Discrete vs. Continuous Data: Differences and Examples
Appinio Research · 14.03.2024 · 24min read
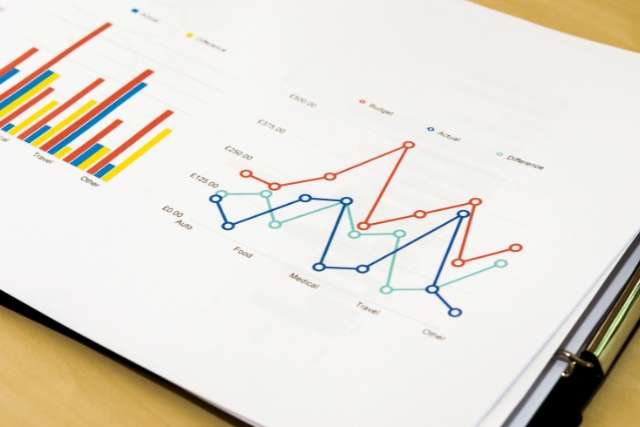
Content
Have you ever wondered why some data seems to come in distinct, separate values, while other data varies smoothly over a range? Understanding this difference is crucial for anyone dealing with data, from students to professionals in fields like finance, healthcare, and engineering. In the world of data analysis, we encounter two main types of data: discrete and continuous. But what exactly do these terms mean, and why do they matter?
In this guide, we'll explore the fundamental concepts of discrete and continuous data, uncover their defining characteristics, delve into practical examples, and provide valuable insights into choosing suitable analysis techniques. Whether you're analyzing survey responses, tracking temperature fluctuations, or optimizing production processes, grasping the distinction between discrete and continuous data is the key to unlocking meaningful insights and making informed decisions.
Understanding Discrete and Continuous Data
Before delving into the practical aspects of analyzing data, it's essential to understand the fundamental concepts of discrete and continuous data.
What is Discrete Data?
Discrete data consists of distinct, separate values that are countable and finite. These values typically arise from counting or categorizing observations and can only take on specific, enumerable values with no intermediate values between them.
Examples of discrete data include the number of students in a classroom, the outcomes of flipping a coin, or the number of defects in a production line.
What is Continuous Data?
Continuous data, on the other hand, can take on an infinite number of values within a specified range. Unlike discrete data, continuous data exhibits a smooth, unbroken continuum without distinct breaks or separations between values. Continuous data often arises from measuring phenomena that can vary continuously, such as time, temperature, or weight.
Examples of continuous data include measuring the height of individuals in a population, recording temperature variations throughout a day, or tracking the speed of a moving vehicle.
Understanding the distinction between discrete and continuous data is crucial for accurately representing and analyzing different types of observations and phenomena.
Importance of Understanding the Distinction
Understanding the difference between discrete and continuous data is essential for several reasons:
- Appropriate Analysis Techniques: Different types of data require different analysis techniques. By recognizing whether your data is discrete or continuous, you can select appropriate statistical measures, visualization methods, and analytical approaches to derive meaningful insights.
- Effective Communication: Communicating findings effectively relies on accurately representing the nature of the data. Understanding whether your data is discrete or continuous allows you to choose visualizations and presentations that best convey the underlying patterns and trends to your audience.
- Informed Decision-Making: Whether you're making business decisions, conducting scientific research, or implementing policy changes, understanding the nature of your data is critical for making informed decisions. By recognizing the distinction between discrete and continuous data, you can make more accurate predictions, identify opportunities, and mitigate risks effectively.
- Interdisciplinary Applications: The distinction between discrete and continuous data extends across various fields, including mathematics, statistics, engineering, finance, healthcare, and beyond. Whether you're analyzing customer survey responses, modeling environmental phenomena, or optimizing manufacturing processes, understanding the nature of your data is fundamental for success across diverse domains.
By understanding the distinction between discrete and continuous data, you can enhance the quality and reliability of your data analysis, enabling more accurate insights, informed decision-making, and effective problem-solving in a wide range of applications.
