What is Ordinal Data? Definition, Analysis, Examples
Appinio Research · 05.02.2024 · 34min read
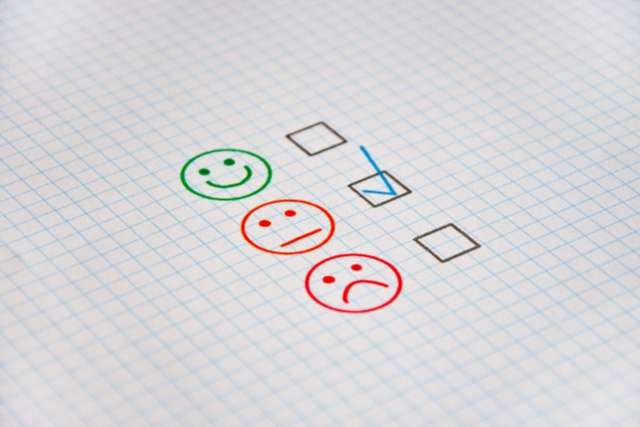
Content
Have you ever wondered how researchers make sense of data with categories that have a clear order but unequal intervals? In the world of statistics and research, this type of data, known as ordinal data, plays a pivotal role in unraveling complex relationships, making informed decisions, and understanding the preferences and perceptions of individuals.
In this guide, we will delve deep into ordinal data, exploring its definition, characteristics, significance, and practical applications. From data collection to advanced analysis techniques, we'll equip you with the knowledge and tools needed to harness the power of ordinal data effectively.
What is Ordinal Data?
Ordinal data represents a categorical or qualitative type of data in which categories possess a meaningful order or ranking but do not have equal intervals between them. Unlike nominal data, where categories are merely labels with no inherent order, ordinal data categorizes information with a clear sequence or hierarchy.
Ordinal Data Characteristics
- Order: The primary feature of ordinal data is that categories are ordered or ranked in a meaningful way. This implies that some categories are "higher" or "lower" than others based on a specific criterion or attribute.
- Unequal Intervals: While there is an order to the categories, the intervals between them are not necessarily equal. In other words, the difference between categories may not be uniform or quantifiable.
- Discrete Categories: Ordinal data consists of discrete categories or levels. Each category represents a distinct and non-overlapping group.
- No Fixed Origin: Ordinal data lacks a fixed origin or zero point. Unlike interval or ratio data, which have meaningful zero points, ordinal data does not possess such a reference point.
- Limited Arithmetic Operations: Mathematical operations like addition, subtraction, multiplication, or division are generally not meaningful for ordinal data. You cannot calculate the average of ordinal categories in a meaningful way.
Understanding these characteristics is essential for appropriate data analysis and interpretation when working with ordinal data.
Importance of Ordinal Data
Ordinal data plays a pivotal role in various fields and research endeavors due to its unique attributes and utility. Here are several reasons why ordinal data holds significant importance:
- Hierarchical Insights: Ordinal data allows researchers to capture hierarchical information, providing insights into the relative order or ranking of categories. This is valuable for understanding preferences, attitudes, or rankings.
- Quantifying Qualitative Information: It bridges the gap between qualitative and quantitative data by providing a structured way to represent subjective or qualitative information. This makes it amenable to statistical analysis.
- Applicability in Surveys: Ordinal data is commonly used in surveys and questionnaires to gauge opinions, perceptions, or levels of agreement. Likert scales, for instance, provide a structured way to collect and analyze ordinal responses.
- Flexibility in Analysis: Researchers can apply various statistical methods tailored to ordinal data, such as ordinal regression, Mann-Whitney U tests, or Kruskal-Wallis tests. This versatility allows for in-depth analysis and hypothesis testing.
- Interdisciplinary Relevance: Ordinal data finds applications in diverse fields, including psychology, social sciences, medicine, marketing, and education. Its ability to capture nuanced relationships and rankings makes it indispensable in these domains.
- Decision-Making Support: In fields like market research and customer satisfaction analysis, ordinal data assists organizations in making informed decisions based on customer preferences and rankings.
