What is Trend Analysis? Definition, Formula, Examples
Appinio Research · 13.02.2024 · 38min read
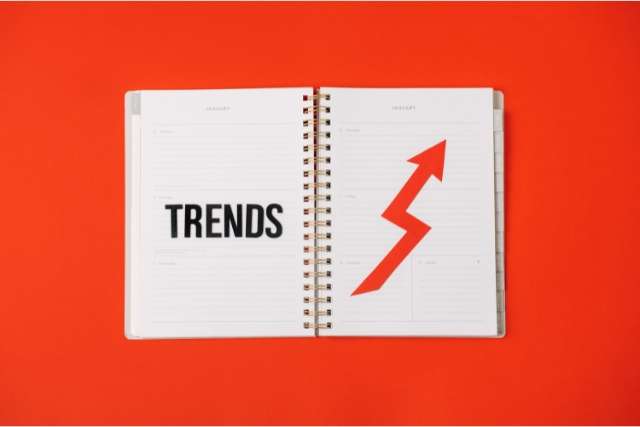
Content
Have you ever wondered how to uncover hidden insights within your data, predict future trends, and make informed decisions that can steer your business or projects toward success? In this guide on trend analysis, we'll unravel the intricacies of this powerful tool, helping you navigate the world of data patterns, forecasts, and informed strategies.
Whether you're a data scientist, a business analyst, or simply curious about understanding and leveraging trends, this guide will equip you with the knowledge and techniques to harness the potential of trend analysis to your advantage.
What is Trend Analysis?
Trend analysis is a statistical technique used to identify and analyze patterns or trends in data over time. It involves examining historical data to uncover insights into past trends and predict future developments. Understanding the components of trend analysis is essential for conducting effective analysis:
Components of Trend Analysis
- Trend: The overall direction in which data is moving over time. Trends can be upward (positive), downward (negative), or flat (no significant change).
- Seasonality: Regular, predictable fluctuations in data that occur at fixed intervals, such as daily, weekly, or yearly patterns.
- Cyclical Patterns: Longer-term fluctuations in data that occur over multiple years, often driven by economic cycles or other external factors.
- Irregular or Random Fluctuations: Unpredictable variations in data that do not follow a discernible pattern. These fluctuations may be due to random events or measurement errors.
Understanding these components allows analysts to differentiate between various types of trends and apply appropriate methods for analysis.
Importance of Trend Analysis
Trend analysis is a crucial tool for decision-making and planning across diverse fields. Here are several reasons why trend analysis is essential:
- Strategic Planning: Trend analysis helps organizations identify emerging opportunities and threats, guiding strategic planning and resource allocation.
- Risk Management: By identifying trends and potential future scenarios, trend analysis enables organizations to mitigate risks and adapt to changing market conditions.
- Performance Evaluation: Trend analysis allows organizations to assess their performance over time, track progress toward goals, and identify areas for improvement.
- Forecasting: Trend analysis provides insights into future trends and developments, helping organizations anticipate changes and make proactive decisions.
- Resource Optimization: By understanding trends in demand, resource utilization, and consumer behavior, organizations can optimize operations and allocate resources efficiently.
- Informed Decision-Making: Trend analysis provides decision-makers with data-driven insights, reducing uncertainty and enabling informed decision-making.
- Competitive Advantage: Organizations that effectively utilize trend analysis gain a competitive advantage by staying ahead of market trends and customer preferences.
- Continuous Improvement: Trend analysis fosters a culture of continuous improvement by encouraging organizations to monitor performance, learn from past trends, and adapt strategies accordingly.
Overall, trend analysis is an indispensable tool for organizations seeking to navigate a dynamic and ever-changing environment effectively. By understanding past trends and anticipating future developments, organizations can position themselves for success and achieve their objectives.
Data Collection for Trend Analysis
In trend analysis, the journey begins with effectively collecting and managing your data. Your ability to make accurate predictions and draw meaningful insights heavily relies on the quality and relevance of the data you collect. Here's a closer look at the critical steps involved in this process.