As you navigate the intricate world of data analysis, Appinio stands as your trusted ally, ready to streamline your research endeavors. Our platform seamlessly integrates with your analytical processes, empowering you to effortlessly collect real-time consumer insights and drive data-driven decisions. With Appinio, you not only gain valuable insights but also uncover opportunities for growth and innovation.
Experience the power of intuitive market research with Appinio – book a demo today and embark on a journey towards data-driven success!
Discrete Data
Understanding discrete data is fundamental to many aspects of data analysis. Let's explore what discrete data entails, along with its characteristics, examples, representations, and statistical measures.
Characteristics of Discrete Data
Discrete data is characterized by distinct, separate values that are countable and finite. Unlike continuous data, which can take on any value within a range, discrete data comprises individual observations with no intermediate values between them. This type of data often arises from counting or categorizing phenomena where values cannot be divided into smaller units.
For instance, consider the number of students in a classroom or the outcomes of flipping a coin. These examples illustrate how discrete data consists of specific, enumerable values that can be counted.
Discrete Data Examples
Examples of discrete data abound in various fields, including mathematics, statistics, and everyday life:
- Counting Data: This includes any data that involves counting discrete items or occurrences, such as the number of books on a shelf, the number of cars in a parking lot, or the number of goals scored in a soccer match.
- Categorical Data: Categorical data represents characteristics or qualities that can be divided into distinct categories. Examples include gender (male/female), eye color (blue, brown, green), or types of fruits (apple, orange, banana).
Discrete Data Representation
Discrete data is often represented visually using histograms or bar charts. These graphical representations provide a clear visualization of the frequency or distribution of discrete values. In a histogram, each bar represents a specific value or category, with the height of the bar indicating the frequency of occurrence.
Bar charts, on the other hand, use bars of equal width to represent categories, with the height of each bar corresponding to the frequency or count of observations in that category.
Discrete Data Statistical Measures
When analyzing discrete data, various statistical measures can provide insights into its central tendency and variability:
- Mode: The mode represents the most frequently occurring value in a dataset. It provides a measure of central tendency and is particularly useful for identifying the most common observation.
- Median: The median is the middle value in a dataset when the values are arranged in ascending or descending order. It divides the dataset into two equal parts, with half of the values falling below and half above the median.
- Mean: While less commonly used for discrete data compared to continuous data, the mean (average) can still be calculated by summing all values and dividing by the total count of observations. However, it may not always accurately represent the central tendency if the data is heavily skewed or contains outliers.
Understanding these statistical measures allows you to gain insights into the distribution and characteristics of discrete data, enabling informed decision-making and analysis.
Continuous Data
Continuous data plays a crucial role in various fields, ranging from scientific research to business analytics. Let's delve deeper into what continuous data entails.
Characteristics of Continuous Data
Continuous data differs from discrete data in that it can take on an infinite number of values within a given range. Unlike discrete data, which consists of distinct, separate values, continuous data is uncountable and can assume any value within a specified interval. This data type often arises from measuring phenomena that can vary continuously, such as time, distance, temperature, or weight.
For example, consider measuring the height of individuals in a population or recording the temperature variations throughout a day. These examples demonstrate how continuous data encompasses an extensive range of possible values without discrete boundaries.
Continuous Data Examples
Continuous data manifests in various forms across different domains:
- Measurements: This category includes any data obtained through measurement processes, such as height, weight, length, or volume. For instance, measuring the length of a piece of string or the volume of liquid in a container yields continuous data since these measurements can take on any value within a range.
- Time: Time is a quintessential example of continuous data, as it can be divided into infinitely small intervals. Whether tracking seconds, minutes, hours, or fractions of a second, time exhibits continuous characteristics with no discrete breaks between intervals.
- Temperature: Temperature readings provide another illustration of continuous data. From sub-zero temperatures to scorching heat, temperature measurements span a continuous spectrum, allowing for an infinite range of values between any two points.
Continuous Data Representation
Visualizing continuous data often involves techniques that highlight the distribution and density of observations:
- Frequency Polygons: Frequency polygons are graphical representations that depict the distribution of continuous data. They consist of line segments connecting points representing the frequencies of different values or intervals. Frequency polygons provide a smooth, continuous representation of the data distribution, allowing for easy interpretation of patterns and trends.
