the survey results may overrepresent the views of younger customers, leading to non-response bias and inaccurate conclusions about overall satisfaction levels.
To address non-response bias:
- Follow-Up Surveys: Implement follow-up surveys or reminders to encourage participation and improve response rates.
- Analyze Non-Response Patterns: Analyze characteristics of non-respondents to identify potential biases and adjust sample weights or sampling strategies accordingly.
Coverage Error
Coverage error occurs when the sampling frame does not include all members of the target population, resulting in a biased sample. This error can arise from incomplete or outdated sampling frames, inadequate coverage of certain population segments, or limitations in data collection methods.
Example:
In a study on smartphone usage among teenagers, the sampling frame consists of registered high school students. However, this sampling frame excludes teenagers who are homeschooled or not enrolled in traditional schooling, leading to coverage error. The resulting sample may not accurately represent the diversity of teenage smartphone users.
To minimize coverage error:
- Update Sampling Frames: Regularly update sampling frames to reflect changes in the population and ensure comprehensive coverage.
- Utilize Multiple Data Sources: Supplement sampling frames with additional data sources, such as census data or administrative records, to capture overlooked population segments.
Understanding and addressing these sampling error sources is essential for ensuring the reliability and validity of research findings. By implementing appropriate strategies and validation procedures, you can minimize the impact of sampling error and produce more accurate insights into the target population.
Examples of Sampling Errors
Understanding sampling error through real-world examples can provide valuable insights into its effects on research outcomes and decision-making processes. Let's explore a range of examples that illustrate different scenarios where sampling errors may arise.
Example 1: Political Polling
Consider a scenario where a polling organization conducts a survey to estimate the proportion of voters supporting a particular candidate in an upcoming election. Due to limitations in resources and time, the organization selects a random sample of registered voters from a specific geographic region. However, the sample inadvertently overrepresents urban areas and underrepresents rural areas.
Effect: The survey results may reflect a higher level of support for the candidate than what exists in the entire population. This discrepancy arises from sampling error, as the sample fails to accurately represent the demographic diversity and voting preferences of the entire electorate.
Example 2: Quality Control in Manufacturing
In a manufacturing plant, quality control inspectors conduct random inspections of finished products to assess their compliance with quality standards. However, due to time constraints, inspectors tend to focus more on products from certain production lines or shifts.
Effect: Sampling error may occur if products from certain production lines or shifts exhibit different quality characteristics than those from others. As a result, the sampled products may not accurately represent the overall quality of the entire production process, leading to biased quality assessments and potentially overlooking quality issues.
Example 3: Public Health Surveys
A public health agency conducts a survey to estimate the prevalence of a specific health condition in a community. The agency randomly selects households from a list of residential addresses and invites residents to participate in the survey. However, some residents decline to participate due to privacy concerns or other reasons.
Effect: Non-response bias may introduce sampling error if the individuals who decline to participate differ systematically from those who agree to participate. Depending on the characteristics of non-respondents, the survey results may underestimate or overestimate the true prevalence of the health condition in the community.
Example 4: Market Research
A market research firm conducts a survey to gather feedback on a new product launch. The firm distributes online surveys to a random sample of customers who have purchased similar products in the past. However, respondents who choose to participate may have stronger opinions or different purchasing behaviors than those who do not participate.
Effect: Self-selection bias may lead to sampling error if the opinions and behaviors of survey respondents differ systematically from those of non-respondents. The survey results may overstate or understate the level of interest or satisfaction with the new product, affecting the validity of market research insights.
These examples demonstrate the diverse contexts where sampling error can occur and its potential implications for research outcomes and decision-making processes. By recognizing and understanding sampling error in practice, researchers and practitioners can take proactive measures to minimize its impact and enhance the reliability and validity of their findings.
Tools for Managing Sampling Error
Access to appropriate tools and resources is crucial for effectively managing sampling error and ensuring the reliability of research outcomes. Let's explore various tools and resources available to address sampling errors.
Statistical Software
Statistical software packages provide powerful tools for analyzing data and addressing sampling error through advanced statistical techniques. Here are some commonly used statistical software options:
- SPSS (Statistical Package for the Social Sciences): SPSS offers a user-friendly interface and a wide range of statistical procedures for data analysis, including descriptive statistics, hypothesis testing, and regression analysis.
- R: R is a free, open-source programming language and software environment for statistical computing and graphics. It offers a vast collection of packages for conducting sophisticated statistical analyses and addressing sampling errors.
- Python: Python, with libraries such as NumPy, pandas, and SciPy, provides robust capabilities for data manipulation, analysis, and visualization. It is widely used in data science and research to address sampling errors and other statistical challenges.