Identifying Relevant Data Sources
Before you embark on any trend analysis, it's essential to pinpoint the most pertinent data sources for your specific objectives. This step requires a deep understanding of your subject matter and a keen eye for potential data goldmines. Consider the following when identifying data sources:
- Internal Data: Start by looking within your organization. This could include databases, CRM systems, financial records, or historical sales data. Internal data is often readily accessible and can provide valuable insights.
- External Data: Expand your horizons by exploring external data sources. Depending on your analysis goals, these might encompass public datasets, industry reports, social media data, economic indicators, or even weather data.
- Surveys and Feedback: If your analysis pertains to customer behavior or opinions, consider conducting surveys, interviews, or collecting feedback directly from your target audience. Qualitative data can be invaluable.
- Web Scraping: In the digital age, web scraping tools can be used to gather data from websites, forums, or online reviews, providing a wealth of information for analysis.
As you navigate the complexities of data collection for trend analysis, consider the seamless integration of Appinio into your research toolkit.
With its intuitive platform and global reach, Appinio streamlines the gathering of real-time consumer insights, ensuring you have the data you need to drive informed decisions. Embrace the power of Appinio to unlock a world of possibilities in trend analysis.
Ready to experience the future of market research? Book a demo today and see how Appinio can revolutionize your approach to data-driven decision-making!
Data Gathering and Preparation
Once you've identified your data sources, the next step is to collect and prepare the data for analysis. This process involves several crucial tasks:
- Data Cleaning: Raw data is often messy, containing errors, duplicates, missing values, and outliers. Data cleaning involves rectifying these issues to ensure the accuracy and integrity of your dataset.
- Data Transformation: Depending on your analysis goals, you may need to transform your data. This could involve aggregating data over time periods, converting units, or normalizing variables to make them comparable.
- Data Integration: If you're using data from multiple sources, integrate it into a single dataset. This requires matching and merging data based on common identifiers.
- Data Documentation: Keep detailed records of your data collection and preparation process. This documentation is invaluable for transparency and reproducibility.
Data Quality Assurance
Data quality is paramount in trend analysis. Poor-quality data can lead to erroneous conclusions and unreliable predictions. To ensure data quality, implement the following practices:
- Data Validation: Validate your data against predefined criteria to identify inconsistencies or errors. This includes checking for data type mismatches, range validations, and logical validations.
- Outlier Detection: Use statistical methods to identify outliers that may distort your analysis. Decide whether to remove, transform, or investigate these outliers based on their impact.
- Data Consistency: Ensure consistency in data formats, units, and measurements. Inconsistent data can lead to misinterpretation.
- Data Security and Privacy: Protect sensitive data through encryption and access controls. Compliance with data privacy regulations, such as GDPR or HIPAA, is crucial.
- Data Governance: Establish data governance policies and procedures within your organization to maintain data quality over time. This includes assigning responsibilities for data quality maintenance and documentation.
By diligently following these data collection and quality assurance steps, you set a solid foundation for meaningful trend analysis, allowing you to extract valuable insights confidently.
Types of Trends
Trend analysis is a versatile tool that can be applied to various types of data, depending on your specific objectives and the nature of the information you're working with. Understanding the different types of trends is crucial for tailoring your analysis approach.
Time Series Trends
Time series trends are perhaps the most familiar and widely used type of trend analysis. This category focuses on data points collected sequentially over time. Time series data can exhibit various patterns and behaviors, including:
- Trends: These are long-term movements in data, indicating a consistent upward or downward direction. For example, monthly sales data for a retail store may exhibit an upward trend if sales are gradually increasing over several years.
- Seasonal Patterns: Seasonality involves repeating patterns within a specific time frame. For instance, ice cream sales tend to rise during the summer and drop during the winter.
- Cyclic Patterns: Cyclic patterns are longer-term fluctuations that don't have fixed durations. They often result from economic cycles and can be challenging to predict accurately.
- Random Noise: Random noise represents unpredictable variations in data. It's essential to filter out noise to identify meaningful trends.