- Density Plots: Density plots, also known as kernel density plots, offer another effective way to visualize the distribution of continuous data. These plots display the probability density function of the data, showing the relative likelihood of different values occurring within the dataset. By providing a smoothed representation of the data distribution, density plots help identify peaks, valleys, and overall patterns in the data.
Continuous Data Statistical Measures
Analyzing continuous data involves employing statistical measures to summarize its central tendency, dispersion, and shape:
- Mean: The mean, or average, is a common measure of central tendency for continuous data. It represents the arithmetic average of all values in the dataset and provides insight into the typical value around which observations cluster.
- Median: Similar to discrete data, the median serves as a measure of central tendency for continuous data. It represents the middle value when the data is sorted in ascending or descending order and is less sensitive to extreme values compared to the mean.
- Mode: While less frequently used for continuous data, the mode can still provide valuable insights by identifying the most frequently occurring value or values in the dataset.
- Measures of Variability: Measures such as standard deviation and variance quantify the spread or dispersion of continuous data around the central tendency. These measures offer insights into the variability and consistency of observations within the dataset.
Understanding these statistical measures enables you to characterize and analyze the distribution of continuous data effectively, facilitating informed decision-making and interpretation of results.
Discrete vs. Continuous Data
Understanding the distinctions between discrete and continuous data is essential for accurate data analysis and interpretation. Let's explore the key differences of these two types of data.
Nature of the Data
The primary distinction between discrete and continuous data lies in their fundamental nature:
- Discrete Data: Discrete data consists of distinct, separate values with no intermediate values between them. These values are countable and finite, representing specific observations or categories. Discrete data typically arises from counting or categorizing phenomena where values cannot be divided into smaller units.
- Continuous Data: In contrast, continuous data can take on an infinite number of values within a given range. It exhibits a smooth, unbroken continuum without distinct breaks or separations between values. Continuous data often arises from measuring phenomena that can vary continuously, such as time, temperature, or weight.
Graphical Representation
The graphical representation of discrete and continuous data differs based on their unique characteristics:
- Discrete Data: Discrete data is often represented using histograms or bar charts, where each bar or column represents a specific value or category. These visualizations provide a clear depiction of the frequency or distribution of discrete values, with distinct bars corresponding to individual observations.
- Continuous Data: Continuous data is typically represented using frequency polygons or density plots, which highlight the distribution and density of observations across a continuous range. Frequency polygons connect points representing the frequencies of different values, while density plots display the probability density function of the data, offering a smoothed representation of the data distribution.
Statistical Analysis
Analyzing discrete and continuous data involves employing different statistical techniques and measures:
- Discrete Data: Statistical measures commonly used for discrete data include the mode, median, and mean. These measures provide insights into the central tendency and variability of discrete values, helping to summarize and interpret the data effectively.
- Continuous Data: Statistical analysis of continuous data often involves measures such as the mean, median, mode, standard deviation, and variance. These measures capture the central tendency, dispersion, and shape of the data distribution, enabling deeper insights into the variability and patterns within the dataset.
Real-World Applications
Both discrete and continuous data find applications across various fields and disciplines:
- Discrete Data: Discrete data is prevalent in fields such as finance, economics, and engineering, where counting or categorizing phenomena is common. Applications include inventory management, survey analysis, and quality control, where discrete values represent tangible observations or events.
- Continuous Data: Continuous data is widely used in scientific research, healthcare, and environmental monitoring, where precise measurements and continuous variables play a crucial role. Applications range from climate modeling and medical diagnostics to financial forecasting, where continuous data provides detailed insights into complex phenomena.
Understanding the differences between discrete and continuous data allows researchers, analysts, and decision-makers to choose appropriate analytical techniques, interpret results accurately, and derive meaningful insights from their data.
Whether analyzing discrete counts or continuous measurements, recognizing these distinctions is vital for effective data-driven decision-making in various domains.