These statistical software packages offer a variety of functions and tools for estimating sampling error, conducting hypothesis tests, and implementing advanced statistical techniques to account for sampling variability.
Sample Size Calculators
Ensuring your survey results accurately represent the population is essential. With the Appinio Sample Size Calculator, you can calculate the minimum sample size needed for representative outcomes based on factors like margin of error, confidence level, and standard deviation.
- Determine representative sample sizes effortlessly: Input your desired margin of error, confidence level, and standard deviation to calculate the minimum sample size required for accurate results.
- Confidence in your data: Using the Sample Size Calculator, you can ensure that your survey results are statistically valid and reliable, minimizing the risk of sampling error.
By utilizing the Sample Size Calculator, you can optimize their survey designs and ensure the reliability and accuracy of their research outcomes.
Data Collection and Survey Tools
Effective data collection and survey tools play a crucial role in minimizing sampling error and ensuring the quality of research data. Appinio offers a comprehensive platform for creating, distributing, and analyzing surveys, providing you with advanced features and capabilities to streamline the research process and obtain accurate insights.
Here's why Appinio stands out among data collection and survey tools:
- Seamless survey creation and distribution: With Appinio's intuitive interface, you can create customized surveys tailored to their specific research objectives and target audience, ensuring relevance and accuracy in data collection.
- Robust data analysis and visualization: Appinio provides powerful analytics tools and interactive reports that enable you to quickly analyze survey responses, identify trends, and generate actionable insights.
- Extensive targeting options: With access to over 1200 characteristics, you can define their target groups precisely and survey them in more than 90 countries, ensuring representative samples and minimizing sampling error.
By leveraging Appinio's platform, you can streamline the data collection process, minimize sampling error, and produce reliable and valid research outcomes. With features designed to enhance efficiency and accuracy, Appinio empowers you to make data-driven decisions with confidence.
By leveraging these tools and resources, you can effectively manage sampling errors, enhance the quality of your research, and produce reliable and valid research outcomes. Choosing tools that align with the specific research objectives, methodologies, and data analysis requirements is essential to optimize research efficiency and accuracy.
Sampling Error Impact and Consequences
Sampling error can have profound implications for data analysis, statistical inference, and decision-making processes. Understanding the impact and consequences of sampling error is essential for interpreting research findings accurately and making informed decisions based on the data.
Effects on Data Accuracy
Sampling error directly affects the accuracy of data collected through sampling methods. When sampling error is present, the characteristics and estimates derived from the sample may deviate from the actual population parameters. This deviation introduces uncertainty into the data and can lead to misleading conclusions about the population as a whole.
- Estimate Confidence Intervals: Calculate confidence intervals around sample estimates to quantify the range of potential error and assess the precision of estimates.
- Compare Sample Characteristics: Compare the characteristics of the sample to known population parameters to identify potential biases or discrepancies.
Example:
In a study measuring the average income of households in a city, sampling error may lead to overestimation or underestimation of the actual average income. If the sample includes disproportionately more high-income households, the average income calculated from the sample may be higher than the actual average income of the entire population.
Influence on Statistical Inference
Sampling error plays a significant role in statistical inference, which involves drawing conclusions about a population based on sample data. Biased or non-representative samples due to sampling error can lead to incorrect inferences about population parameters, undermining the validity of statistical analyses.
- Use Robust Statistical Methods: Employ statistical techniques that are less sensitive to the effects of sampling error, such as bootstrapping or robust regression methods.
- Sensitivity Analysis: Conduct sensitivity analyses to assess the robustness of statistical conclusions to variations in sample composition or size.
Example:
In hypothesis testing, sampling error can influence the interpretation of statistical significance. If a sample is biased towards a particular group or characteristic, statistical tests may erroneously detect significance where none exists, or fail to detect true effects due to insufficient representation.
Implications for Decision-Making
Sampling error can have far-reaching implications for decision-making processes in various domains, including business, policy, and academia. Decisions based on flawed or biased data can lead to ineffective strategies, misallocation of resources, or misguided policies.
- Consider Margin of Error: Take into account the margin of error associated with sample estimates when making decisions based on survey or research findings.
- Validate Findings: Validate research findings through independent replication or triangulation with additional data sources to ensure robustness and reliability.
Example:
In market research, sampling error can impact product development and marketing strategies. If a survey sample fails to capture the preferences of a key demographic segment, decisions based on that data may result in products that do not resonate with the target audience, leading to missed opportunities and reduced profitability.
By recognizing the effects of sampling error on data accuracy, statistical inference, and decision-making, researchers and practitioners can take proactive measures to mitigate its impact and improve the reliability of research outcomes. Incorporating uncertainty assessments and validation procedures into research protocols enhances the credibility and usefulness of findings for informed decision-making.