Analyzing time series trends involves techniques like moving averages, exponential smoothing, and autoregressive models (ARIMA). These methods help extract underlying trends and patterns from noisy time series data, facilitating better predictions and decision-making.
Cross-Sectional Trends
Cross-sectional trends, on the other hand, focus on data collected at a single point in time, often comparing different entities or groups. This type of analysis is prevalent in market research, social sciences, and many other fields.
- Comparative Analysis: Cross-sectional analysis allows you to compare different groups or entities at a specific moment. For instance, you might analyze the salaries of employees across various departments within a company to identify disparities or trends.
- Demographic Studies: In demographic research, cross-sectional data can reveal trends in population characteristics, such as income distribution, education levels, or healthcare access.
- Market Segmentation: In marketing, cross-sectional trends help identify consumer preferences and segment markets based on various attributes like age, gender, or location.
Analyzing cross-sectional trends often involves descriptive statistics, hypothesis testing, and data visualization techniques like bar charts, pie charts, and histograms to compare and contrast different groups.
Longitudinal Trends
Longitudinal trends, also known as panel data analysis, focus on changes within individual entities or subjects over an extended period. This type of analysis is prevalent in fields like healthcare, education, and social sciences. Here's a closer look at longitudinal trends:
- Individual Tracking: Longitudinal studies track the same subjects or entities over time to observe changes. For instance, a medical study may follow patients over several years to assess the effectiveness of a treatment.
- Growth and Development: Longitudinal analysis can reveal patterns of growth, development, or decline within individuals or entities. This is vital in understanding human development, product lifecycle, or organizational evolution.
- Event Impact: It allows for the evaluation of how specific events or interventions affect subjects over time. For example, assessing the long-term impact of an educational program on student performance.
Analyzing longitudinal trends often involves statistical methods like growth curve modeling, repeated measures analysis, and mixed-effects models to account for individual variations and changes over time.
Understanding these distinct types of trends equips you with the knowledge needed to choose the appropriate analysis methods and techniques based on your data and objectives. Whether you're dealing with time series, cross-sectional, or longitudinal data, the insights gained from trend analysis can drive informed decision-making and strategy development in various domains.
Trend Analysis Methods
Now that you have a solid foundation in understanding the types of trends, it's time to delve deeper into the various methods used for trend analysis. These methods serve as powerful tools to extract meaningful insights and make predictions based on historical data.
Moving Averages
Moving averages are a fundamental technique in trend analysis, widely used in fields like finance, economics, and marketing. They help smooth out noisy data and identify underlying trends. Here's how moving averages work and how they can be applied:
- Smoothing Data: Moving averages involve calculating the average of a specified number of previous data points. This rolling average effectively filters out short-term fluctuations, highlighting longer-term trends.
- Types of Moving Averages: There are different types of moving averages, including Simple Moving Averages (SMA), Exponential Moving Averages (EMA), and Weighted Moving Averages (WMA). Each has its strengths and weaknesses.
- Application: Moving averages find applications in forecasting, trend identification, and anomaly detection. For example, in finance, analysts use moving averages to identify trends in stock prices and predict potential reversals.
Formula for Simple Moving Average (SMA):
SMA = (Sum of Data Points in a Period) / (Number of Data Points in the Period)
Exponential Smoothing
Exponential smoothing is another essential method for trend analysis, particularly suited for short-term forecasting and trend prediction. This technique assigns different weights to data points, with more significance given to recent observations. Here's how exponential smoothing works:
- Weighted Averaging: Exponential smoothing involves computing a weighted average of past data points with decreasing weights as you move further back in time. This reflects the belief that recent data is more relevant for predictions.
- Adaptive to Change: Exponential smoothing adapts to changes in data trends over time, making it valuable for scenarios where trends are subject to sudden shifts or fluctuations.
- Applications: This method is commonly used in demand forecasting, inventory management, and financial analysis for short-term predictions.