Discrete and Continuous Data Analysis
When conducting data analysis, several crucial considerations can significantly impact the accuracy and reliability of your findings. Let's explore key factors to keep in mind when analyzing both discrete and continuous data.
Choosing Appropriate Visualizations
Selecting the correct visualization is paramount to effectively communicate insights from your data. Consider the following factors when choosing visualizations:
- Nature of the Data: Determine whether your data is discrete or continuous, as this will influence the choice of visualization. For discrete data, histograms or bar charts are often suitable, providing a clear representation of frequencies or categories. Continuous data, on the other hand, may be best visualized using frequency polygons or density plots to highlight distribution patterns.
- Audience and Purpose: Tailor your visualizations to the intended audience and the specific objectives of your analysis. Choose visualizations that are intuitive and easy to interpret for stakeholders who may not have a technical background. Consider whether your audience will benefit more from static charts or interactive visualizations that allow for exploration and interaction with the data.
- Complexity of the Data: Assess the complexity of your data and choose visualizations that effectively capture its intricacies. Consider using techniques such as scatter plots, heatmaps, or parallel coordinates for datasets with multiple variables or dimensions to visualize relationships and patterns effectively.
Selecting Relevant Statistical Measures
Choosing the appropriate statistical measures is essential for gaining insights into your data and making informed decisions. Consider the following factors when selecting statistical measures:
- Type of Data: Determine whether your data is discrete or continuous, as this will dictate which statistical measures are most relevant. For discrete data, focus on measures such as mode, median, and mean, which provide insights into central tendency and variability. For continuous data, consider additional measures such as standard deviation, variance, and correlation coefficients to capture the distribution and relationships within the dataset.
- Research Questions: Align your choice of statistical measures with the research questions or objectives of your analysis. Identify the key insights you hope to uncover and select measures that directly address these objectives. For example, if you're interested in understanding the variability of a dataset, measures of dispersion such as range or interquartile range may be more relevant.
- Data Distribution: Consider the distribution of your data when selecting statistical measures. If your data follows a normal distribution, parametric measures such as the mean and standard deviation may be appropriate. However, if your data is skewed or exhibits non-normal distribution, non-parametric measures or transformations may be necessary to accurately summarize and analyze the data.
Addressing Data Limitations and Assumptions
Acknowledging and addressing the limitations and assumptions of your data is crucial for ensuring the validity and reliability of your analysis.
- Data Quality: Assess the quality of your data by examining factors such as completeness, accuracy, and consistency. Address any missing or erroneous data through data cleaning and validation procedures to minimize the impact of data quality issues on your analysis results.
- Assumptions of Statistical Tests: Be aware of the underlying assumptions of the statistical tests or techniques you're using and assess whether these assumptions hold true for your data. For example, many statistical tests assume that data is independent and identically distributed, so verifying these assumptions before interpreting the results is essential.
- Sampling Bias: Consider the potential for sampling bias or selection bias in your data collection process. Ensure that your sample is representative of the population of interest and take steps to mitigate bias through random sampling techniques or stratification.
By carefully considering these factors during the data analysis process, you can enhance the quality and reliability of your findings, enabling more accurate decision-making and actionable insights.
Discrete and Continuous Data Analysis Examples
Let's delve into practical examples and applications to illustrate how to analyze discrete and continuous datasets effectively, as well as how to handle mixed datasets containing both types of variables.
Analyzing Discrete Datasets
Analyzing discrete datasets often involves understanding the frequency distribution of values and summarizing central tendencies. Here's a step-by-step example:
Example: Analyzing Survey Responses
Suppose you conducted a survey to gather feedback on customer satisfaction, and respondents were asked to rate their satisfaction on a scale of 1 to 5, with 1 being "Very Dissatisfied" and 5 being "Very Satisfied."
- Data Collection: Collect responses from survey participants and organize them into a dataset.
- Frequency Distribution: Calculate the frequency of each response value (1 to 5) to understand the distribution of satisfaction levels among respondents.