How to Minimize Sampling Error?
Minimizing sampling error is essential for obtaining reliable and accurate data that can inform robust research outcomes and decision-making processes. Implementing effective strategies throughout the sampling process helps mitigate the risk of sampling error and improves the validity of research findings.
Proper Sampling Techniques
Proper sampling techniques are fundamental to reducing sampling error and ensuring the representativeness of the sample. Consider the following methods:
- Random Sampling: Use random sampling methods, such as simple random sampling or stratified random sampling, to ensure that every member of the population has an equal chance of being included in the sample.
- Stratification: Divide the population into homogeneous strata based on relevant characteristics (e.g., age, gender, income) and then sample proportionally from each stratum to ensure adequate representation of diverse subgroups.
- Cluster Sampling: Divide the population into clusters (e.g., geographic areas) and randomly select clusters to sample from. Then, sample all individuals within the selected clusters.
Increasing Sample Size
Increasing the sample size can help reduce the impact of sampling error by providing more reliable estimates of population parameters. To increase sample size:
- Power Analysis: Conduct power analysis to determine the minimum sample size required to detect meaningful effects or differences with sufficient statistical power.
- Sampling Efficiency: Strive to achieve the largest sample size feasible within practical constraints, balancing considerations such as cost, time, and resources.
Utilizing Randomization
Randomization techniques help minimize systematic biases and ensure the representativeness of the sample.
- Random Assignment: Randomly assign participants to treatment groups or conditions in experimental studies to distribute potential confounding variables evenly across groups.
- Random Selection: Randomly select individuals or units from the sampling frame to minimize selection bias and ensure the randomness of the sample.
Addressing Non-Response Bias
Non-response bias can significantly impact the validity of research findings, particularly in survey research. Addressing non-response bias requires proactive measures to encourage participation and mitigate its effects.
- Follow-Up Surveys: Implement follow-up surveys or reminders to non-respondents to encourage participation and improve response rates.
- Incentives: Offer incentives, such as monetary rewards or gift cards, to motivate participation and reduce non-response bias.
- Sensitive Recruitment: Use personalized and targeted recruitment strategies to engage hard-to-reach populations and minimize non-response bias.
Enhancing Measurement Accuracy
Measurement accuracy is crucial for minimizing data collection errors and ensuring research findings' reliability. Enhance measurement accuracy through the following approaches:
- Standardized Instruments: Use standardized measurement instruments and protocols to ensure consistency and reliability in data collection across different settings.
- Pilot Testing: Conduct pilot testing of survey instruments or data collection protocols to identify and address potential sources of measurement error before full-scale implementation.
- Training and Calibration: Provide training and calibration sessions for data collectors to improve measurement accuracy and reduce inter-rater variability.
By incorporating these strategies into the sampling process, you can minimize sampling error and enhance the validity and reliability of your research findings. Tailoring sampling strategies to the specific research context and objectives while considering practical constraints and ethical considerations is essential. Regular monitoring and validation of sampling procedures help ensure the integrity and robustness of research outcomes.
Conclusion for Sampling errors
Sampling error is a critical concept to grasp for anyone working with data. It's the reason why survey results may not always perfectly reflect reality and why estimates based on samples can sometimes be off the mark. By understanding the nature of sampling error and its potential sources, impacts, and mitigation strategies, you'll be better equipped to interpret research findings accurately and make informed decisions based on reliable data. Remember, while sampling error is inevitable, being aware of its presence allows you to take proactive steps to minimize its impact and ensure the validity and reliability of your data analysis.
Furthermore, sampling error underscores the importance of sound research methodology and careful consideration of sampling techniques. By implementing proper sampling methods, increasing sample sizes where feasible, and utilizing randomization techniques, researchers can minimize the effects of sampling error and produce more robust and reliable research outcomes.
Leveraging tools such as statistical software, sampling calculators, and data collection platforms can help manage sampling errors effectively. Ultimately, by embracing best practices and staying vigilant in addressing sampling errors, you can enhance the credibility and utility of your research findings, contributing to evidence-based decision-making and advancing knowledge in your field.
How to Avoid Sampling Error?
Introducing Appinio, the real-time market research platform revolutionizing how companies gather consumer insights. Say goodbye to sampling error and hello to fast, accurate, data-driven decisions. Here's why you'll love using Appinio:
- From questions to insights in minutes: Conduct your own market research effortlessly and get actionable insights in no time.
- No research expertise required: Our intuitive platform means anyone can use it; no PhD in research needed.
- Reach your target audience worldwide: Define your target group from over 1200 characteristics and survey them in more than 90 countries.
Join the loop 💌
Be the first to hear about new updates, product news, and data insights. We'll send it all straight to your inbox.