Formula for Exponential Smoothing (ETS):
Forecast(t+1) = α * Actual(t) + (1-α) * Forecast(t)
Regression Analysis
Regression analysis is a versatile statistical technique used to understand the relationship between one or more independent variables and a dependent variable. It's widely employed in trend analysis for various purposes:
- Linear Regression: Simple linear regression models the relationship between two variables using a straight line. It's used when you want to predict a continuous outcome variable based on one predictor variable.
- Multiple Regression: Multiple regression extends the concept to include multiple independent variables, enabling more complex trend analysis by considering numerous factors simultaneously.
- Applications: Regression analysis is used in fields like economics, marketing, and social sciences to identify trends, make predictions, and assess the impact of variables on an outcome.
Seasonal Decomposition
Seasonal decomposition is a method used to break down time series data into its constituent components: trend, seasonality, and residuals. This helps you understand and analyze the different aspects of your data:
- Trend Component: The trend component represents the underlying long-term movement in the data, allowing you to identify overall trends.
- Seasonal Component: Seasonal decomposition helps isolate and quantify repeating patterns or seasonality within your data. This is crucial for understanding periodic fluctuations.
- Residual Component: The residual component captures the unexplained variations in your data, often considered as noise or random fluctuations.
Other Analytical Techniques
Apart from the core methods mentioned above, numerous other analytical techniques can be employed depending on your specific data and analysis goals. These may include:
- ARIMA Modeling: AutoRegressive Integrated Moving Average (ARIMA) models are used for time series forecasting. They combine autoregressive and moving average components to make predictions.
- Machine Learning Algorithms: Various machine learning algorithms, such as decision trees, random forests, and neural networks, can be applied for trend analysis, especially when dealing with complex datasets.
- Nonlinear Models: In cases where linear models don't fit the data, nonlinear models like polynomial regression or logistic regression may be appropriate.
- Time Series Clustering: Cluster analysis techniques can help group similar time series data, allowing for trend analysis within clusters.
The choice of trend analysis method depends on your data characteristics, objectives, and domain-specific considerations. By mastering these techniques, you'll be well-equipped to extract valuable insights from your data and make informed decisions.
Visualization of Trends
Visualizing trends is a crucial aspect of trend analysis, as it allows you to gain a deeper understanding of your data and convey insights effectively to stakeholders. We'll explore various methods and best practices for visualizing trends.
Graphical Representations
Graphical representations are perhaps the most intuitive and widely used way to visualize trends in data. They help you spot patterns, anomalies, and correlations at a glance. Here are some common graphical representations:
Line Charts
Line charts are a fundamental tool for visualizing trends over time. They are beneficial for showcasing time series data. A line chart typically plots data points on the y-axis against time on the x-axis. The resulting line connects the data points, revealing trends and fluctuations.
Bar Graphs
Bar graphs are effective for comparing data across categories or groups. You can use vertical or horizontal bars to represent data, making it easy to see variations and trends. Bar graphs are often used in market research, demographics, and sales analysis.
Scatter Plots
Scatter plots are valuable for examining the relationships between two variables. Each data point is plotted on a two-dimensional grid, allowing you to visualize patterns, correlations, and outliers.
Area Charts
Area charts are similar to line charts but provide a visual representation of the area beneath the lines. They are instrumental in showing cumulative data, such as the total sales over a period.
Heatmaps
Heatmaps use color gradients to represent data values within a matrix. They are excellent for visualizing large datasets and identifying patterns or trends in complex data.
Histograms
Histograms are used to depict the distribution of data. They divide data into bins and display the frequency or density of data points within each bin. Histograms are commonly used in statistical analysis.
Dashboards and Tools
While individual graphs and charts are valuable, creating interactive dashboards can provide a holistic view of trends. Dashboards allow you to combine multiple visualizations into a single interface, making it easier to explore and analyze your data. Some popular dashboard tools include:
- Appinio: Appinio's intuitive platform streamlines the process of gathering real-time consumer insights, making it a valuable addition to your toolkit for trend analysis. With its global reach and user-friendly interface, Appinio empowers you to visualize trends and make data-driven decisions effortlessly.