- Visual Representation: Create a bar chart or histogram to visually represent the frequency distribution of satisfaction ratings. This visualization provides a clear overview of the distribution and allows you to identify any patterns or trends.
- Statistical Measures: Calculate the mode, median, and mean of the satisfaction ratings to summarize the central tendency of the dataset. These measures provide insights into the most common response value and the overall average satisfaction level.
Analyzing Continuous Datasets
Analyzing continuous datasets involves examining the distribution, central tendency, and variability of data points. Here's an example of analyzing temperature data:
Example: Analyzing Daily Temperature Readings
Suppose you have collected daily temperature readings over a month to analyze weather patterns.
- Data Collection: Collect temperature data for each day of the month and organize it into a dataset.
- Data Visualization: Create a line graph or scatter plot to visualize the daily temperature readings over the month. This visualization allows you to observe any trends or patterns in temperature fluctuations.
- Statistical Analysis: Calculate the mean, median, and standard deviation of the temperature readings to understand the central tendency and variability of the data. Additionally, you can identify outliers or unusual temperature spikes that may warrant further investigation.
Mixed Datasets: Handling Both Discrete and Continuous Variables
Handling mixed datasets containing both discrete and continuous variables requires careful consideration of data types and appropriate analysis techniques. Here's an example of analyzing a mixed dataset:
Example: Analyzing Sales Data
Suppose you have a dataset containing both discrete variables (e.g., number of products sold) and continuous variables (e.g., sales revenue) for a retail store.
- Data Organization: Organize the dataset into separate columns for discrete and continuous variables to facilitate analysis.
- Discrete Variable Analysis: Analyze the frequency distribution of the number of products sold using histograms or bar charts. Calculate the mode, median, and mean to summarize the central tendency of sales volume.
- Continuous Variable Analysis: Analyze the distribution of sales revenue using density plots or frequency polygons. Calculate the mean, median, and standard deviation to understand the central tendency and variability of revenue.
- Correlation Analysis: Explore potential relationships between discrete and continuous variables by calculating correlation coefficients. For example, you may examine the correlation between sales volume and revenue to understand how changes in one variable affect the other.
By following these steps and utilizing appropriate analysis techniques, you can gain valuable insights from mixed datasets containing both discrete and continuous variables, enabling informed decision-making and actionable insights.
Conclusion for Discrete and Continuous Data
Understanding the difference between discrete and continuous data is like having a powerful tool in your data analysis toolkit. By recognizing whether your data comes in distinct counts or smooth ranges, you can choose the proper statistical measures, visualization techniques, and analysis methods to uncover valuable insights. Whether you're a student learning about data analysis or a seasoned professional making critical decisions, mastering the distinction between discrete and continuous data empowers you to extract meaningful information from your datasets with confidence.
Moreover, as you continue your journey in data analysis, remember that the distinction between discrete and continuous data extends far beyond mere numbers. It's about understanding the underlying nature of the phenomena you're studying, whether it's the behavior of customers, the fluctuations of temperatures, or the intricacies of financial markets. By embracing this understanding, you not only enhance your analytical skills but also gain a deeper appreciation for the rich complexity of the world around us. So, armed with this knowledge, go forth and analyze your data with clarity and precision, knowing that you have the insight to make a real difference in your field.
How to Easily Collect Discrete and Continuous Data?
Introducing Appinio, your go-to platform for real-time market research! Say goodbye to tedious data collection and hello to lightning-fast insights for your business decisions. With Appinio, conducting your own market research becomes a breeze, whether you're exploring discrete or continuous data.
Here's why Appinio stands out:
- Lightning-fast Insights: Get from questions to actionable insights in minutes, allowing you to make informed decisions on the fly.
- User-Friendly Interface: No need for a research PhD – our intuitive platform empowers anyone to dive into market research effortlessly.
- Global Reach, Local Insights: Reach your target audience with precision from over 90 countries, and tailor your surveys based on 1200+ characteristics.
- Expert Guidance: Our dedicated research consultants will be by your side every step of the way, ensuring you extract maximum value from your data.
Get facts and figures 🧠
Want to see more data insights? Our free reports are just the right thing for you!