Recognizing the importance of ordinal data and its unique characteristics empowers researchers to extract valuable insights and draw meaningful conclusions from this type of data, contributing to the advancement of knowledge across various disciplines.
Ordinal Data Collection and Measurement
In ordinal data, meticulous data collection and measurement are paramount for accurate analysis and meaningful insights. Let's explore this critical aspect, covering various nuances and techniques to enhance your data collection process.
Types of Ordinal Scales
Ordinal scales come in different forms, each suited to specific research questions and scenarios. Here's a closer look at some common types:
- Likert Scale: The Likert scale is perhaps the most familiar ordinal scale. It comprises a series of statements or questions, allowing respondents to express their level of agreement or disagreement. Ranging from "strongly agree" to "strongly disagree," these responses are typically quantified using numerical values, such as 1 to 5 or 0 to 4. Likert scales are widely used in surveys, but variations like the semantic differential scale or the Guttman scale offer additional options.
- Ordinal Rankings: This type of ordinal scale involves ranking items or options based on a specific criterion. For example, you might ask respondents to rank product features from most important to least important or prioritize a list of tasks. Ordinal rankings are valuable when you want to understand preferences or priorities without assigning precise values to the categories.
- Ordinal Categories with Descriptions: In some cases, ordinal data may include categories with descriptions rather than just numerical values. For instance, in a customer feedback survey, respondents might rate their experience on a scale of "excellent," "good," "average," "poor," and "very poor." These descriptive categories can provide richer insights but may require careful handling during analysis.
Remember that the choice of ordinal scale should align with your research objectives and the nature of the data you aim to collect.
Data Collection Methods
Selecting the proper data collection method is pivotal in ensuring the quality and reliability of your ordinal data.
- Surveys and Questionnaires: Surveys and questionnaires are versatile tools for collecting ordinal data. Crafting well-designed questions is crucial. Ensure that your questions are clear, concise, and free from bias. Additionally, pilot testing can help identify and address any ambiguities or issues before launching the survey.
- Observations: When dealing with observable behaviors or phenomena, observational data collection can be invaluable. Researchers systematically record and categorize observations into ordinal scales. For example, in educational research, observers might use ordinal scales to assess classroom behavior or student engagement.
- Interviews: Interviews are an excellent choice when you need to delve deeper into respondents' thoughts and experiences. They allow for open-ended discussions and can be especially beneficial in qualitative research. However, interviews can be resource-intensive and require skilled interviewers to maintain consistency.
- Existing Data: Sometimes, you might have access to existing datasets that contain ordinal data. These datasets can be valuable for secondary analysis. However, it's crucial to understand how the data was collected, coded, and labeled to ensure its suitability for your research.
As you navigate the intricacies of ordinal data collection, consider leveraging cutting-edge solutions like Appinio to streamline your research endeavors. Appinio empowers you with the tools to design user-friendly surveys and questionnaires, ensuring clarity and precision in your data collection process. With its intuitive platform, you can effortlessly gather real-time insights from a global audience, accelerating your research efforts.
Ready to see it in action? Book a demo now to discover the power of Appinio and enhance your data collection capabilities!
Data Coding and Labeling
Once you've gathered your ordinal data, the next step is to code and label it effectively. This process enhances the usability and interpretability of your dataset.
- Numerical Values: Assign numerical values to ordinal categories that reflect their order, but remember that these values do not imply equal intervals. For instance, on a Likert scale ranging from 1 to 5, "strongly agree" might be coded as 5, and "strongly disagree" as 1. Ensure that the chosen numerical scale is logical and meaningful.
- Clear and Consistent Labels: Consistency in labeling is paramount. Use clear and unambiguous labels for each ordinal category. Labels should be intuitive, allowing anyone working with the data to understand the meaning of each category. Ambiguous or confusing labels can lead to misinterpretation.
- Data Validation: Implement data validation procedures to identify and rectify coding or labeling errors. Data validation checks help maintain data accuracy and reliability. They can include range checks, logic checks, and consistency checks to catch inconsistencies or anomalies.
- Documentation: Keep thorough documentation of your coding and labeling process. This documentation should include the rationale behind numerical assignments and any decisions related to category labels. Clear documentation ensures transparency and facilitates collaboration with other researchers.