- Tableau: Tableau is a powerful data visualization tool that enables you to create interactive and shareable dashboards. It supports a wide range of data sources and offers drag-and-drop functionality.
- Power BI: Microsoft's Power BI offers robust dashboarding capabilities with seamless integration with other Microsoft products. It's known for its user-friendly interface and extensive data connectors.
- Google Data Studio: Google Data Studio is a free, cloud-based tool for creating interactive reports and dashboards. It integrates seamlessly with other Google services like Google Sheets and Google Analytics.
Interpretation of Visualizations
Creating visualizations is just the first step; interpreting them correctly is crucial. Here are some best practices for interpreting visualizations effectively:
- Understand the Data: Before interpreting a visualization, ensure you have a solid understanding of the data, its context, and the specific question you're trying to answer.
- Identify Trends: Look for patterns, trends, and anomalies in the data. Are there noticeable peaks, troughs, or recurring patterns? Do certain data points stand out?
- Correlations and Relationships: If you're working with multiple variables, analyze how they interact. Are there strong correlations or causal relationships?
- Context Matters: Always consider the broader context of your analysis. External factors, seasonal variations, or other variables may influence the observed trends.
- Be Critical: Question your findings and assumptions. Don't jump to conclusions based solely on visualizations; cross-reference them with other data sources and conduct further analysis if necessary.
- Effective Communication: When presenting visualizations to others, ensure that your message is clear and concise. Use labels, legends, and annotations to guide your audience's understanding.
By mastering the art of visualizing trends and interpreting visualizations effectively, you can unlock valuable insights from your data, share them with stakeholders, and make informed decisions based on a deeper understanding of the trends at hand.
How to Identify Patterns and Anomalies?
In trend analysis, recognizing patterns and detecting anomalies is akin to uncovering hidden gems within your data. These insights can lead to informed decision-making and a deeper understanding of underlying trends. Here are some techniques and best practices for identifying patterns and anomalies.
Pattern Recognition
Pattern recognition involves identifying recurring structures or behaviors within your data. Patterns can take various forms, depending on your dataset and analysis goals. Here's a closer look at this crucial aspect of trend analysis:
- Types of Patterns: Patterns can manifest as trends (long-term movements), seasonality (repeating patterns), cycles (long-term fluctuations), or even more complex structures unique to your data.
- Visualization Tools: Data visualization tools and techniques, such as line charts, heatmaps, and scatter plots, are invaluable for spotting patterns. Visual representations can reveal trends that may not be apparent in raw data.
- Statistical Approaches: Statistical methods, such as time series decomposition or clustering, can help identify patterns. Decomposition separates data into trend, seasonality, and residuals while clustering groups similar data points based on patterns.
- Machine Learning: Machine learning algorithms, including clustering algorithms, neural networks, and decision trees, can be employed to automatically identify complex patterns in large datasets.
Outlier Detection
Outlier detection is the process of identifying data points that deviate significantly from the norm or expected behavior. Outliers can distort your analysis and lead to inaccurate conclusions. Here's how to effectively detect and handle outliers:
- Visual Inspection: Start by visually inspecting your data using box plots, scatter plots, or histograms. Outliers often appear as data points far removed from the bulk of the data.
- Statistical Methods: Statistical techniques like the Z-score or Tukey's method are commonly used for outlier detection. The Z-score measures how many standard deviations a data point is from the mean, helping identify extreme values.
Formula for Z-score:
Z = (X - μ) / σ
Where:- X is the data point
- μ is the mean
- σ is the standard deviation
- Machine Learning: Machine learning models, such as Isolation Forests or One-Class SVMs, can be trained to detect outliers automatically. These models are advantageous for handling large and complex datasets.
- Domain Knowledge: Sometimes, outliers can be legitimate data points with meaningful insights. It's essential to consider domain knowledge and the specific context of your analysis before deciding whether to exclude or investigate outliers.
Statistical Significance
Ensuring that the trends and patterns you identify are statistically significant is crucial for drawing reliable conclusions. Statistical significance helps you differentiate between patterns that occur by chance and those with real-world relevance.