Properly managed data coding and labeling set the stage for robust analysis and meaningful findings. By paying careful attention to these steps, you ensure that your ordinal data is both accurate and accessible for subsequent research or analysis endeavors.
Descriptive Statistics for Ordinal Data
Now that you've gained a solid understanding of collecting and measuring ordinal data, it's time to explore the tools and techniques used to describe and summarize this type of data. Descriptive statistics provide valuable insights into the distribution, central tendencies, and spread of your ordinal data.
Frequency Distribution
A frequency distribution is a fundamental tool for analyzing ordinal data. It provides a clear overview of how frequently each category or response occurs within your dataset. Let's dive deeper into this crucial aspect:
- Creating a Frequency Table: Start by building a frequency table that lists each ordinal category along with the corresponding counts. This table offers a quick snapshot of your data's distribution.
- Visualizing Frequencies: Transforming your frequency table into a visual representation, such as a bar chart or histogram, can make the distribution patterns even more accessible. These visuals allow you to see the relative frequencies of each category and identify any trends or patterns.
- Interpreting Skewness: Pay attention to the shape of the frequency distribution. Ordinal data distributions can exhibit various patterns, including symmetry, skewness, or multimodality. Understanding these patterns is essential for drawing meaningful conclusions from your data.
- Percentages and Proportions: In addition to counts, consider expressing frequencies as percentages or proportions. This helps you compare the relative importance of different categories within your dataset, particularly in scenarios where sample sizes vary.
Measures of Central Tendency
Measures of central tendency help you identify the "center" or typical value within your ordinal data. While ordinal data lacks precise intervals, these measures provide valuable insights into the data's central tendencies:
- Mode: The mode represents the most frequently occurring category within your ordinal dataset. It is a valuable measure for identifying the most common response or category. For example, in a Likert scale survey, the mode might reveal the most prevalent level of agreement.
- Median: The median is the middle value when your ordinal data is arranged in order. It is less influenced by extreme values or outliers compared to other central tendency measures. For skewed distributions, the median can provide a more robust estimate of the center.
- Mean (Cautionary Note): While calculating the mean (average) is possible for ordinal data, it's essential to use caution. The mean assumes equal intervals between categories, which may not be valid for ordinal data. If using the mean, be sure to acknowledge its limitations and consider it alongside other measures.
Measures of Dispersion
Measures of dispersion reveal how spread out or variable your ordinal data is. Understanding the spread of data is crucial for assessing the degree of agreement or disagreement among respondents or the variability in rankings:
- Range: The range is the simplest measure of dispersion and indicates the difference between the highest and lowest values in your ordinal dataset. While easy to calculate, the range can be sensitive to outliers.
- Interquartile Range (IQR): The IQR focuses on the middle 50% of your data. It is calculated as the difference between the third quartile (Q3) and the first quartile (Q1). The IQR is robust against outliers and provides insights into the central spread of data.
- Variance and Standard Deviation (Cautionary Note): Variance and standard deviation are typically used with interval or ratio data. While they can be calculated for ordinal data, their interpretation should be cautious due to the assumption of equal intervals between categories.
- Box Plots: Box plots visually represent the spread of ordinal data by showing quartiles, outliers, and the median. They are especially useful for comparing distributions across different groups or categories.
By exploring these descriptive statistics, you can gain a better understanding of your ordinal data's characteristics, distribution, and central tendencies. These insights pave the way for further analysis and interpretation.
Data Visualization for Ordinal Data
Visualizing ordinal data is a powerful way to extract meaningful insights and communicate your findings effectively. Let's explore various visualization techniques tailored specifically for ordinal data analysis.
Bar Charts
Bar charts are among the most common and intuitive tools for visualizing ordinal data. They are instrumental when working with ordinal data that involves Likert scales or other categorical responses. Here's a closer look at how to effectively use bar charts:
- Category Frequency Display: In a bar chart, each ordinal category is represented as a separate bar. The height or length of the bar corresponds to the frequency or count of responses in that category. This allows you to quickly identify the most and least common responses.
- Comparative Analysis: Bar charts are excellent for comparing different categories or groups. You can easily see which categories have higher or lower frequencies, making it valuable for understanding patterns in your data.
- Grouped Bar Charts: When comparing multiple groups or subcategories, grouped bar charts can be employed. They enable you to display and compare multiple sets of ordinal data side by side, providing insights into variations across groups.
- Stacked Bar Charts: Stacked bar charts are suitable for illustrating the composition of ordinal data within a single category. Each bar is divided into segments, with each segment representing a subcategory or response option. This type of chart is useful when exploring the distribution of ordinal responses within specific contexts.
Pareto Charts
A Pareto chart is a specialized form of bar chart that prioritizes ordinal categories based on their frequency or impact. It's handy when you want to focus on the most significant factors within your data:
- Frequency-Ordered Categories: In a Pareto chart, ordinal categories are arranged in descending order of frequency, with the most frequent category on the left. This visual representation helps you identify and prioritize the most prevalent responses or issues.
- Focus on the Vital Few: The Pareto principle, often referred to as the 80/20 rule, suggests that a significant portion of effects comes from a small portion of causes. Pareto charts help you pinpoint the "vital few" categories that contribute the most to your dataset's characteristics.
- Dual-Axis Charts: In some cases, Pareto charts may incorporate a dual-axis approach. This means that you can overlay a line graph on the bar chart to represent cumulative percentages. This provides additional insights into the cumulative impact of ordinal categories as you move from left to right on the chart.
- Actionable Insights: Pareto charts are not only descriptive but also actionable. They guide decision-makers by highlighting areas that require attention or intervention. By focusing efforts on the most significant categories, you can optimize resources and strategies effectively.
Ordered Probit Plots
Ordered probit plots are specialized graphical tools used primarily in statistical modeling when you want to understand the relationship between ordinal data and other variables, such as time or demographics:
- Probit Transformation: Ordered probit plots are based on the probit transformation, which converts ordinal data into a continuous scale. This transformation is helpful when you want to incorporate ordinal variables into regression models, such as ordinal logistic regression.
- Ordered Categories: In these plots, the ordinal categories are represented on the vertical axis, and the probit-transformed values are plotted on the horizontal axis. This allows you to visualize the relationship between ordinal responses and other continuous variables.
- Slope and Thresholds: By examining the slope and thresholds of ordered probit plots, you can gain insights into how changes in predictor variables impact the likelihood of moving from one ordinal category to another. This is valuable for understanding the drivers of ordinal responses.
- Model Validation: Ordered probit plots are also used in model validation. They help assess the goodness of fit of ordinal regression models by comparing observed and predicted probabilities of category transitions.
By incorporating these advanced visualization techniques into your analysis, you can not only explore the distribution of ordinal data but also uncover relationships and prioritize factors for further investigation. Visualization is a powerful tool for making your ordinal data analysis more insightful and accessible.
Inferential Statistics for Ordinal Data
Once you've explored descriptive statistics and visualized your ordinal data, the next step is to delve into inferential statistics. We'll cover key statistical tests tailored for ordinal data analysis to help you draw meaningful conclusions and make informed decisions based on your findings.
Chi-Square Test
The Chi-Square Test is a powerful statistical test for assessing the association or independence between two categorical variables, often involving ordinal data. It's beneficial when you want to determine if there's a significant relationship between the variables. Here's how it works:
- Contingency Tables: To perform a Chi-Square Test with ordinal data, you typically create a contingency table that cross-tabulates the two categorical variables. This table displays the frequency of each combination of responses.
- Expected and Observed Frequencies: The test calculates expected frequencies under the assumption of independence between the variables. It then compares these expected frequencies to the observed frequencies in your dataset. Deviations from expected values indicate a significant association.
- Degrees of Freedom: The Chi-Square Test is associated with degrees of freedom, which depend on the dimensions of the contingency table. Degrees of freedom affect the critical value used for hypothesis testing.
- Hypothesis Testing: The Chi-Square Test involves setting up null and alternative hypotheses. The test statistic is compared to a critical value (usually from a Chi-Square distribution table) to determine whether to reject the null hypothesis, indicating a significant relationship.