- Hypothesis Testing: Hypothesis testing is a common approach to assess statistical significance. It involves formulating null and alternative hypotheses and conducting tests (e.g., t-tests or chi-square tests) to determine if there's enough evidence to reject the null hypothesis.
- P-Values: P-values indicate the probability of observing the data if the null hypothesis is true. A low p-value (typically below 0.05) suggests that the observed results are statistically significant.
- Effect Sizes: In addition to statistical significance, consider the effect size, which quantifies the magnitude of the observed effect. A large effect size may be practically significant even if p-values are marginal.
- Multiple Comparisons: When conducting multiple tests or comparisons, be cautious of the multiple comparisons problem, which can inflate the likelihood of finding false positives. Adjustments like Bonferroni correction can be applied to mitigate this issue.
By employing these techniques for pattern recognition, outlier detection, and assessing statistical significance, you can confidently identify meaningful trends and anomalies within your data. These insights will serve as a solid foundation for making informed decisions and taking appropriate actions based on the patterns you've uncovered.
Forecasting Using Trends
Forecasting is a vital application of trend analysis, allowing us to peer into the future and make informed decisions based on historical data patterns.
Time Series Forecasting
Time series forecasting is the art of predicting future values based on historical time series data. It's an indispensable tool in various domains, including finance, economics, and supply chain management. Here's a closer look at how time series forecasting works:
- Historical Data: Time series forecasting starts with historical data, typically collected at regular intervals (e.g., daily, monthly, annually). This data serves as the foundation for making predictions.
- Trend and Seasonality: Analysts often decompose time series data into trend, seasonal, and residual components. This decomposition helps identify underlying patterns, making it easier to create accurate forecasts.
- Forecasting Methods: Various methods can be used for time series forecasting, including:
- Moving Averages: Simple moving averages or weighted moving averages are often used for short-term forecasting.
- Exponential Smoothing: Exponential smoothing methods, such as Holt-Winters, are suitable for capturing trends and seasonality in the data.
- ARIMA Models: AutoRegressive Integrated Moving Average (ARIMA) models are powerful tools for forecasting, especially when dealing with non-stationary data.
- Prophet: Developed by Facebook, Prophet is a user-friendly tool for forecasting time series data that handles holidays, seasonality, and outliers effectively.
- Evaluation: To ensure the accuracy of your forecasts, it's essential to evaluate them using appropriate metrics like Mean Absolute Error (MAE), Mean Squared Error (MSE), or Root Mean Squared Error (RMSE).
- Continuous Monitoring: Time series forecasting is an ongoing process. Regularly update your models with new data to improve forecasting accuracy and adapt to changing trends.
Predictive Modeling
While time series forecasting focuses on one variable over time, predictive modeling expands the scope by considering multiple variables to make predictions. This approach is handy when dealing with complex datasets and scenarios. Here's how predictive modeling fits into trend analysis:
- Feature Selection: In predictive modeling, you'll typically work with multiple features (independent variables) that may influence the target variable (what you're trying to predict). Feature selection is crucial to identify the most relevant variables for your analysis.
- Machine Learning Algorithms: Predictive modeling often leverages machine learning algorithms, such as regression, decision trees, random forests, or neural networks. These algorithms can capture complex relationships between variables.
- Training and Testing: A crucial step in predictive modeling is splitting your dataset into training and testing sets. The training set is used to build and train the model, while the testing set evaluates its performance.
- Hyperparameter Tuning: Fine-tuning the model's hyperparameters is essential to achieve the best predictive performance. Techniques like cross-validation can help in this process.
- Evaluation: Similar to time series forecasting, predictive modeling requires evaluation metrics to assess model accuracy. Standard metrics include accuracy, precision, recall, F1-score, and ROC-AUC.
Forecast Evaluation
Evaluating your forecasts is a critical aspect of trend analysis. It ensures that your predictions are reliable and can be used for decision-making. Here's how you can effectively evaluate your forecasts:
- Error Metrics: Error metrics quantify the difference between predicted values and actual observations. Standard error metrics include:
- Mean Absolute Error (MAE): The average of the absolute differences between predicted and actual values.