- Interpretation: A significant Chi-Square result suggests that the two categorical variables are not independent. The strength and direction of the relationship can be further explored through measures like Cramér's V or phi coefficient.
Mann-Whitney U Test
The Mann-Whitney U Test is a non-parametric statistical test used to compare two independent groups when the dependent variable is ordinal. It's used when you want to assess whether there are significant differences in ordinal data between two groups. Here's how it operates:
- Ranking Data: In the Mann-Whitney U Test, data from both groups are combined and ranked. Each observation receives a rank based on its position in the combined dataset, from lowest to highest.
- Test Statistic: The test statistic U is calculated, representing the sum of ranks for one group relative to the other. It measures the likelihood that a randomly selected observation from one group will have a higher value than a randomly selected observation from the other group.
- Hypothesis Testing: Similar to other statistical tests, the Mann-Whitney U Test involves setting up null and alternative hypotheses. The test statistic U is compared to critical values to determine statistical significance.
- Effect Size: Effect size measures like the common language effect size (CL) or the rank-biserial correlation (r) can be calculated to assess the practical significance of the differences between the groups.
- Assumptions: Unlike parametric tests, the Mann-Whitney U Test does not assume normality of data or equal variances. It is robust against outliers and skewed distributions.
Kruskal-Wallis Test
The Kruskal-Wallis Test is an extension of the Mann-Whitney U Test and is used to compare ordinal data among three or more independent groups. It's a non-parametric alternative to one-way analysis of variance (ANOVA) when dealing with ordinal data.
Here's how it works:
- Ranking Data: Similar to the Mann-Whitney U Test, the Kruskal-Wallis Test ranks data from all groups together. It assigns ranks based on the combined dataset's values.
- Test Statistic: The Kruskal-Wallis test statistic H is calculated to assess whether there are significant differences among the groups. It considers the dispersion and distribution of ordinal data across multiple groups.
- Hypothesis Testing: The Kruskal-Wallis Test involves formulating null and alternative hypotheses. The test statistic H is compared to critical values from the Kruskal-Wallis distribution to determine if there are significant differences among the groups.
- Post-Hoc Tests: If the Kruskal-Wallis Test indicates significant differences among groups, post-hoc tests like Dunn's test or Conover's test can be conducted to identify which specific groups differ from each other.
- Assumptions: Like the Mann-Whitney U Test, the Kruskal-Wallis Test does not assume normality of data or equal variances. It is robust against skewed distributions and outliers.
These inferential statistical tests provide valuable insights into relationships and differences within ordinal data, allowing you to draw conclusions about the significance of your findings. Choosing the appropriate test depends on the nature of your research question and the number of groups you're comparing.
Regression Analysis with Ordinal Data
Regression analysis is a powerful statistical tool that allows you to explore relationships between variables. When working with ordinal data, it's essential to use specialized regression techniques tailored to the unique characteristics of this data type.
Ordinal Logistic Regression
Ordinal Logistic Regression is a regression model designed to analyze the relationship between ordinal dependent variables and one or more independent variables. It's a versatile tool when you want to predict the odds of an outcome falling into a specific ordinal category. Here's a detailed look at this regression method:
- Dependent Variable: In ordinal logistic regression, the dependent variable is ordinal, meaning it consists of categories with a natural order but unequal intervals. Examples include Likert scale ratings, educational attainment levels, or socioeconomic status.
- Cumulative Logit Model: Ordinal logistic regression employs the cumulative logit model, which estimates the cumulative odds of an observation falling into a specific category or a lower category on the ordinal scale. These cumulative odds are then used to make predictions.
- Independent Variables: You can include one or more independent variables in the model to assess their impact on the ordinal dependent variable. Independent variables can be categorical or continuous, making this regression method flexible for a variety of research questions.
- Proportional Odds Assumption: Ordinal logistic regression assumes the proportional odds assumption. This means that the odds of an observation falling into a specific category are proportional across all levels of the independent variables. Violation of this assumption may necessitate alternative regression models.
- Interpretation: Interpretation of ordinal logistic regression results involves examining odds ratios. These ratios provide insights into the likelihood of an outcome occurring in a particular category compared to the reference category based on changes in the independent variables.
- Model Fit and Validation: Assessing the goodness of fit of the model and validating its predictive performance are essential steps in ordinal logistic regression. Techniques like likelihood ratio tests and cross-validation can be employed for this purpose.
Proportional Odds Model
The Proportional Odds Model, also known as the parallel regression model, is a specific form of ordinal logistic regression. It assumes that the relationship between independent variables and the ordinal dependent variable is consistent across all levels of the ordinal categories. Here's a closer examination of this model:
- Cumulative Log-Odds: Like ordinal logistic regression, the Proportional Odds Model estimates cumulative log-odds of an observation falling into a specific category or a lower category on the ordinal scale.
- Simplified Assumption: The key assumption of this model is that the coefficients for independent variables remain constant across the ordinal categories. In other words, the odds ratios associated with the independent variables do not vary as you move up or down the ordinal scale.
- Advantages: The Proportional Odds Model simplifies interpretation by assuming a consistent relationship. This can be advantageous when this assumption holds true in your data.
- Likelihood Ratio Test: Researchers often use the likelihood ratio test to assess whether the proportional odds assumption is met. If the assumption is violated, alternative models like the partial proportional odds model or adjacent-category logit model may be considered.
- Practical Application: This model is widely applied in various fields, such as psychology, epidemiology, and social sciences, to analyze ordinal data when the proportional odds assumption is deemed reasonable.
Selecting the appropriate regression method for your ordinal data analysis depends on the nature of your research question, the assumptions of the models, and the characteristics of your data. Careful consideration and model validation are crucial in conducting meaningful regression analyses with ordinal data.
Ordinal Data Examples
To gain a deeper understanding of ordinal data and its real-world applications, let's explore some concrete examples across various domains. These examples illustrate how ordinal data is collected, represented, and utilized in different contexts.
Education Levels
One common application of ordinal data is the representation of educational attainment levels. In this scenario, individuals' highest education achieved is categorized into ordinal categories, typically ranging from lower to higher levels of education. Examples of such categories include:
- High School Diploma: This category represents individuals who have completed their high school education.
- Associate's Degree: This level indicates individuals who have earned an associate's degree typically obtained from a community college.
- Bachelor's Degree: Individuals who have completed a bachelor's degree at a college or university fall into this category.
- Master's Degree: Those who have pursued further education and obtained a master's degree occupy this ordinal category.
- Doctorate: The highest level of educational achievement is often represented by this category, including individuals with doctoral degrees such as Ph.D. or Ed.D.
Ordinal data on education levels is frequently used in studies related to employment, income, and socioeconomic status. Researchers can analyze this data to understand the impact of education on various outcomes, such as job opportunities, earnings potential, and career advancement.
Rating Scales in Customer Surveys
Ordinal data is extensively utilized in customer satisfaction surveys and product evaluations. Researchers often employ rating scales to gather customer opinions, preferences, or feedback. A prime example is the Likert scale, which offers respondents a range of options to express their level of agreement or satisfaction, such as:
- Strongly Disagree
- Disagree
- Neutral
- Agree
- Strongly Agree
Each response category in the Likert scale represents an ordinal level of agreement, and respondents select the option that best aligns with their sentiment. The data collected using such scales are then analyzed to assess customer satisfaction, identify areas for improvement, or make marketing decisions.
Pain Severity Assessment
In healthcare and medical research, ordinal data is frequently encountered when assessing the severity of pain or discomfort experienced by patients. Medical professionals often use ordinal pain scales to gauge the intensity of pain, with categories that may include:
- No Pain
- Mild Pain
- Moderate Pain
- Severe Pain
- Excruciating Pain
Patients are asked to describe their pain levels based on these ordinal categories, allowing healthcare providers to make informed decisions regarding pain management and treatment options.
Socioeconomic Status
Socioeconomic status (SES) is another area where ordinal data plays a crucial role. Researchers and sociologists often classify individuals or households into ordinal categories based on factors such as income, education, and occupation. Ordinal categories for SES may include:
- Low SES
- Lower-Middle SES
- Upper-Middle SES
- High SES
Analyzing ordinal SES data helps researchers understand disparities in access to resources, opportunities, and quality of life, contributing to studies on social inequality and policy development.