- Mean Squared Error (MSE): The average of the squared differences between predicted and actual values.
- Root Mean Squared Error (RMSE): The square root of the MSE, providing a measure in the original units of the data.
- Visual Inspection: Visualizing your forecasts alongside the actual data can help identify patterns of overestimation or underestimation and detect any systematic errors.
- Residual Analysis: Analyzing the residuals (the differences between predicted and actual values) can reveal whether your forecasts exhibit bias or randomness.
- Forecasting Intervals: Consider constructing prediction intervals (e.g., 95% prediction intervals) to provide a range of possible outcomes, accounting for uncertainty.
- Benchmarking: Compare your forecasts to benchmark models or historical averages to determine if your model adds value.
By rigorously applying time series forecasting, predictive modeling techniques, and thorough forecast evaluation, you can harness the power of trend analysis to make accurate predictions and informed decisions that can drive success in various domains.
Examples of Trend Analysis
To truly grasp the power and practical application of trend analysis, let's delve into a few real-world examples that showcase its relevance and impact across various domains:
Financial Market Trends
Financial analysts and traders heavily rely on trend analysis to make investment decisions. By examining historical stock prices, they can identify trends such as bullish (upward) or bearish (downward) markets.
Technical indicators like moving averages and Relative Strength Index (RSI) help traders spot entry and exit points. Additionally, trend analysis can be used to predict broader economic trends, helping policymakers and investors make strategic choices.
Epidemiological Trends
In the field of public health, trend analysis plays a critical role in monitoring and managing disease outbreaks. Epidemiologists track the spread of diseases like COVID-19 by analyzing infection rates, hospitalizations, and mortality data over time. This information guides the implementation of public health measures and vaccine distribution strategies.
Retail Sales and Consumer Behavior
Retailers use trend analysis to understand consumer behavior and optimize their business strategies. By analyzing sales data, they can identify seasonal buying patterns, determine the effectiveness of marketing campaigns, and forecast future demand. This enables them to adjust inventory levels, pricing, and promotional efforts accordingly.
Climate Change and Environmental Trends
Scientists and environmentalists utilize trend analysis to study long-term climate patterns and assess the impact of climate change. They can identify trends such as rising global temperatures and sea levels by analyzing temperature, precipitation, and greenhouse gas concentration data. This information is essential for policymakers and organizations working to mitigate climate change.
Social Media Engagement
Marketing professionals and social media managers use trend analysis to monitor online conversations and engagement. By tracking metrics like likes, shares, and comments, they can identify trending topics and content that resonates with their target audience. This helps them tailor their social media strategies for maximum impact.
These examples illustrate the versatility and significance of trend analysis in diverse fields. Whether you're making financial decisions, safeguarding public health, optimizing business strategies, addressing climate change, enhancing social media engagement, or improving education, trend analysis equips you with the insights needed to make informed choices and drive positive outcomes. Identifying, interpreting, and acting upon trends is a valuable skill that empowers individuals and organizations to thrive in an ever-changing world.
Trend Analysis Challenges
Trend analysis, while a powerful tool for deriving insights from data, is not without its challenges and potential pitfalls. Being aware of these challenges is crucial for conducting effective trend analysis.
Here's a list of common trend analysis challenges and pitfalls to watch out for:
- Data Quality: Inaccurate or incomplete data can lead to erroneous conclusions. Ensure data is clean, consistent, and relevant.
- Overfitting: Overfitting occurs when a model is too complex and fits the noise in the data rather than the underlying trend. It can result in poor generalization to new data.
- Assumption Violation: Many trend analysis methods make assumptions about data distribution or stationarity. Violating these assumptions can lead to incorrect results.
- Missing Data: Dealing with missing data is a common challenge. Ignoring missing data or using inappropriate imputation methods can skew results.
- Outliers: Outliers can significantly impact trend analysis. Failing to detect and handle outliers can lead to inaccurate trend identification.