These examples illustrate how ordinal data is encountered in diverse fields and how it effectively captures information with inherent order or ranking. By recognizing and appropriately analyzing ordinal data, researchers can extract valuable insights and inform decision-making processes in numerous areas of study.
Assumptions of Ordinal Data Analysis
When working with ordinal data, several assumptions should guide your analysis. Here are the key assumptions:
- Proportional Odds Assumption: Many ordinal data analysis techniques, such as ordinal logistic regression and the proportional odds model, rely on the assumption that the relationship between independent variables and the ordinal dependent variable remains constant across all levels of the ordinal categories. Violating this assumption can lead to inaccurate results.
- Independence of Observations: It is assumed that the observations in your dataset are independent of each other. In other words, the response of one individual or unit should not be influenced by or related to the response of another individual.
- Ordinality of the Data: It's crucial to recognize that your data truly represents ordinal categories with a meaningful order but unequal intervals. Treating ordinal data as if it were interval or ratio data can lead to erroneous conclusions.
- Proper Scaling: When assigning numerical values to ordinal categories for analysis, ensure that the scaling is logical and meaningful. Avoid arbitrary numerical assignments that might misrepresent the data.
Ordinal Data Limitations
While ordinal data is valuable in various research contexts, it comes with inherent limitations that researchers must consider:
- Loss of Information: Ordinal data, by nature, does not capture the full range of nuances in underlying attitudes, preferences, or perceptions. It simplifies complex information into ordered categories, potentially leading to a loss of detailed insights.
- Equal Spacing Assumption: Analyzing ordinal data often involves treating the intervals between categories as equal, even though they may not be. This assumption can introduce errors in statistical analyses, mainly when working with models that assume equal intervals.
- Limited Arithmetic Operations: Arithmetic operations like addition and subtraction are not meaningful with ordinal data. You cannot accurately calculate the average of ordinal categories or perform other mathematical operations without violating the data's characteristics.
- Statistical Power: Ordinal data analysis may have lower statistical power compared to analyses of continuous data. This can make detecting statistically significant effects or relationships more challenging, especially with small sample sizes.
- Subjectivity in Coding: Assigning numerical values to ordinal categories can be subjective and may vary across researchers. This subjectivity can introduce inconsistencies or bias into the analysis.
- Interpretation Complexity: Interpreting results from ordinal data analyses can be more complex than working with continuous data. Researchers need to be cautious in explaining the practical significance of findings.
Understanding these assumptions and limitations is crucial for making informed decisions throughout the research process. While ordinal data analysis offers valuable insights, acknowledging its constraints helps researchers avoid pitfalls and draw robust conclusions.
Conclusion for Ordinal Data
Ordinal data is a valuable asset in the world of research and analysis. Its ordered categories help us understand preferences, make informed decisions, and uncover patterns in various fields, from education to healthcare. By recognizing the characteristics and nuances of ordinal data, you can confidently collect, analyze, and interpret this type of information to gain valuable insights.
Remember, ordinal data may have its limitations, but when handled with care and using appropriate statistical techniques, it can unlock a wealth of knowledge and contribute to meaningful discoveries. So, whether you're conducting surveys, evaluating customer feedback, or exploring socioeconomic trends, ordinal data is a reliable companion on your journey to better understand the world around you.
How to Collect Ordinal Data in Minutes?
Introducing Appinio, the real-time market research platform that revolutionizes ordinal data collection. Say goodbye to the complexities of traditional research and embrace the future of fast, intuitive, and seamlessly integrated consumer insights.
Here's why:
- Rapid Insights: Get from questions to valuable insights in minutes, empowering you to make data-driven decisions swiftly.
- User-Friendly: No need for a PhD in research; our platform is designed for everyone, making market research accessible and enjoyable.
- Global Reach: Survey your target audience in over 90 countries, with precise targeting based on 1200+ characteristics.
Get facts and figures 🧠
Want to see more data insights? Our free reports are just the right thing for you!