- Selection Bias: Biased sampling or selection of data can introduce bias into trend analysis, leading to non-representative results.
- Data Snooping Bias: Repeated testing and tuning on the same dataset can lead to overly optimistic results. To mitigate this bias, use separate datasets for training, validation, and testing.
- Model Complexity: Using overly complex models can lead to difficulties in interpretation and may not necessarily yield better results.
- Overemphasis on Short-Term Trends: Focusing solely on short-term trends can lead to neglecting important long-term patterns and insights.
- Lack of Domain Knowledge: Trend analysis should be complemented with domain knowledge to ensure that trends are interpreted correctly and aligned with business objectives.
Best Practices for Effective Trend Analysis
To conduct effective trend analysis and mitigate the challenges and pitfalls mentioned above:
- Clearly Define Objectives: Begin with a clear understanding of your analysis goals and objectives. Define what you want to achieve with your trend analysis.
- Data Preprocessing: Invest time in data preprocessing, including data cleaning, transformation, and handling missing values. Quality data is the foundation of reliable analysis.
- Exploratory Data Analysis (EDA): Use exploratory data analysis techniques to gain insights into your data's distribution, relationships, and potential outliers before applying trend analysis methods.
- Time Series Decomposition: When dealing with time series data, consider decomposing it into trend, seasonality, and residuals to better understand underlying patterns.
- Cross-Validation: Implement cross-validation techniques to assess the performance of your models and ensure they generalize well to new data.
- Benchmarking: Compare your analysis results against benchmark models or historical averages to gauge the added value of your trend analysis.
- Interpretability: Choose models and methods that are interpretable and align with your audience's level of understanding. Transparent models are often preferred.
- Regular Updates: Trend analysis is not a one-time task. Periodically update your analysis to capture evolving trends and changing patterns.
- Validation: Ensure the reliability of your analysis by seeking validation from domain experts or peers, especially when making critical decisions based on trends.
- Documentation: Maintain detailed documentation of your data sources, preprocessing steps, model choices, and assumptions. This documentation is invaluable for reproducibility.
- Continuous Learning: Stay informed about emerging trends in data analysis, machine learning, and statistical techniques to continually improve your trend analysis skills.
By adhering to these best practices and remaining vigilant about potential challenges and pitfalls, you can enhance the effectiveness and reliability of your trend analysis, ultimately leading to more informed decision-making and actionable insights.
Conclusion for Trend Analysis
Trend analysis is your compass in the vast sea of data. It helps you navigate uncertainty by identifying patterns, predicting future developments, and making well-informed decisions. By following the methods, best practices, and avoiding common pitfalls outlined in this guide, you can harness the power of trends and turn data into actionable insights. Remember, whether you're steering a business, solving real-world problems, or just satisfying your curiosity, trend analysis is a valuable tool that can guide you toward success.
Now armed with the knowledge and skills needed to decipher data trends, you can embark on a journey of discovery, continuously learning, adapting, and making data-driven choices. As you traverse this landscape, keep in mind that trends are the threads connecting the past, present, and future, allowing you to confidently shape your path and navigate toward your desired destination.
How to Conduct Trend Analysis in Minutes?
Discover the power of real-time market research with Appinio! As a cutting-edge platform, Appinio empowers businesses to harness real-time consumer insights for rapid, data-driven decision-making. Say goodbye to lengthy research processes and hello to actionable insights in minutes.
Here's why Appinio is your ultimate partner in trend analysis:
- Instant Insights: With our platform, you can go from questions to insights in minutes, ensuring you have the data you need precisely when you need it.
- User-Friendly Interface: No research degree required! Our intuitive platform makes market research accessible to everyone, allowing you to conduct sophisticated analyses without the complexity.
- Global Reach: Reach your target audience effortlessly with access to over 90 countries and the ability to define precise target groups from over 1200 characteristics. Conduct research on a global scale with ease.
Get facts and figures 🧠
Want to see more data insights? Our free reports are just the right thing for you!